0082 External Validation of an Enhanced Machine Learning Algorithm: Polysomnography-Based Narcolepsy-Like Feature Assessment and Clinician Notification in Routine Sleep Medicine Clinics
Sleep(2022)
摘要
Abstract Introduction Polysomnography (PSG) contains quantitative information that, using machine learning (ML) algorithms, may aid the identification of type 1 narcolepsy. This study aimed to evaluate the utility of a previously developed quantitative tool (ML evaluation of PSG data) for detecting narcolepsy (types 1 and 2) in a “real-world” sleep clinic population. Methods Nocturnal PSG studies from a random sample of sleep clinic patients (narcolepsy, n=302; controls, n=21,535) were randomly split (1:1) into a training set and a validation set for algorithm testing. A separate, external PSG dataset was used for additional testing of the final model. Sleep stage probability graphs (hypnodensities) were estimated from PSGs on 15-second epochs using a previously developed convolutional neural network. Feature engineering was applied to hypnodensities to create a feature vector that was used to train a Gaussian process (GP) model to identify patients with a high probability of having narcolepsy. Features could be scaled to the 85th percentile, zero-mean and unit-variance, or unscaled. A recursive feature-elimination scheme was compared with training the GP kernel’s length scale for determining the subset of features that best discriminate narcolepsy and controls. A synthetic minority oversampling technique was applied in combination with random undersampling to balance the distribution of cases and controls in the training set. Several kernels and relevant hyperparameters were evaluated. Model performance (specificity and sensitivity) was examined using receiver operating characteristics with the goal of achieving an area under the curve (AUC) ≥0.80. Results The final GP model used a Matérn 5/2 covariance kernel with the length scale hyperparameter trained to determine the feature subset selection. Input features were normalized to zero-mean and unit-variance. The model had AUC=0.9960 and AUC=0.8014 for classifying narcolepsy in the training and validation sets, respectively. Sensitivity ranged from 73% to 65% when specificity was between 75% and 80%. Final performance evaluation of the model for classifying narcolepsy in an external PSG dataset is ongoing; results will be presented at the congress. Conclusion An ML-based algorithm can offer an objective, sensitive, and specific tool for alerting sleep clinicians about patients at risk for narcolepsy, using nocturnal PSG in general sleep medicine clinics. Support (If Any) Jazz Pharmaceuticals.
更多查看译文
AI 理解论文
溯源树
样例
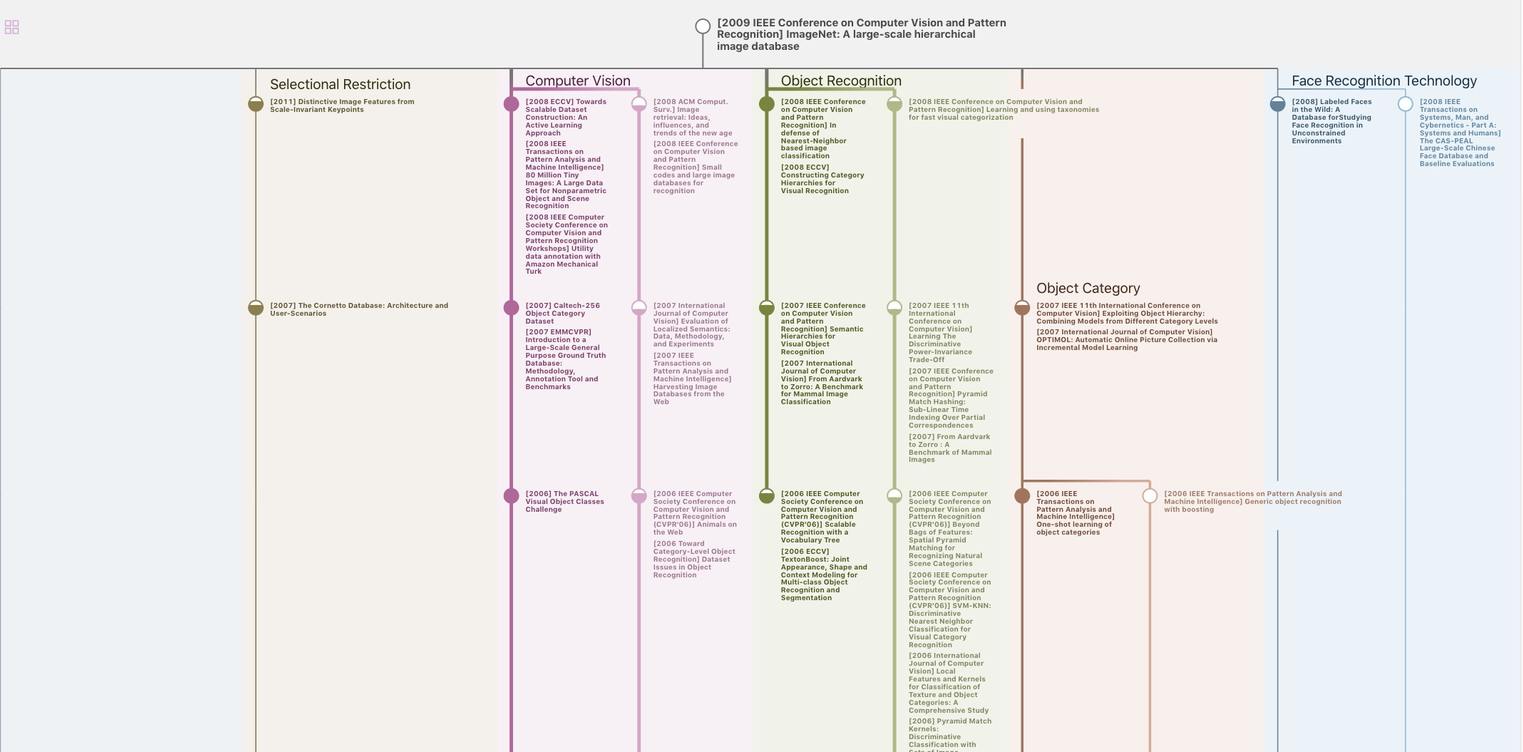
生成溯源树,研究论文发展脉络
Chat Paper
正在生成论文摘要