The use of artificial intelligence with uncertainty estimation to predict lung cancer relapse from histopathology.
Journal of Clinical Oncology(2022)
摘要
8549 Background: First-line treatment of early stage non-small cell lung cancer (NSCLC) is surgical resection, but with a 5-year survival of only 54%, rates of future relapse are high. Identifying patients at high risk of relapse can help guide adjuvant treatment decisions. Deep convolutional neural network (DCNN) AI models trained on tumor histology have shown incredible flexibility as potential biomarkers, both in lung cancer and more generally across many malignancies. While DCNN models can obtain extremely accurate results when used for routine purposes such as diagnosis, subtyping, and grading of malignancies, most models trained for prognostication or treatment response prediction do not reach performance sufficient for clinical application. Uncertainty quantification (UQ) – a family of techniques that give DCNN models the ability to report confidence alongside predictions – is an underexplored avenue in cancer AI that may help further improve performance and clinical application of models designed to provide clinicians with estimations of risk. Methods: To explore the potential use of UQ in clinically-oriented DCNN models, we trained models on a single-institution, retrospective digital tumor histology slide cohort from patients with Stage I-III NSCLC who underwent surgical resection to predict risk of future relapse. Estimation of uncertainty was performed using dropout as a Bayesian approximation, and uncertainty thresholds were calculated from training sets using a novel method to identify and remove low-confidence predictions. For comparison, a separate multivariate logistic regression was trained in cross-validation using known clinical risk factors. Results: We trained DCNN models on slides from 198 patients (40 relapsed, 158 without relapse). In this cohort, 130 patients had stage I disease (65.6%), 42 had stage II (21.2%), and 26 had stage III (13.2%). The average age was 69 years, 85% were current or previous smokers, and 38 received guideline-concordant adjuvant chemotherapy. Without UQ, a DCNN model predicted risk of future relapse with an average area under receiver operator curve (AUROC) of 0.74 across three-fold cross-validation. Using UQ estimation, 63% of slides were reported with high confidence. Relapse prediction was significantly improved in the high-confidence cohort, with an average AUROC of 0.83 in cross-validation. With a specificity of 70%, this corresponds to an average sensitivity of 86.8% across the three cross-folds (79.1%, 85.5%, and 95.7%). In comparison, a clinical-only multivariate regression model predicted relapse with a cross-validated AUROC of 0.67. Conclusions: This method of uncertainty quantification appears to be a powerful tool to predict lung cancer recurrence risk from digital histopathology while simultaneously providing clinicians with a measurement of algorithm trustworthiness.
更多查看译文
关键词
lung cancer relapse,lung cancer,uncertainty estimation
AI 理解论文
溯源树
样例
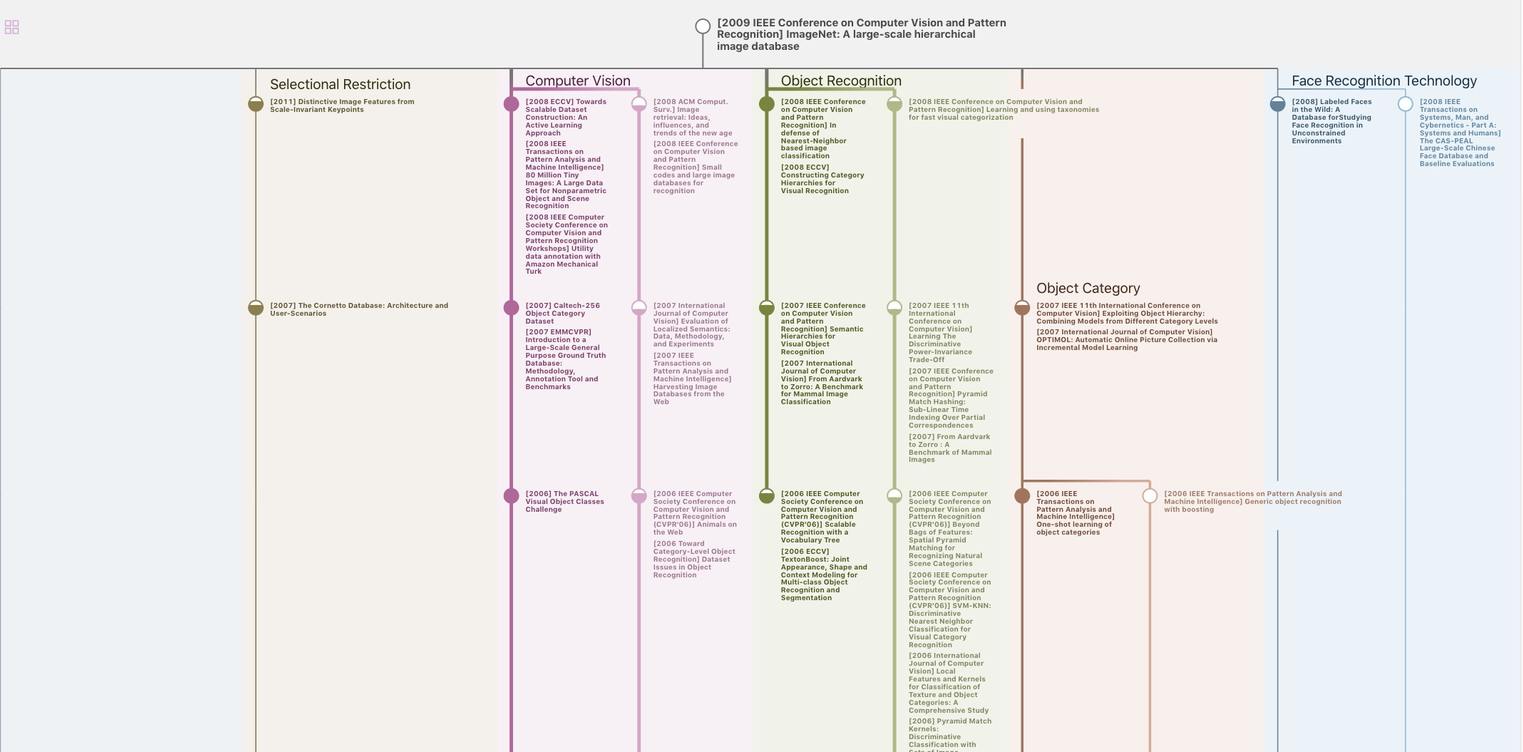
生成溯源树,研究论文发展脉络
Chat Paper
正在生成论文摘要