Optimal Data-Driven Difference-Inversion-Based Iterative Control: High-Speed Nanopositioning Tracking Example
IEEE Transactions on Control Systems Technology(2023)
摘要
In this article, an optimal data-driven difference-inversion-based iterative control (ODDD-IIC) method is proposed for high-speed precision tracking in the presence of dynamics changes and random disturbances. Iterative learning control (ILC) has been shown to be advantageous over feedback and feedforward control for repetitive operations. Challenges, however, still exist to achieve high accuracy and fast convergence in ILCs as the bandwidth, i.e., the frequency range for guaranteed convergence, can be limited by adverse effects of modeling error and random disturbances. The aim of the proposed method is to compensate for these adverse effects through a data-driven approach without a modeling process. A frequency- and iteration-dependent iteration gain is introduced in the control law to enhance both the tracking performance and the robustness. The technique is illustrated in an output tracking experiment on a piezoelectric actuator, with comparison to two existing ILC methods.
更多查看译文
关键词
Convergence,Robustness,System dynamics,Nanopositioning,Data models,Uncertainty,Aerodynamics,Data-driven,iterative learning control (ILC),nanopositioning control,system inversion
AI 理解论文
溯源树
样例
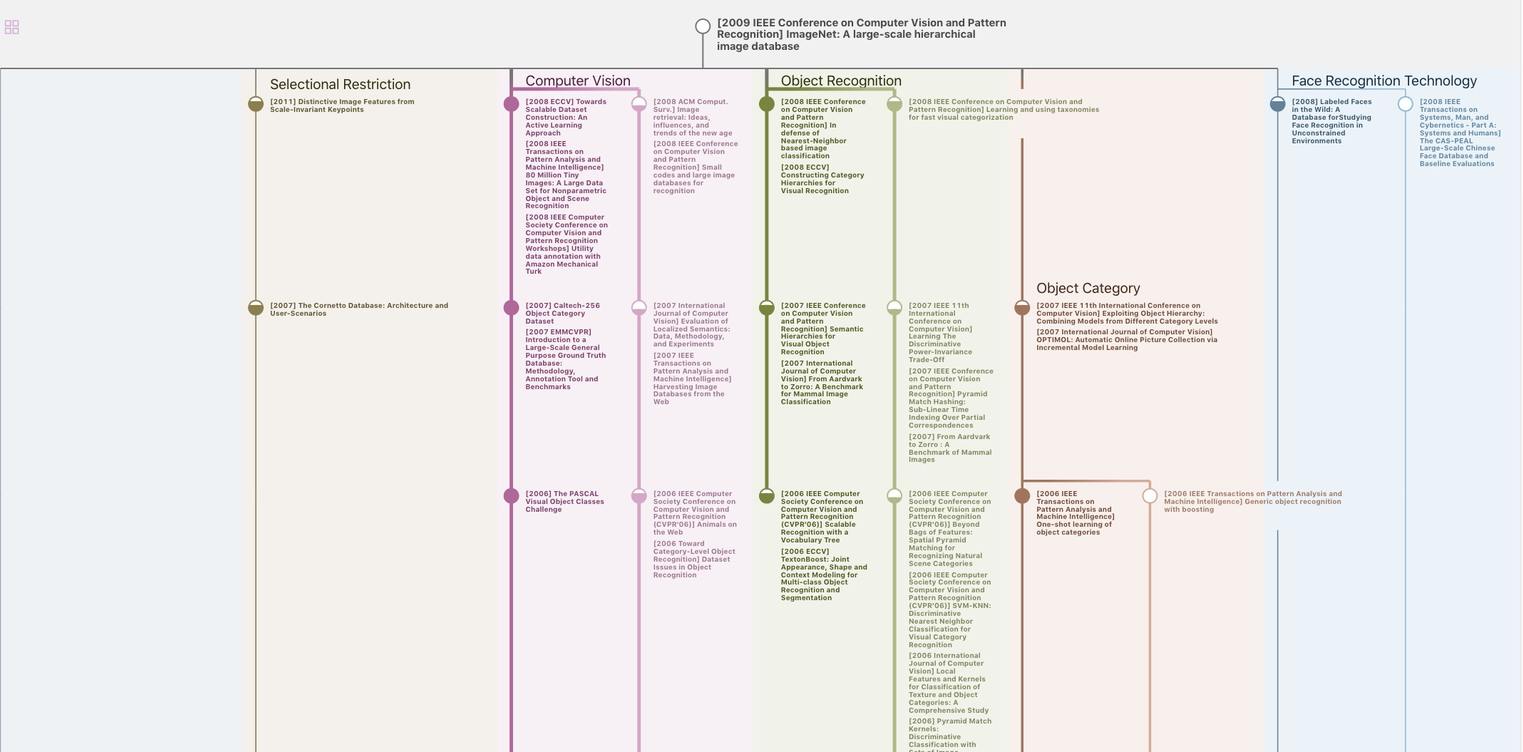
生成溯源树,研究论文发展脉络
Chat Paper
正在生成论文摘要