A Gaussian Mixture Model Based Fast Motion Planning Method Through Online Environmental Feature Learning
IEEE Transactions on Industrial Electronics(2023)
摘要
Rapidly exploring random tree and its applications for mobile robot navigation have attracted extensive interest in the human-robot interaction environment. However, most current sampling-based path planning algorithms are easy to fall into the trap space in complex and dynamic environments. In this article, a fast path planning algorithm based on Gaussian mixture model (GMM) is proposed to allow robotic systems to efficiently extract feature nodes and generate collision-free paths considering the pedestrian density and the environmental structure. First, the preliminary feature nodes are obtained by training GMM, in which the covariance matrix of each feature node contains the environmental structure information. Second, an online feature learning method is presented to extract features during the movement of the robot by the dual-channel scale filter and the secondary distance fusion. Once the obstacle-free grid points can be connected to the feature nodes without any collision, a heuristic path is generated directly to guide the robot to avoid the crowds according to the proposed pedestrian matrix. For the same environment, the learned information can be reused as prior knowledge in the next path planning to further improve the efficiency of path replanning. A series of experimental studies demonstrate that our proposed method can remarkably reduce the time taken to reach a goal and enhance the success rate of navigation in trapped and narrow environments.
更多查看译文
关键词
Gaussian mixture model (GMM),mobile robots,online feature learning,sampling-based path planning
AI 理解论文
溯源树
样例
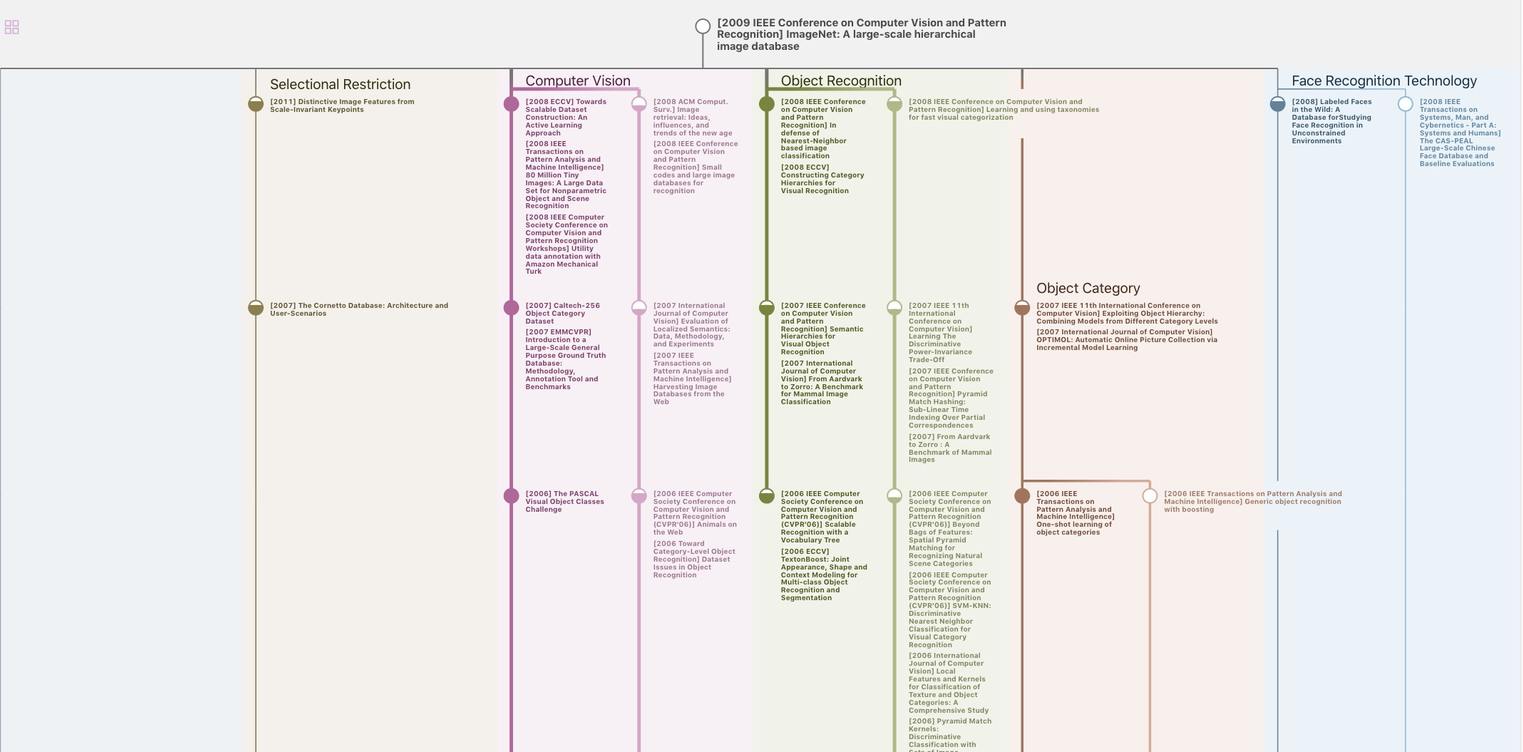
生成溯源树,研究论文发展脉络
Chat Paper
正在生成论文摘要