A combined clustering/symbolic regression framework for fluid property prediction
Physics of Fluids(2022)
摘要
Symbolic regression techniques are constantly gaining ground in materials informatics as the machine learning counterpart capable of providing analytical equations exclusively derived from data. When the feature space is unknown, unsupervised learning is incorporated to discover and explore hidden connections between data points and may suggest a regional solution, specific for a group of data. In this work, we develop a Lennard-Jones fluid descriptor based on density and temperature values and investigate the similarity between data corresponding to diffusion coefficients. Descriptions are linked with the aid of clustering algorithms, which lead to fluid groups with similar behavior, bound to physical laws. Keeping in mind that the fluid data space goes over the gas, liquid, and supercritical states, we compare clustering results to this categorization and found that the proposed methods can detect the gas and liquid states, while distinct supercritical region characteristics are discovered, where fluid density and temperature affect the diffusion coefficient in a more complex way. The incorporation of symbolic regression algorithms on each cluster provides an in-depth investigation on fluid behavior, and regional expressions are proposed. Published under an exclusive license by AlP Publishing.
更多查看译文
关键词
clustering/symbolic regression framework,prediction,property
AI 理解论文
溯源树
样例
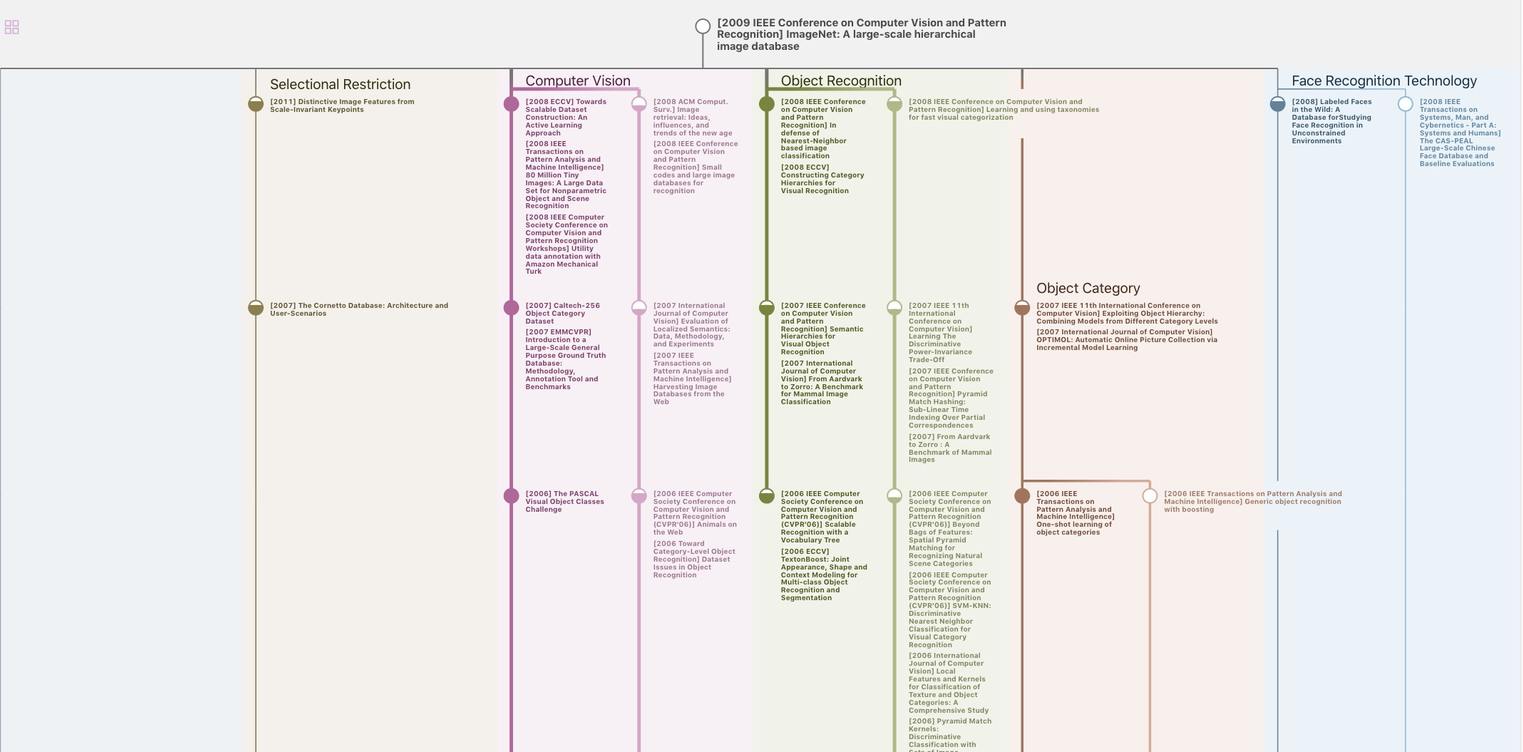
生成溯源树,研究论文发展脉络
Chat Paper
正在生成论文摘要