Link-Based Traffic Estimation and Simulation for Road Networks Using Electronic Registration Identification Data
IEEE Transactions on Vehicular Technology(2022)
摘要
Traffic estimation and traffic simulation are essential parts of the intelligent transportation system. In recent years, massive traffic data has brought many data-based traffic estimation methods, but few are utilized in traffic simulation. The cause is that the data-based traffic simulation has high requirements for data quality, needing the trip information of all vehicles, not met by common traffic data. Fortunately, the electronic registration identification (ERI) data of the vehicle can satisfy. Therefore, we utilize ERI data to study the traffic estimation and simulation for the road network. The core of this research is the travel time estimation model, which is constructed by combining traffic theory models and the deep neural network. The traffic theory models are a group of linear models, which represent the relationship between traffic flow or traffic density and travel time. The deep neural network can extract the temporal and spatial characteristics of the road network traffic by Moving Average Convergence-Divergence (MACD) and Graph Convolutional Network (GCN), respectively. We named the travel time estimation model MGCN. Then, we employed MGCN for traffic simulation. In the experiment section, we employed Chongqing ERI data to verify the research content. Compared with some baseline methods, our method is superior in travel time estimation and traffic simulation.
更多查看译文
关键词
Travel time estimation,traffic simulation,electronic registration identification of the vehicle,graph convolutional network,moving average convergence-divergence
AI 理解论文
溯源树
样例
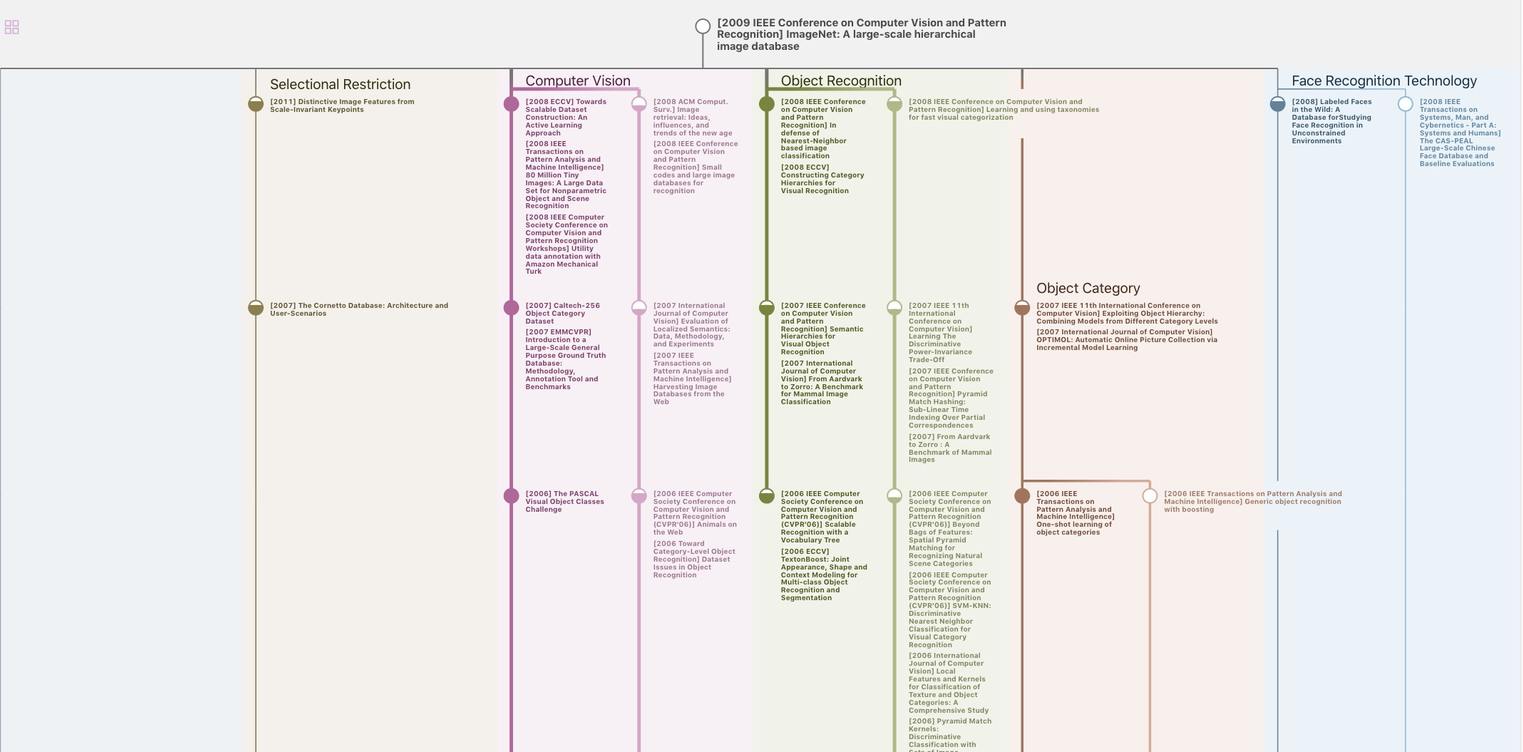
生成溯源树,研究论文发展脉络
Chat Paper
正在生成论文摘要