MUAN: Multi-Scale Up-Sampling Aggregation Network for 3D Point Cloud Segmentation.
IEEE Geoscience and Remote Sensing Letters(2022)
摘要
Three-dimensional point cloud semantic segmentation is of great significance for self-driving and virtual reality (VR), and it is an important research topic in 3-D vision. In this letter, we present a multiscale upsampling aggregation network (MUAN) to improve semantic segmentation performance in 3-D sophisticated environments. We take position adaptive convolution (PAConv) as the backbone of MUAN. First, to overcome the problem of weak capture ability of the multilayer perceptron (MLP) in low-dimensional space, we introduce an Attentive ScoreNet (ASN) module that combines a point feature enrichment (PFE) module and an attention mechanism to ensure provide effective distribution scores for the weight matrices. Second, to address the issue of the semantic gap between the encoders and decoders, we present a novel multiscale upsampling aggregation (MUA) module that expands the receptive field before semantic prediction, and it fused multiscale features containing information about multiscale encoder blocks and decoder blocks. Third, to facilitate the MUA module to achieve better results, we design a bilateral feature fusion (BFF) module which aims to boost the global awareness of the network in each decoder block. A combination of BFF and MUA pays attention to multiscale features of the encoder and decoder, thereby improving both the semantic representation and the possibility of correct semantic prediction of the point cloud. The experimental results reported that our MUAN can improve at least 2.36% mean intersection over union (mIoU) and 0.5% class mIoU in the Stanford 3D indoor scene dataset (S3DIS) Area-5 and ShapeNet datasets compared with PAConv.
更多查看译文
关键词
Semantics, Point cloud compression, Three-dimensional displays, Feature extraction, Decoding, Kernel, Fuses, 3-D point cloud segmentation, Attentive ScoreNet (ASN), bilateral feature fusion (BFF), multiscale upsampling aggregation (MUA), semantic gap
AI 理解论文
溯源树
样例
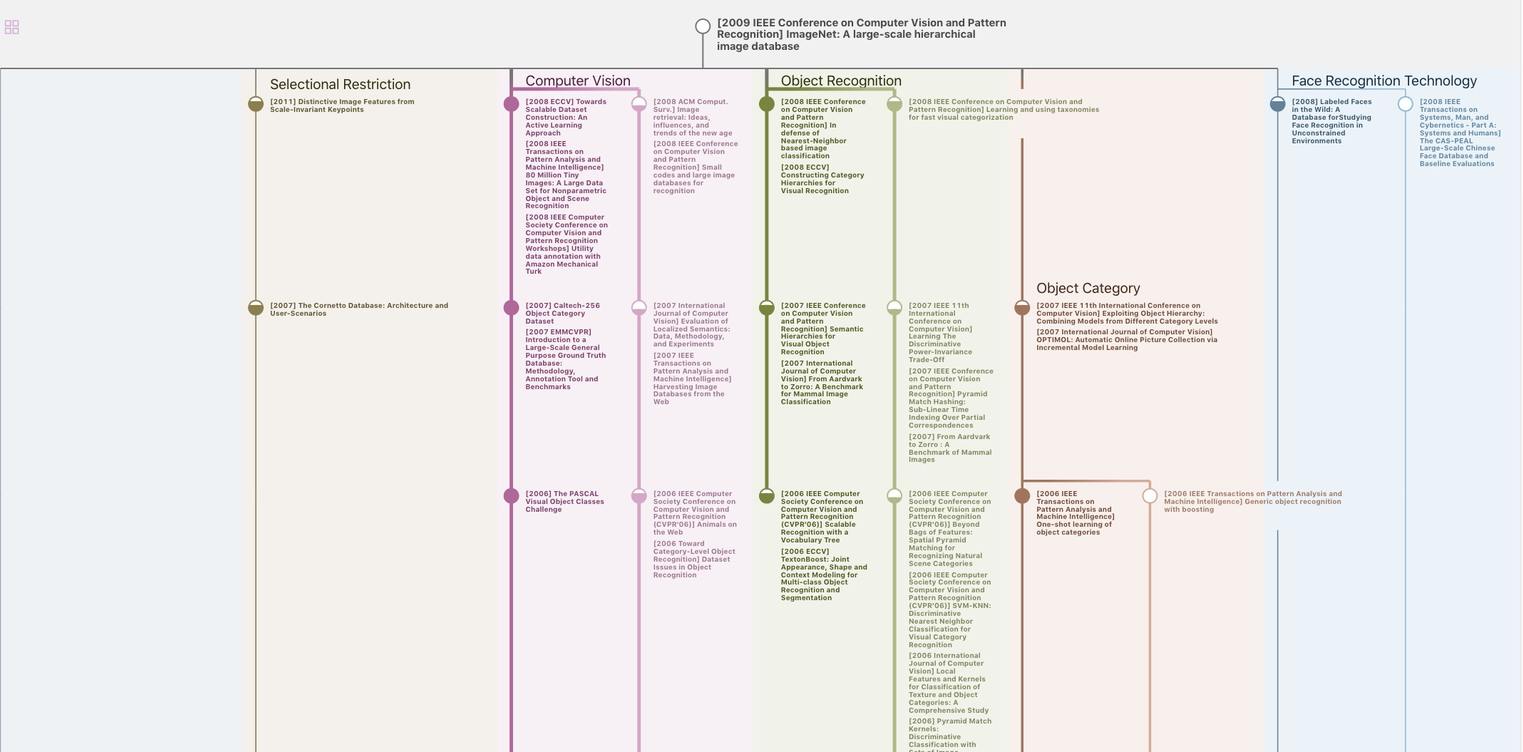
生成溯源树,研究论文发展脉络
Chat Paper
正在生成论文摘要