Detection of Direct Sequence Spread Spectrum Signals Based on Deep Learning
IEEE Transactions on Cognitive Communications and Networking(2022)
摘要
Direct spread spectrum communication has the advantages of strong anti-jamming ability and low probability of interception, which plays an essential role in both civil and military communications. The detection of direct sequence spread spectrum (DSSS) signal becomes very difficult because of its low power spectral density. In this paper, the detection of DSSS signals under non-cooperative conditions is carried out based on the classification technology of deep learning. Firstly, a detection scheme based on convolutional neural network (CNN) is proposed, in which the neural network is used to learn the features of DSSS signal and noise automatically without extracting the features in advance. In addition, we also propose a hybrid CNN-correlation (CORR)-based detection scheme in order to reduce the computational complexity. In this scheme, the autocorrelation of the received signal is truncated and used as the input of the neural network for training and inference. A large number of simulation results show that the detection performance of the proposed two schemes is significantly better than the traditional autocorrelation-based detection method in various scenarios.
更多查看译文
关键词
Detection,direct spread spectrum,deep learning,CNN,autocorrelation
AI 理解论文
溯源树
样例
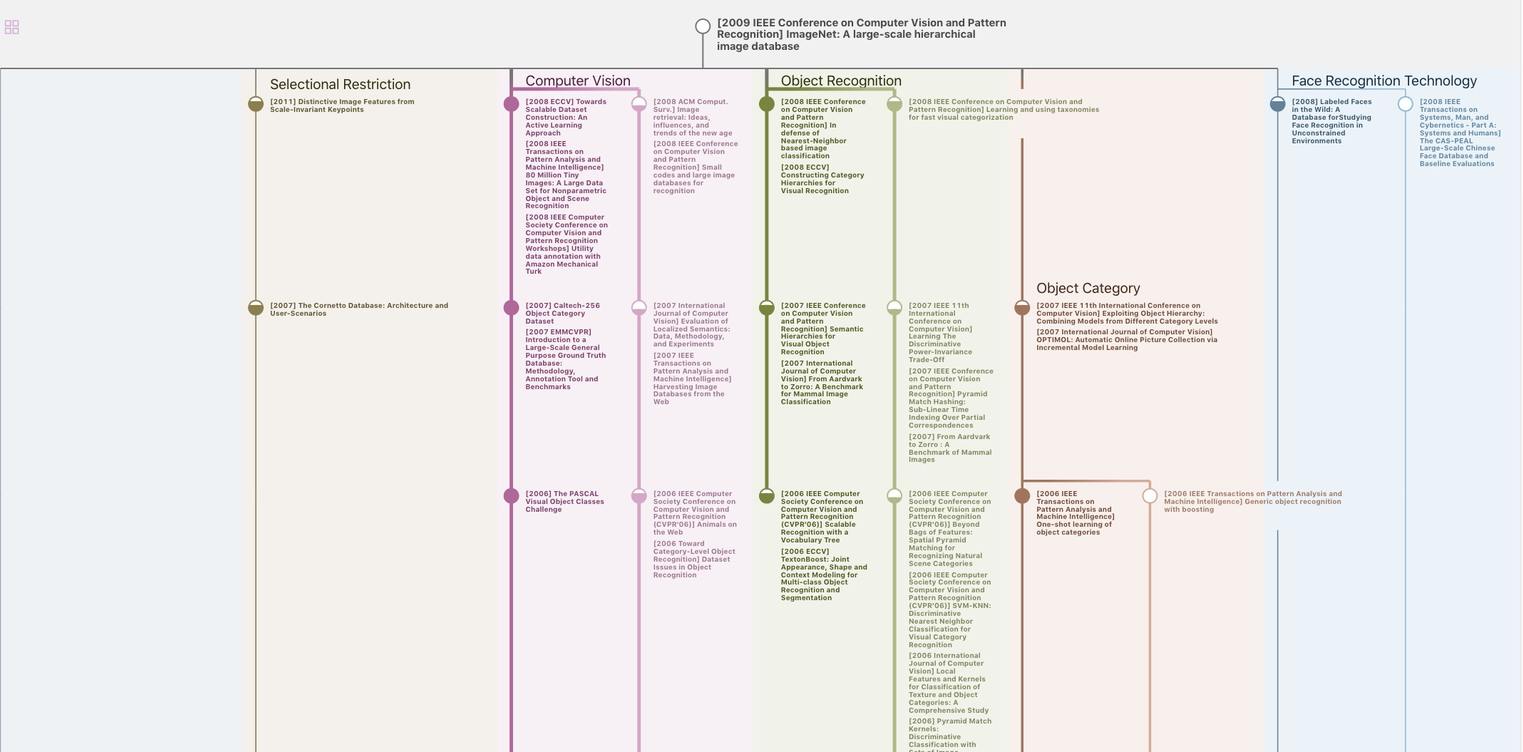
生成溯源树,研究论文发展脉络
Chat Paper
正在生成论文摘要