Using tandem repeat genomic features for cancer signal detection across multiple cancer types.
Journal of Clinical Oncology(2022)
摘要
e13586 Background: Next generation sequencing methods enable the identification of molecular signatures predictive of cancers. Large-scale cancer genomic projects such as The Cancer Genome Atlas (TCGA) molecularly characterized over 20,000 primary cancer and matched normal samples spanning 33 cancer types. The resulting data provides an opportunity to uncover a list of recurrent genomic aberrations, such as mutations, amplifications, insertions, and deletions, that can be used in machine learning models for multiple cancer type detection. Previous research has demonstrated significant variability in the stability of tandem repeat sequences between different cancer types, with consequences for gene expression, which is consistent with known oncogenic mechanisms. Methods: Our approach identifies a set of tandem repeats from whole-exome sequencing data to analyze, and computes differences relative to the reference genome in the cancer patients’ samples. For each tandem repeat sequence (referred to hereafter as TRS), we count the frequency of occurrence of the TRS in its reference state, as well as its specific variations (deletions/insertions) in each cancer patient exome sequence. These TRS read counts are the features for our cancer type prediction model. Through filtering out features of lower significance, we condense our training and testing datasets down from a half million possible features per sample, to merely thousands of features common across all the samples. From there, we train multi-class one-vs-all logistic regression models rapidly and optimize our feature selection and preprocessing to maximize predictive accuracy. Results: As can be seen in the Table below, our logistic regression model trained on TRS features enables us to predict cancer type with high accuracy in TCGA patient samples (as compared with random prediction accuracy, which would be approximately 10%). Conclusions: This approach lays the groundwork for novel TRS-based genetic tests for early detection and diagnosis of multiple types of cancer. [Table: see text] [Table: see text]
更多查看译文
关键词
Cancer Progression
AI 理解论文
溯源树
样例
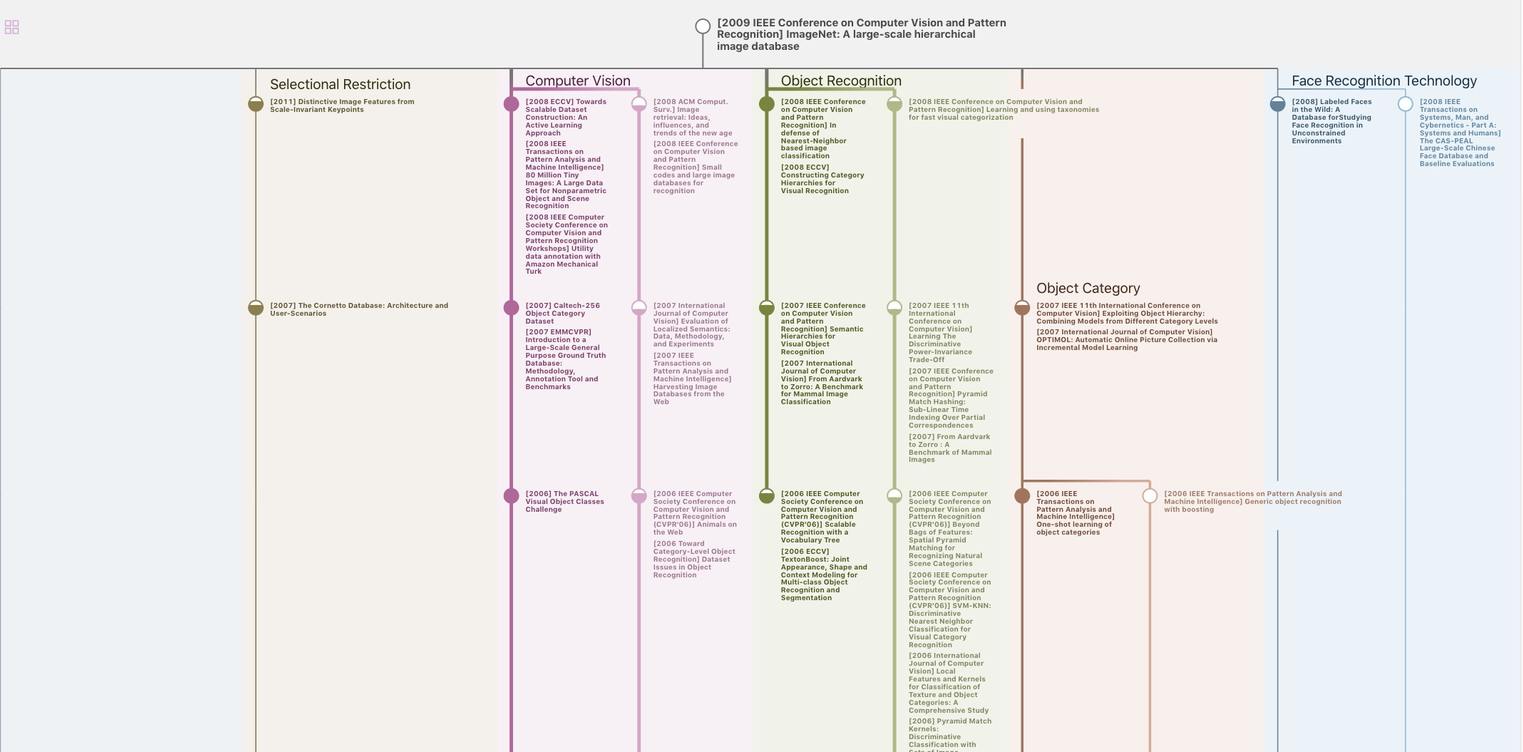
生成溯源树,研究论文发展脉络
Chat Paper
正在生成论文摘要