Comparing Methods for Handling Missing Covariates in Meta‐Regression
Research Synthesis Methods(2022)
摘要
Meta-analysts often encounter missing covariate values when estimating meta-regression models. In practice, ad hoc approaches involving data deletion have been widely used. The current study investigates the performance of different methods for handling missing covariates in meta-regression, including complete-case analysis (CCA), shifting-case analysis (SCA), multiple imputation (MI), and full information maximum likelihood (FIML), assuming missing at random mechanism. According to the simulation results, we advocate the use of MI and FIML than CCA and SCA approaches in practice. In addition, we cautiously note the challenges and potential advantages of using MI in the meta-analysis context.
更多查看译文
关键词
complete-case analysis,full information maximum likelihood,meta-analysis,meta-regression,missing data,multiple imputation,shifting-case analysis
AI 理解论文
溯源树
样例
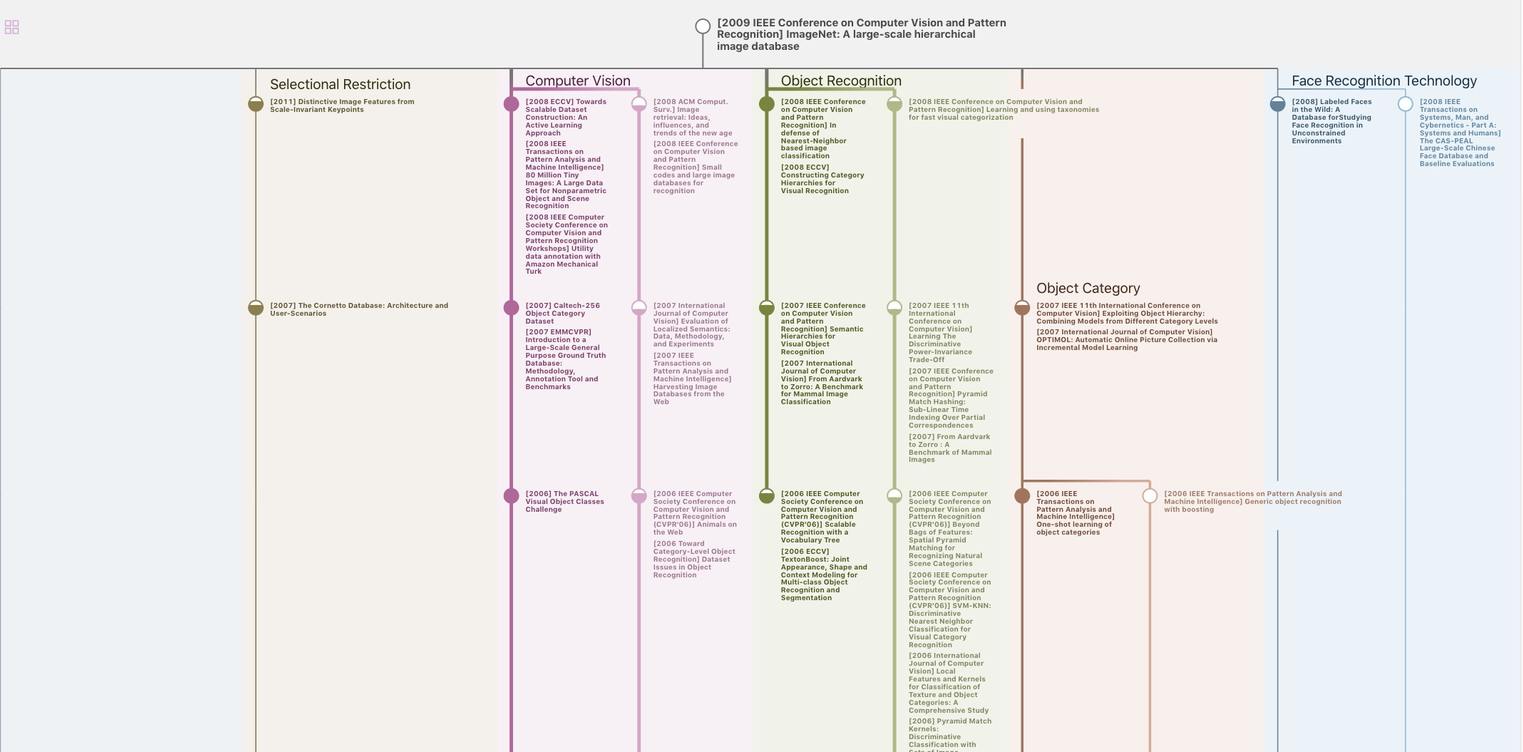
生成溯源树,研究论文发展脉络
Chat Paper
正在生成论文摘要