High fidelity virtual try-on network via semantic adaptation and distributed componentization
Computational Visual Media(2022)
摘要
Image-based virtual try-on systems have significant commercial value in online garment shopping. However, prior methods fail to appropriately handle details, so are defective in maintaining the original appearance of organizational items including arms, the neck, and in-shop garments. We propose a novel high fidelity virtual try-on network to generate realistic results. Specifically, a distributed pipeline is used for simultaneous generation of organizational items. First, the in-shop garment is warped using thin plate splines (TPS) to give a coarse shape reference, and then a corresponding target semantic map is generated, which can adaptively respond to the distribution of different items triggered by different garments. Second, organizational items are componentized separately using our novel semantic map-based image adjustment network (SMIAN) to avoid interference between body parts. Finally, all components are integrated to generate the overall result by SMIAN. A priori dual-modal information is incorporated in the tail layers of SMIAN to improve the convergence rate of the network. Experiments demonstrate that the proposed method can retain better details of condition information than current methods. Our method achieves convincing quantitative and qualitative results on existing benchmark datasets.
更多查看译文
关键词
virtual try-on,conditional image synthesis,human parsing,thin plate spline,semantic adaptation
AI 理解论文
溯源树
样例
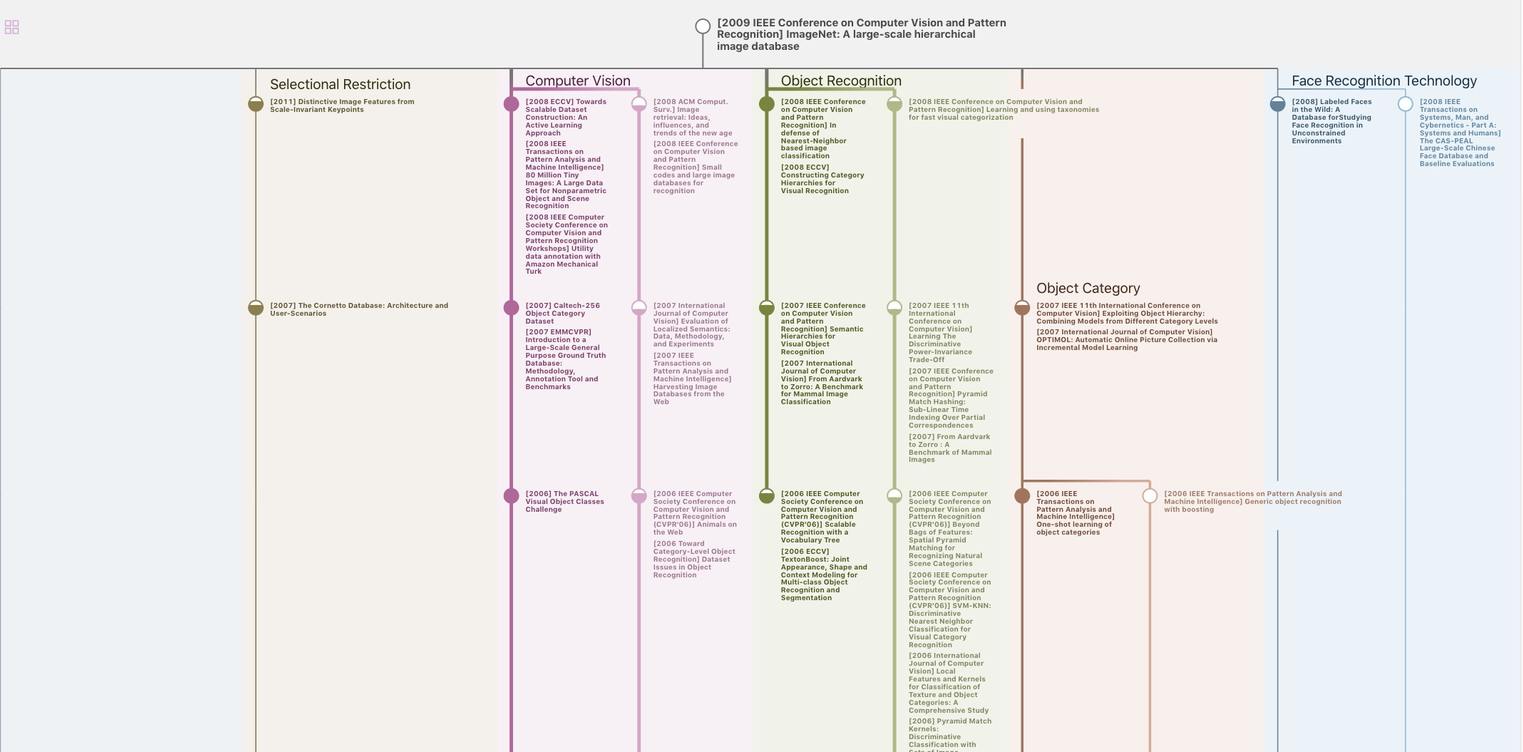
生成溯源树,研究论文发展脉络
Chat Paper
正在生成论文摘要