An Ensemble Learning Recommender System for Interactive Platforms
World Journal of Engineering and Technology(2022)
摘要
In interactive platforms, we often want to predict which items could be more relevant for users, either based on their previous interactions with the system or their preferences. Such systems are called Recommender Systems. They are divided into three main groups, including content-based, collaborative and hybrid recommenders. In this paper, we focus on collaborative filtering and the improvement of the accuracy of its techniques. Then, we suggest an Ensemble Learning Recommender System model made of a probabilistic model and an efficient matrix factorization method. The interactions between users and the platform are scored by explicit and implicit scores. At each user session, implicit scores are used to train a probabilistic model to compute the maximum likelihood estimator for the probability that an item will be recommended in the next session. The explicit scores are used to know the impact of the user’s vote on an item at the time of the recommendation.
更多查看译文
关键词
ensemble learning recommender system,interactive platforms
AI 理解论文
溯源树
样例
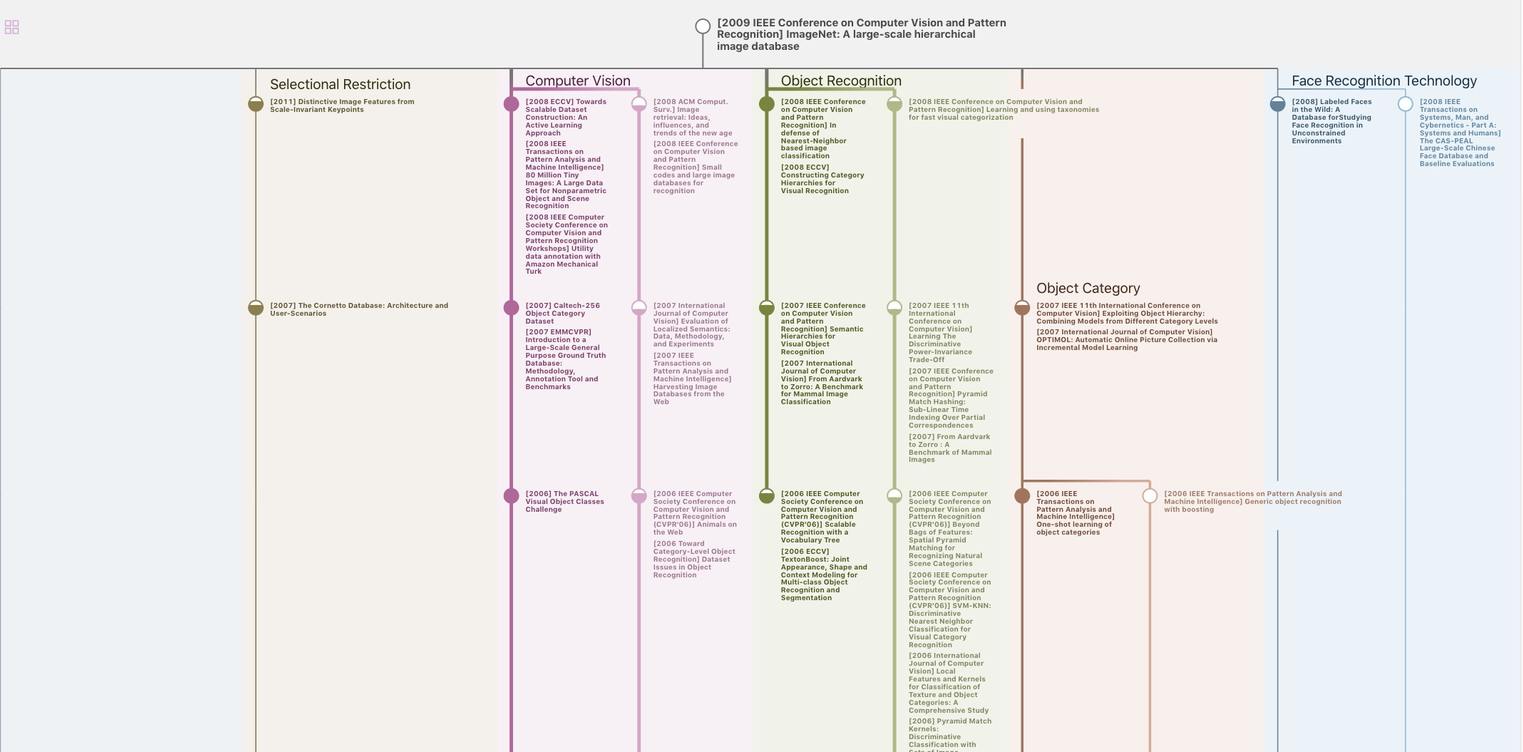
生成溯源树,研究论文发展脉络
Chat Paper
正在生成论文摘要