Fully Automated 3D Vestibular Schwannoma Segmentation with and without Gadolinium-based Contrast Material: A Multicenter, Multivendor Study
RADIOLOGY-ARTIFICIAL INTELLIGENCE(2022)
摘要
Purpose: To develop automated vestibular schwannoma measurements on contrast-enhanced T1- and T2-weighted MRI scans. Materials and Methods: MRI data from 214 patients in 37 different centers were retrospectively analyzed between 2020 and 2021. Patients with hearing loss (134 positive for vestibular schwannoma [mean age 6 SD, 54 years 6 12; 64 men] and 80 negative for vestibular schwannoma) were randomly assigned to a training and validation set and to an independent test set. A convolutional neural network (CNN) was trained using fivefold cross-validation for two models (T1 and T2). Quantitative analysis, including Dice index, Hausdorff distance, surface-to-surface distance (S2S), and relative volume error, was used to compare the computer and the human delineations. An observer study was performed in which two experienced physicians evaluated both delineations. Results: The T1-weighted model showed state-of-the-art performance, with a mean S2S distance of less than 0.6 mm for the whole tumor and the intrameatal and extrameatal tumor parts. The whole tumor Dice index and Hausdorff distance were 0.92 and 2.1 mm in the independent test set, respectively. T2-weighted images had a mean S2S distance less than 0.6 mm for the whole tumor and the intrameatal and extrameatal tumor parts. The whole tumor Dice index and Hausdorff distance were 0.87 and 1.5 mm in the independent test set. The observer study indicated that the tool was similar to human delineations in 85%-92% of cases. Conclusion: The CNN model detected and delineated vestibular schwannomas accurately on contrast-enhanced T1- and T2-weighted MRI scans and distinguished the clinically relevant difference between intrameatal and extrameatal tumor parts. (C) RSNA, 2022
更多查看译文
关键词
MRI,Ear,Nose, and Throat,Skull Base,Segmentation,Convolutional Neural Network (CNN),Deep Learning Algorithms,Machine Learning Algorithms
AI 理解论文
溯源树
样例
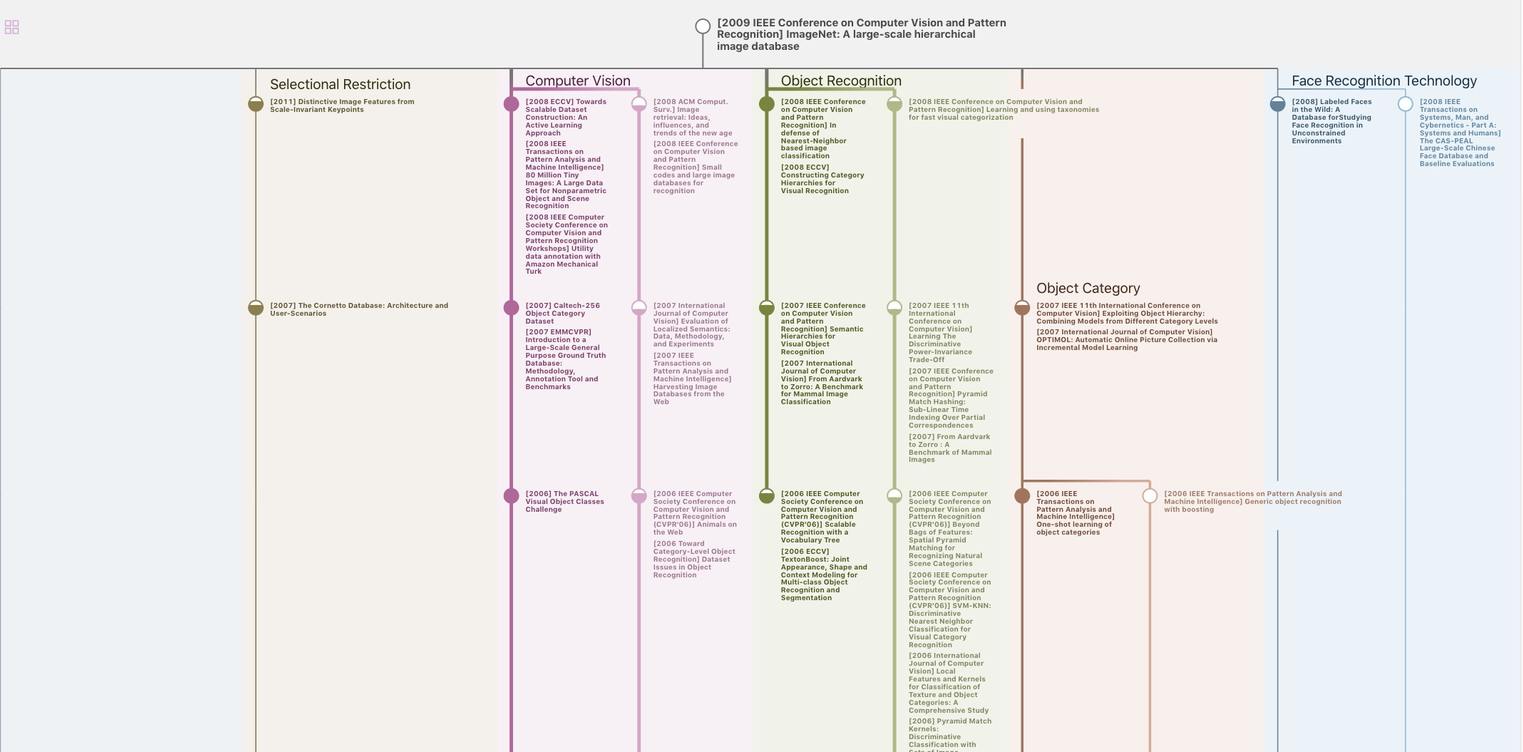
生成溯源树,研究论文发展脉络
Chat Paper
正在生成论文摘要