Recurring event survival analyses: A methodological approach to model recurring event data in cancer patients receiving multiple lines of therapy.
Journal of Clinical Oncology(2022)
摘要
e18693 Background: In cancer treatment, patients often receive multiple rounds of treatment, or Lines of Therapy (LoT). The time between start of a LOT and change to a new LOT is an important outcome in clinical oncology, for understanding disease progression and anticipating response to further treatment. Research questions may focus on the associations between duration of an LOT (the dependent variable) and independent variables of interest, such as time-invariant demographic (gender, age, race) and clinical factors (comorbidities, stage of disease) as well as time-varying factors such as Patient Reported Outcomes (PRO’s), resource utilization, and adverse events. These associations can be tested empirically with traditional “survival analyses”, more generally known as time-to-event when there is a single event (such as 5-year survival). The analyses, however, may become more complex when patients experience more than one LOT. This is due to the possibility that the Time-to-Events may be correlated within an individual patient, similarly to how outcome variables are correlated in other repeated measures designs. If this correlation is not captured in the modeling, the validity of parameters estimates may be in doubt. Thus, it is important to analyze the data using methods which account for the within-person correlation among LOT durations through the use of “Recurring Event” survival analyses. Methods: This methodological presentation will report an example of a real world “Recurring Event” analysis in a study of Multiple Myeloma patients who received one or more than one LOT. The study data set was derived from users of PROmpt®, an application for remote patient reporting and provider symptom management. Patient characteristics and treatment history were assessed by survey and review of patient EMR records. 80 patients were identified, with a treatment history of receiving between 1 and 19 LOT’s (mean = 5.2). Time invariant predictors are age, gender, and frailty (employing the Modified Geriatric Assessment). Time varying variables are the incidence of hematological and non-hematological toxicities. Results: The presentation will provide: an overview of how to interpret the model parameters, a discussion of the data structure required by the modeling, and a series of results in which LOT durations are analyzed as first independent and then when modeled as a “Recurring Event” analysis. The results are examined across different modeling strategies to illustrate a model testing approach, as well as to quantitatively demonstrate the impact on survival analysis results when incorporating “recurring event” methodology. Conclusions: The intent of the presentation is to alert researchers to the need to analyze Time-To-Event models using a recurring event framework when patients experience recurring events in order to produce valid and generalizable parameters.
更多查看译文
关键词
event survival,cancer patients,event data
AI 理解论文
溯源树
样例
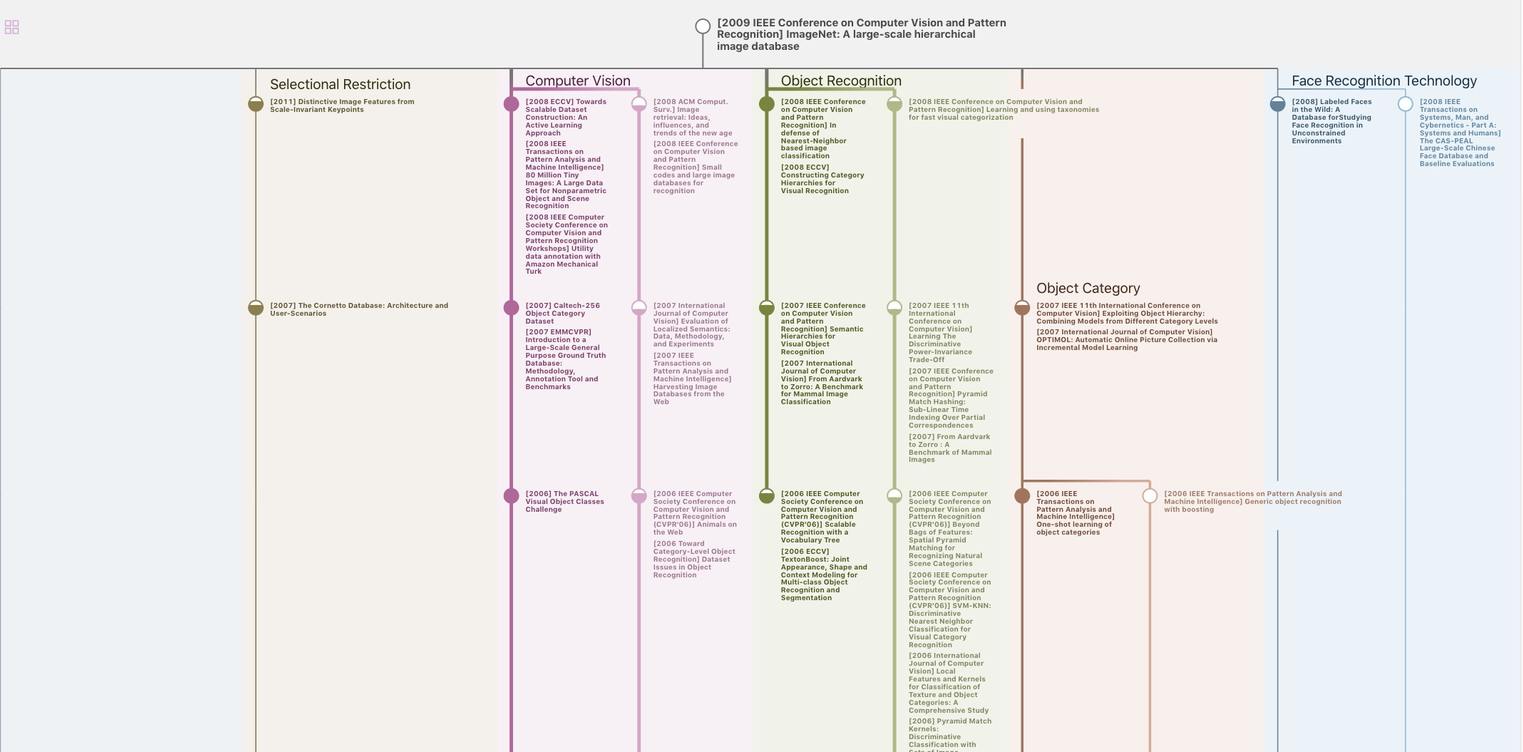
生成溯源树,研究论文发展脉络
Chat Paper
正在生成论文摘要