TaskPOI Priority-Based Energy Balanced Multi-UAVs Cooperative Trajectory Planning Algorithm in 6G Networks
IEEE Transactions on Green Communications and Networking(2023)
摘要
In the emergency rescue scenario where the communication infrastructure is damaged, Unmanned Air Vehicle (UAV) networking communication, as a part of the 6G air-space-ground integrated network system, plays a huge role in the establishment of the emergency communication network. In this paper, we fully consider the distribution of rescue areas, the type of tasks and the characteristics of UAVs, and design the TaskPOI-based multi-UAVs collaborative trajectory planning algorithm (TMCTP) based on regional tasks, aiming to improve the completed efficiency of rescue tasks and the utilization rate of energy consumption in the UAV backbone network. Firstly, we filter the population-intensive points of interest (PiPOI) region based on the location heat map of population density distribution and use the K-means clustering algorithm to generate task points of interest (TaskPOI) and use the neural collaborative filtering algorithm to determine the task priority and different priorities correspond to different task types. Then, we design a single UAV flight model and a multi-UAVs cooperative flight model. At the same time, considering the flight energy consumption, communication energy consumption and data acquisition energy consumption of UAV, the energy consumption equilibrium distribution model is designed to ensure that multi-UAVs have low energy consumption and balance the residual energy distribution while completing various levels of tasks. Finally, based on UAV flight model, energy consumption equilibrium distribution model, and combined with task priority of TaskPOI, TMCTP algorithm for the trajectory planning of the UAV group is designed by using multi-agent reinforcement learning algorithm. The experimental results show that in the TMCTP algorithm, the success rate of the UAV arriving at the goal and the completed rate of the action are converged to 1, the times of collisions is converged to 0, and the average reward, the loss of algorithm are all converged to a good degree, and the PiPOI coverage rate in the area exceeds 60%. In terms of energy consumption, the difference between the energy consumption of each UAV is small, and compared with the average residual energy using GP3, the difference is not large, indicating that the algorithm can improve the PiPOI coverage of the entire rescue area without generating a large energy consumption. In this way, the UAV group after arriving at the goal will provide communication services for the rescue area and improve the connectivity and life cycle of the 6G air network.
更多查看译文
关键词
Multi-UAVs cooperation,trajectory planning algorithm,multi-agent reinforcement learning,balanced distribution of energy consumption,6G
AI 理解论文
溯源树
样例
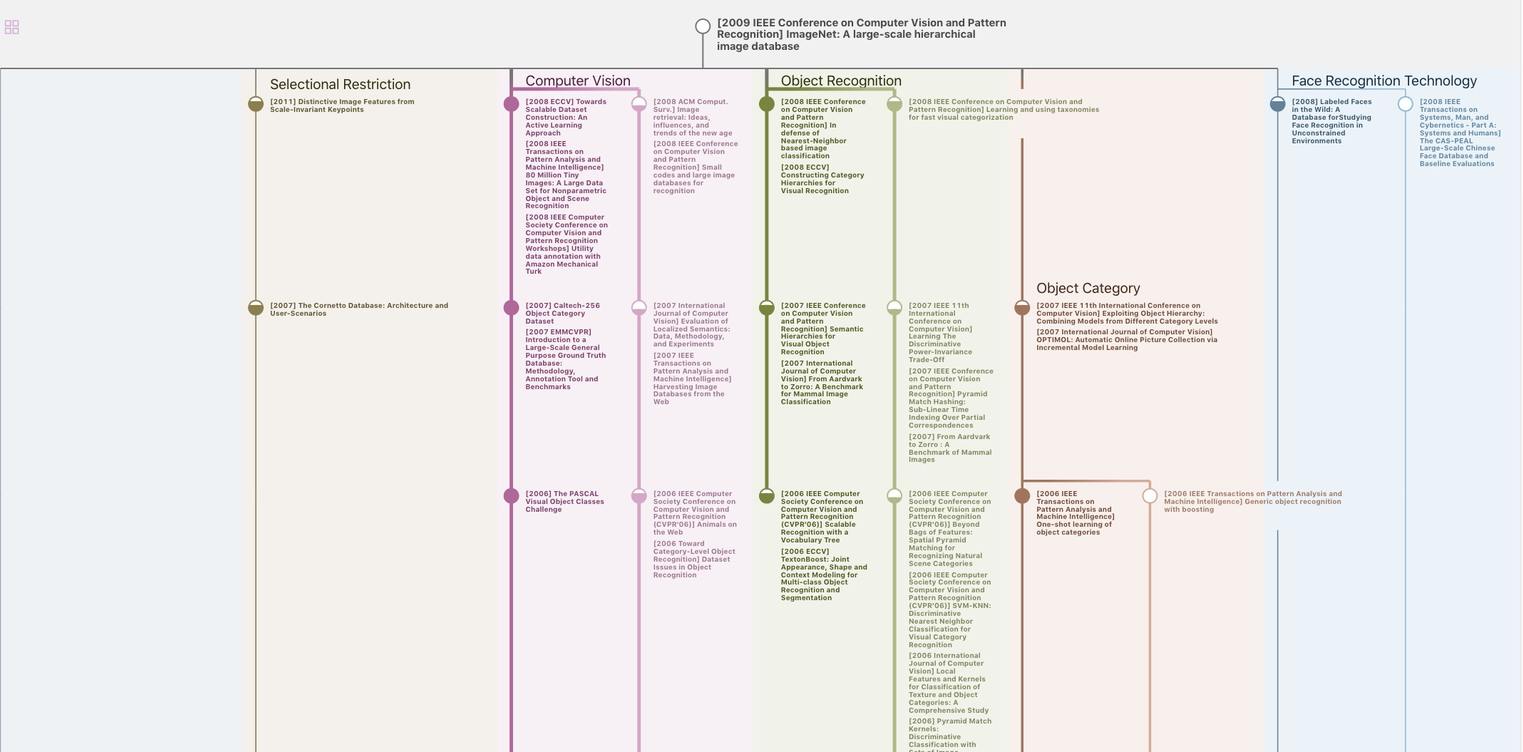
生成溯源树,研究论文发展脉络
Chat Paper
正在生成论文摘要