Task Offloading for Deep Learning Empowered Automatic Speech Analysis in Mobile Edge-Cloud Computing Networks
IEEE Transactions on Cloud Computing(2023)
摘要
With the explosive growth of mobile multimedia services and artificial intelligence applications involving automatic speech analysis (ASA), mobile devices are increasingly unable to handle these computation-intensive tasks generated by users due to the limited computing resource. Besides, the existing cloud computing paradigm is not capable of processing such real-time and delaysensitive ASA tasks. In this paper, by leveraging mobile edge computing and deep learning (DL), we investigate task offloading for DLempowered ASA in mobile edge-cloud computing networks to minimize the total time for processing ASA tasks, thereby providing an agile service response. Specifically, to accelerate the processing of ASA tasks, we decompose a convolutional neural network based encoder-decoder model and deploy the encoder at edge servers to extract the features of ASA tasks. Moreover, edge servers derive the user tolerance limit by using a linear regression model for further enhancing the quality of experience of users. Based on some certain network constraints (i.e., user association and edge servers' storage/computing capacity), we propose a low-complexity and distributed offloading framework to solve the formulated complex problem. Evaluation results demonstrate the effectiveness of the proposed framework on reducing the total time and improving the satisfaction rate of users.
更多查看译文
关键词
Automatic speech analysis,deep learning,edge-cloud computing,mobile edge computing,task offloading
AI 理解论文
溯源树
样例
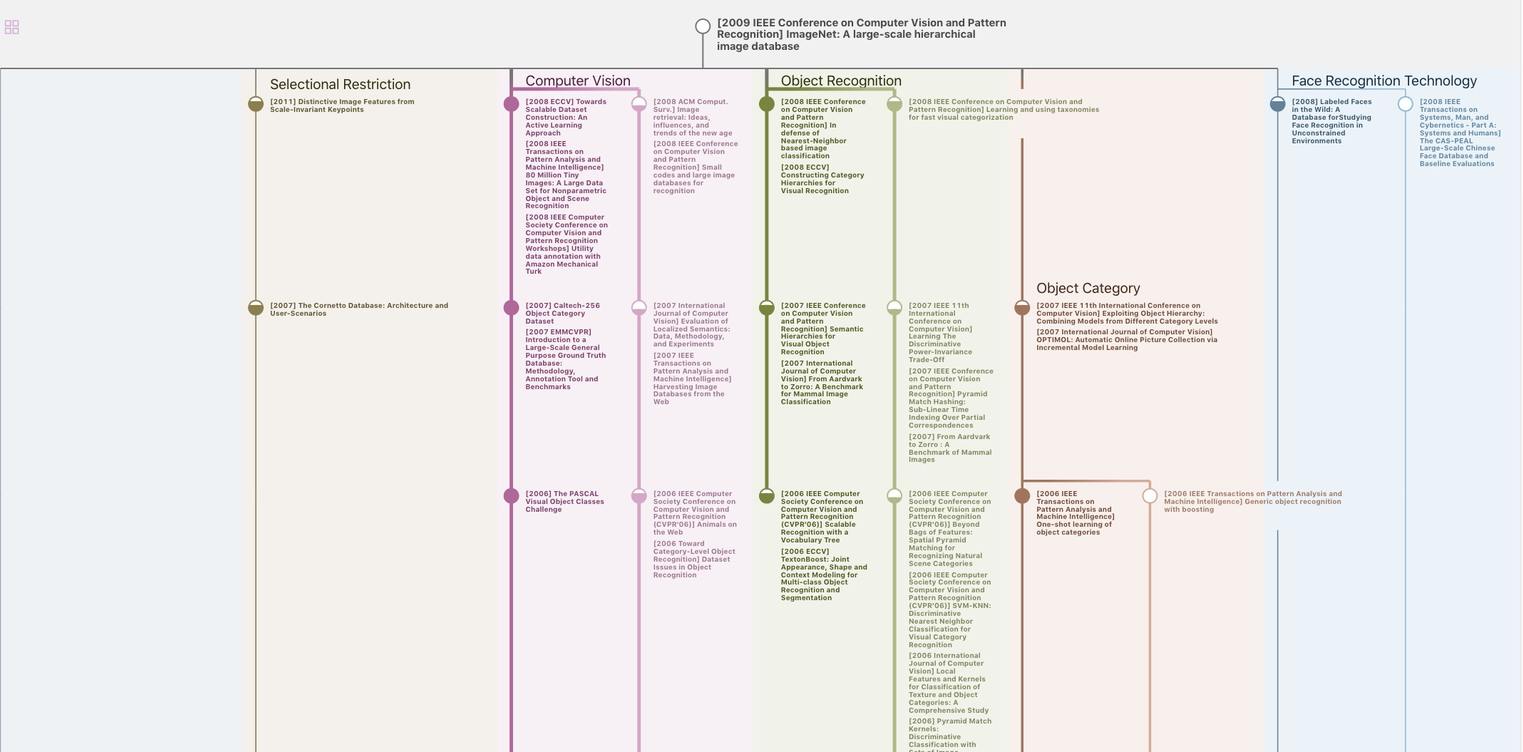
生成溯源树,研究论文发展脉络
Chat Paper
正在生成论文摘要