AUTOMATED DETECTION OF ISOLATED REM SLEEP BEHAVIOR DISORDER (IRBD) DURING SINGLE NIGHT IN-LAB VIDEO-POLYSOMNOGRAPHY (PSG) USING COMPUTER VISION
Sleep(2022)
摘要
Abstract Introduction At least 1% of adults aged 45 and above have isolated REM sleep behavior disorder (iRBD), a prodromal stage of a synucleinopathy, however a majority remains undiagnosed until they develop irreversible neuropathology. The gold-standard diagnostic procedure, the in-lab video-polysomnography (PSG), relies on complex rules for sleep staging and electromyography quantification which potentially lead to incorrect diagnoses. We tested a computer vision classifier for RBD versus other sleep conditions based on automated video analysis of body movements during a single-night in-lab PSG. Methods Raw PSG video recordings of 60 iRBD patients (mean 67 years, 78% males) and 100 controls (mean 65, 65% males) were selected from the Stanford Sleep Center database. Our pipeline involved three main steps: movement detection, feature extraction, and RBD recognition. The recordings between lights off and lights on were 7 to X hours in duration with some interspersed short movements. For every recording, short video segments that contain movements were first extracted using background subtraction. Additional features, such as sleep-onset time and wake-up time, were used to filter out noisy segments that did not involve patients being asleep. Next, I3D, a state-of-the-art method for action recognition, was used to derive a feature vector of 2048 features from every extracted segment. RBD diagnosis could be considered as an action recognition task in which ‘RBD’ was the action category to be classified. For every recording, the feature vectors for every segments were averaged, resulting in one feature vector per subject. With 160 PSG video recordings, this resulted in a dataset of 160 feature vectors that were used to train a multi-layer perceptron (MLP) for RBD recognition. Results Leave-one-out cross-validation (LOOC) procedure was used for evaluation achieving 91.9% accuracy, with a 78.3% sensitivity and 100% precision. Conclusion We tested the feasibility and performance of a new diagnostic paradigm for RBD solely based on single-night video analysis of PSG. Our results suggest that a machine learning model can detect and distinguish the characteristics of movements related to RBD versus other sleep disorders and normal sleep. Larger studies in heterogeneous datasets are needed to validate these findings. Support (If Any) Google
更多查看译文
关键词
irbd,sleep,computer vision,in-lab,video-polysomnography
AI 理解论文
溯源树
样例
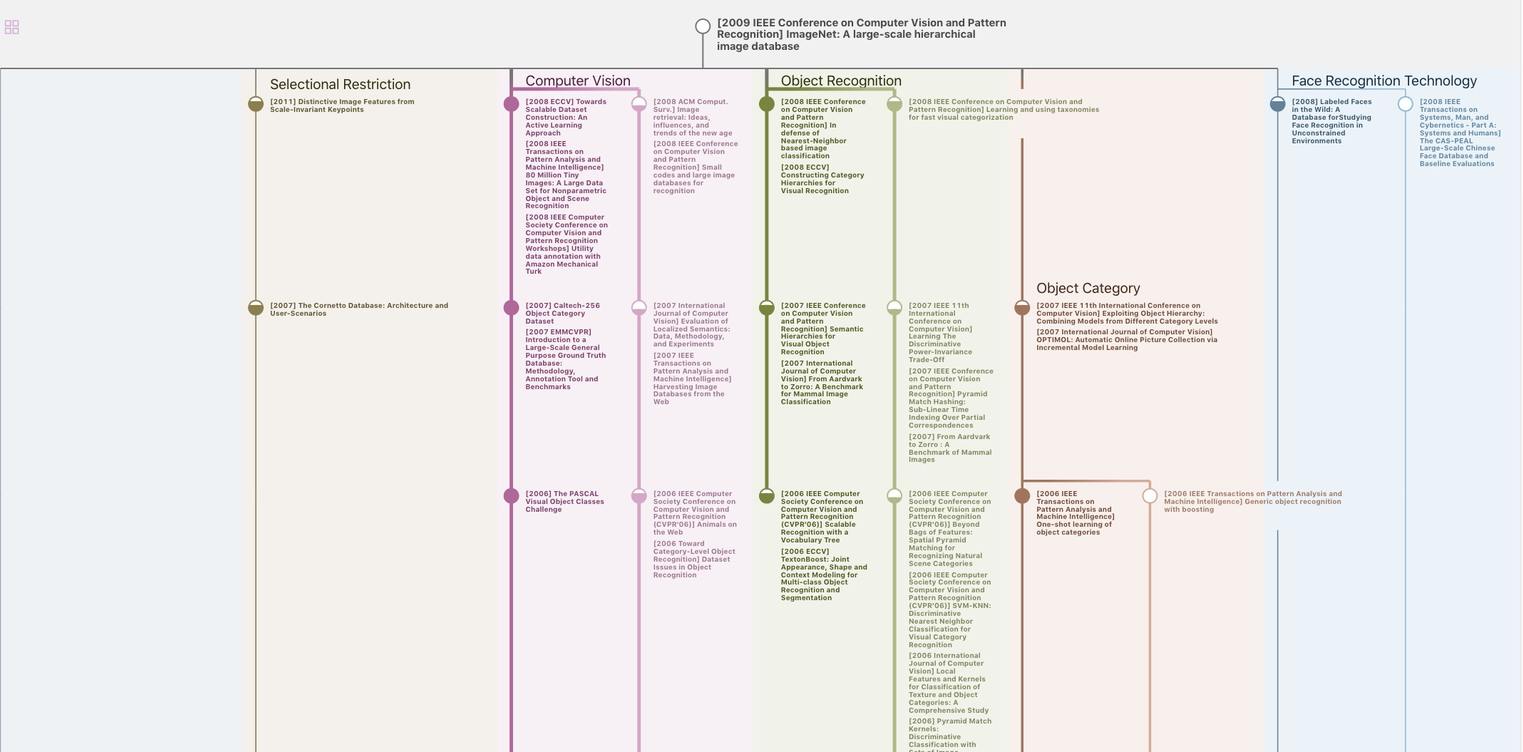
生成溯源树,研究论文发展脉络
Chat Paper
正在生成论文摘要