Learning to Overcome Noise in Weak Caption Supervision for Object Detection
IEEE Transactions on Pattern Analysis and Machine Intelligence(2023)
摘要
We propose the first mechanism to train object detection models from weak supervision in the form of captions at the image level. Language-based supervision for detection is appealing and inexpensive: many blogs with images and descriptive text written by human users exist. However, there is significant noise in this supervision: captions do not mention all objects that are shown, and may mention extraneous concepts. We first propose a technique to determine which image-caption pairs provide suitable signal for supervision. We further propose several complementary mechanisms to extract image-level pseudo labels for training from the caption. Finally, we train an iterative weakly-supervised object detection model from these image-level pseudo labels. We use captions from four datasets (COCO, Flickr30K, MIRFlickr1M, and Conceptual Captions) whose level of noise varies. We evaluate our approach on two object detection datasets. Weighting the labels extracted from different captions provides a boost over treating all captions equally. Further, our primary proposed technique for inferring pseudo labels for training at the image level, outperforms alternative techniques under a wide variety of settings. Both techniques generalize to datasets beyond the one they were trained on.
更多查看译文
关键词
Language-supervised object detection,weakly-supervised object detection,vision and language
AI 理解论文
溯源树
样例
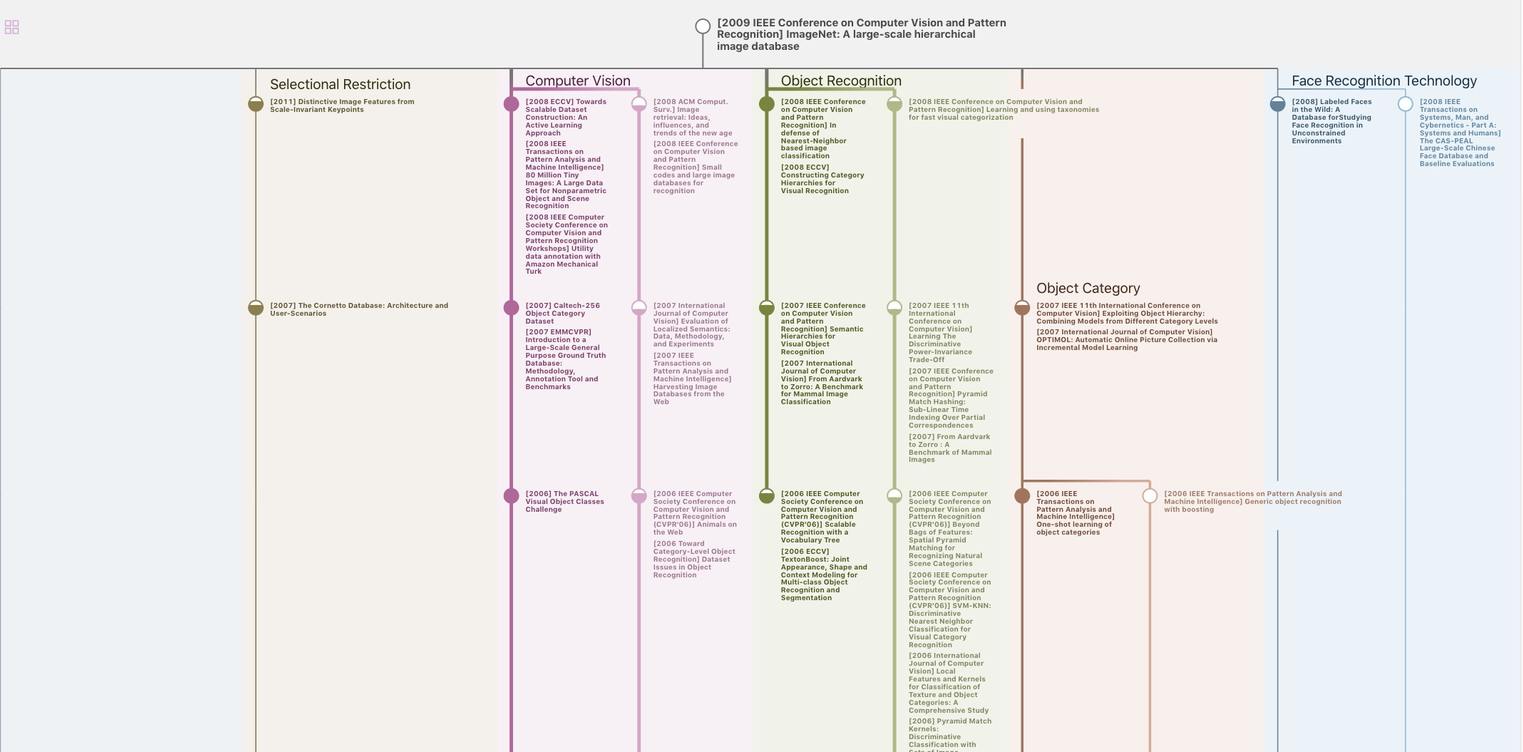
生成溯源树,研究论文发展脉络
Chat Paper
正在生成论文摘要