Use of latent profile analysis to characterise patterns of participation in crowdsourcing.
BEHAVIOUR & INFORMATION TECHNOLOGY(2023)
摘要
Crowdsourced applications are plagued by participation inequality. Only a small number of individuals are responsible for the majority of contributions, while most are engaged at the periphery. Early efforts to understand participation inequality used a percentile approach and a single measure of engagement to describe participants, usually the number of activities completed. With the advent of increasingly sophisticated web analytics, methods for characterising patterns of participation have also become more sophisticated. More recent research uses multiple metrics and clustering algorithms to understand both intensity and duration of participation. We extend research on this topic by applying a model-based approach to analyse multiple metrics of participation. Specifically, we demonstrate the use of latent profile analysis to understand the underlying structure of crowdsourcer participation data from a citizen science project. We make comparisons between latent profile analysis and more traditional methods of characterising participation. We find that considering multiple metrics of engagement provides a more nuanced view of participation inequality, useful in generating both theoretical and applied research questions. We also find that a model-based approach offers several advantages over clustering algorithms including the ease with which results can be integrated in downstream analyses and the ability to report on model fit.
更多查看译文
关键词
Participation inequality, clustering, citizen science, participatory research, crowdsourcing, segmentation
AI 理解论文
溯源树
样例
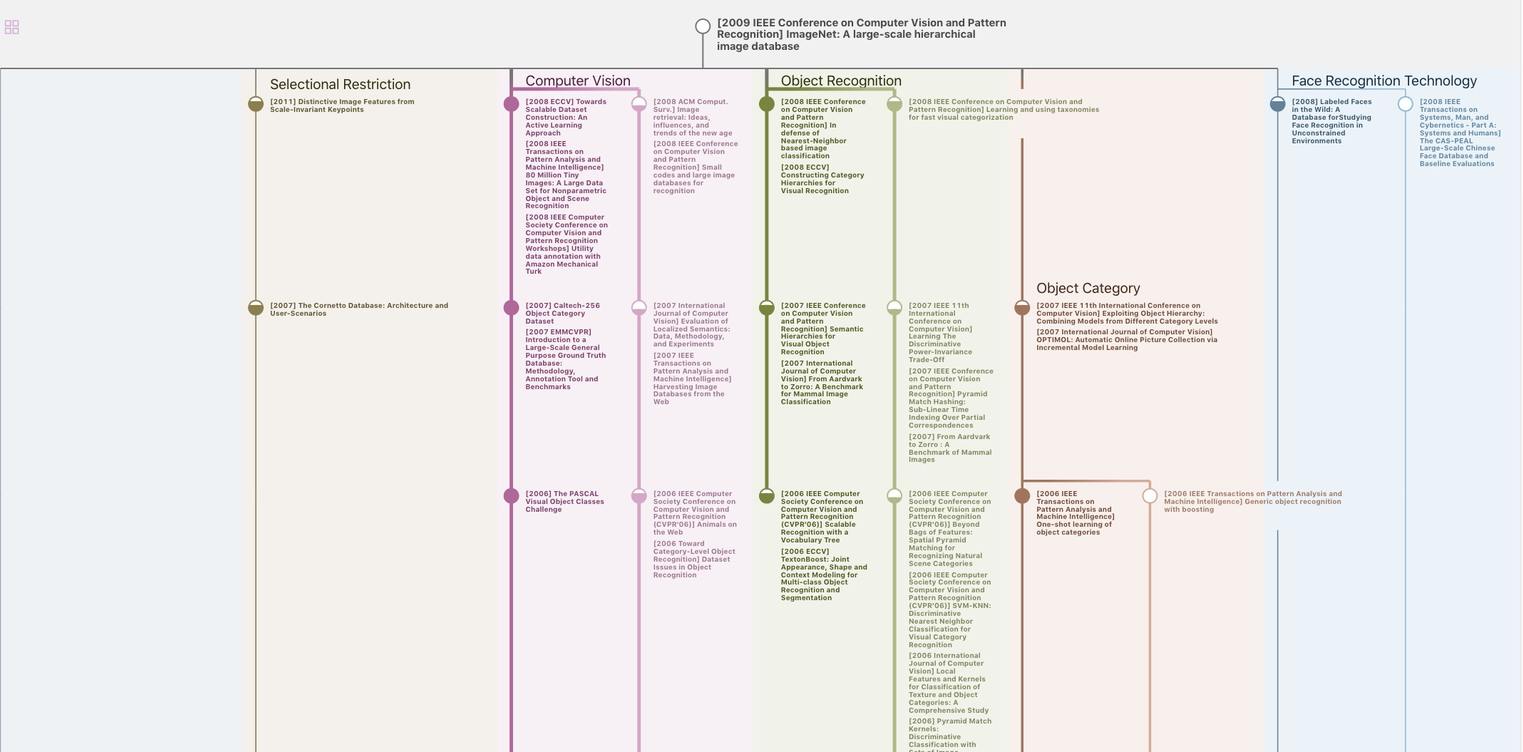
生成溯源树,研究论文发展脉络
Chat Paper
正在生成论文摘要