A Convolutional Neural Network Based on Self-Attention Mechanism for Molecular Property Prediction Using Molecular Hidden Fingerprints: An efficient molecular property prediction method.
2021 The 10th International Conference on Networks, Communication and Computing(2021)
摘要
With the development of artificial intelligence, the project of using deep learning for molecular property prediction has gained much attention. The current widely used molecular description methods, such as Morgan molecular fingerprinting, need further improvement in their molecular description capability, and the current networks widely used for molecular property classification, such as feed forward neural networks (FFN), also have the problem of insufficient molecular feature extraction capability. To address the above problems, we propose the 1DCNN+Self-Attention model, which is applied to the molecular hidden fingerprint obtained by the Directed Message Passing Neural Network (D-MPNN) model processing. Unlike the traditional molecular description methods, this molecular hidden fingerprint is a bond-centered molecular description method, which has shown better molecular description ability after experiments. 1DCNN+Self-Attention model can extract data features better than FFN model and improve the classification effect. The proposed method was validated on four datasets, BBBP (Blood-brain barrier penetration) dataset, ClinTox dataset, SIDER (Side Effect Resource ) dataset, and Tox21 (Toxicology in the 21st Century) dataset, and the AUC index was applied as the network classification ability evaluation index, and the final experimental results showed that the results achieved by the classification method in this paper were better than the classification methods adopted in previous experiments.
更多查看译文
AI 理解论文
溯源树
样例
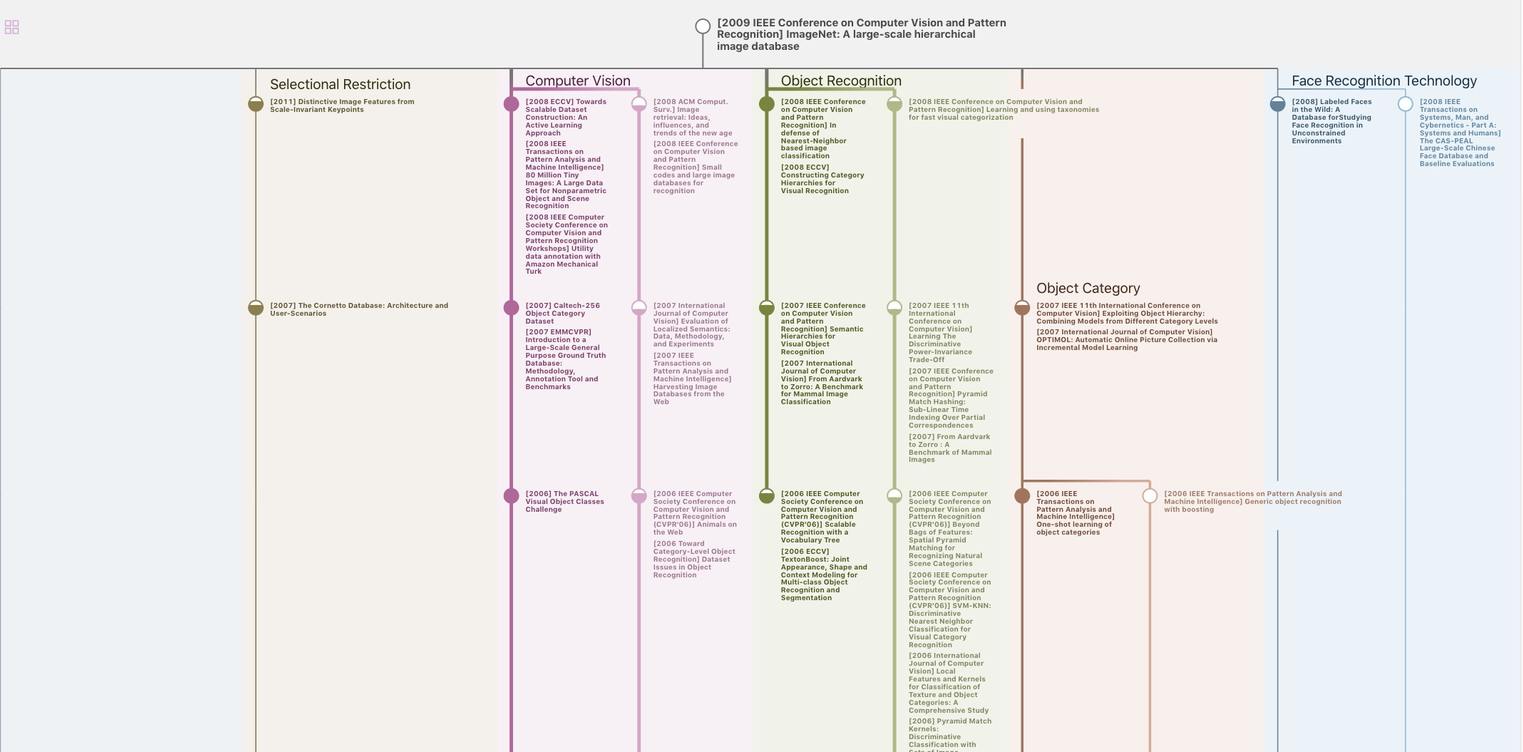
生成溯源树,研究论文发展脉络
Chat Paper
正在生成论文摘要