A Neural-network Enhanced Video Coding Framework beyond VVC.
IEEE Conference on Computer Vision and Pattern Recognition(2022)
摘要
This paper presents a hybrid video compression framework, aiming at providing a demonstration of applying deep learning-based approaches beyond conventional coding framework. The proposed hybrid framework is established over the Enhanced Compression Model (ECM) of which the core is the Versatile Video Coding (VVC) standard. We propose to integrate a series of enhanced coding tools, such as block partitioning, intra prediction, and inter prediction to further remove the spatial and temporal redundancy. Moreover, deep learning-based technologies including loop filter and super resolution are involved to restore the compression distortion. Compared with the VVC software VTM-11.0, experimental results demonstrate the effectiveness of the proposed learning-based framework, leading to 25.81%, 35.08%, and 37.54% bit-rate savings for Y, Cb and Cr components, respectively under random access configuration. In addition, the proposed framework achieves 39.313 and 32.050 PSNRs in the test set under 1 Mbps and 0.1 Mbps video compression tracks of CLIC-2022. 33.522, 30.758, and 28.300 in terms of PSNR are obtained in 0.3 bpp, 0.15 bpp, and 0.075 bpp image compression tracks.
更多查看译文
关键词
VVC software VTM-11,bpp image compression tracks,neural-network,video coding framework,hybrid video compression frame-work,deep learning-based approaches,enhanced compression model,versatile video coding standard,block partitioning,intra prediction,inter prediction,spatial redundancy,temporal redundancy,loop filter,super resolution
AI 理解论文
溯源树
样例
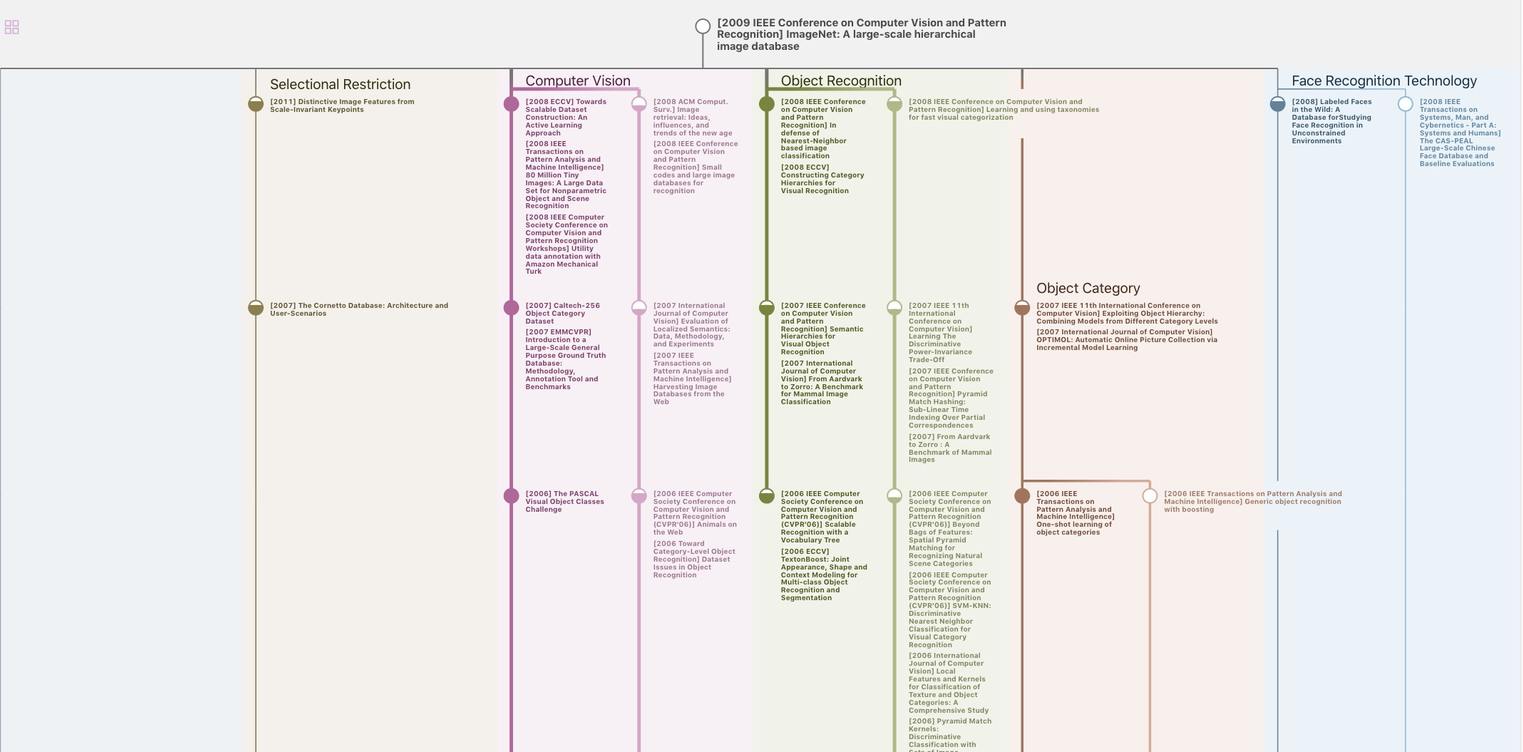
生成溯源树,研究论文发展脉络
Chat Paper
正在生成论文摘要