PD-0904 Clinical experiences with online adaptive radiotherapy of vulvar carcinoma
Radiotherapy and Oncology(2022)
摘要
Radiotherapy for vulvar carcinoma is challenging due to relatively high risk of locoregional disease recurrence, a technically challenging target, and postoperative lymphocele, and a high risk radiation sequelae. We aim to explore, if it is possible to reduce dose to normal tissue, while maintaining CTV coverage for this patient group with online adaptive radiotherapy.20 patients with vulvar carcinoma (527 fractions) were treated with online adaptation on a Varian Ethos accelerator. Setup CBCTs were acquired daily for adaptive planning. Verification CBCTs were acquired immediately prior to dose delivery. CTV dose coverage and dose to bladder and rectum were extracted from the scheduled and adapted plans as well as from adapted plans recalculated based on verification CBCTs. In addition, analysis of the decision of the adaptive procedure was performed for 17 patients (465 fractions).Mean CTV D95% and standard deviation was 98% ± 5% for the scheduled plan compared to 100.0 ± 0.3% and 100.0 ± 0.8% for the adapted plan on the setup and verification CBCT respectively. Dose to OARs varied substantially and did not show any benefit from adaption itself, however a margin reduction was implemented after the first patients treated. The adapted plan was chosen for 63.5% of the fractions and dominant reasons for not adapting were 'no significant dosimetric gain' (75 fractions, 14%) and 'Medical doctor (MD) not available for treatment' (50 fractions, 9.5%). The median adaption time was 15 min and the 25th and 75th percentile was 12 and 17 min, respectively.CTVs and PTVs dose coverage were significantly improved with adaptation compared to image-guided RT. This gain was robust during the treatment time.
更多查看译文
关键词
online adaptive radiotherapy,carcinoma,clinical experiences
AI 理解论文
溯源树
样例
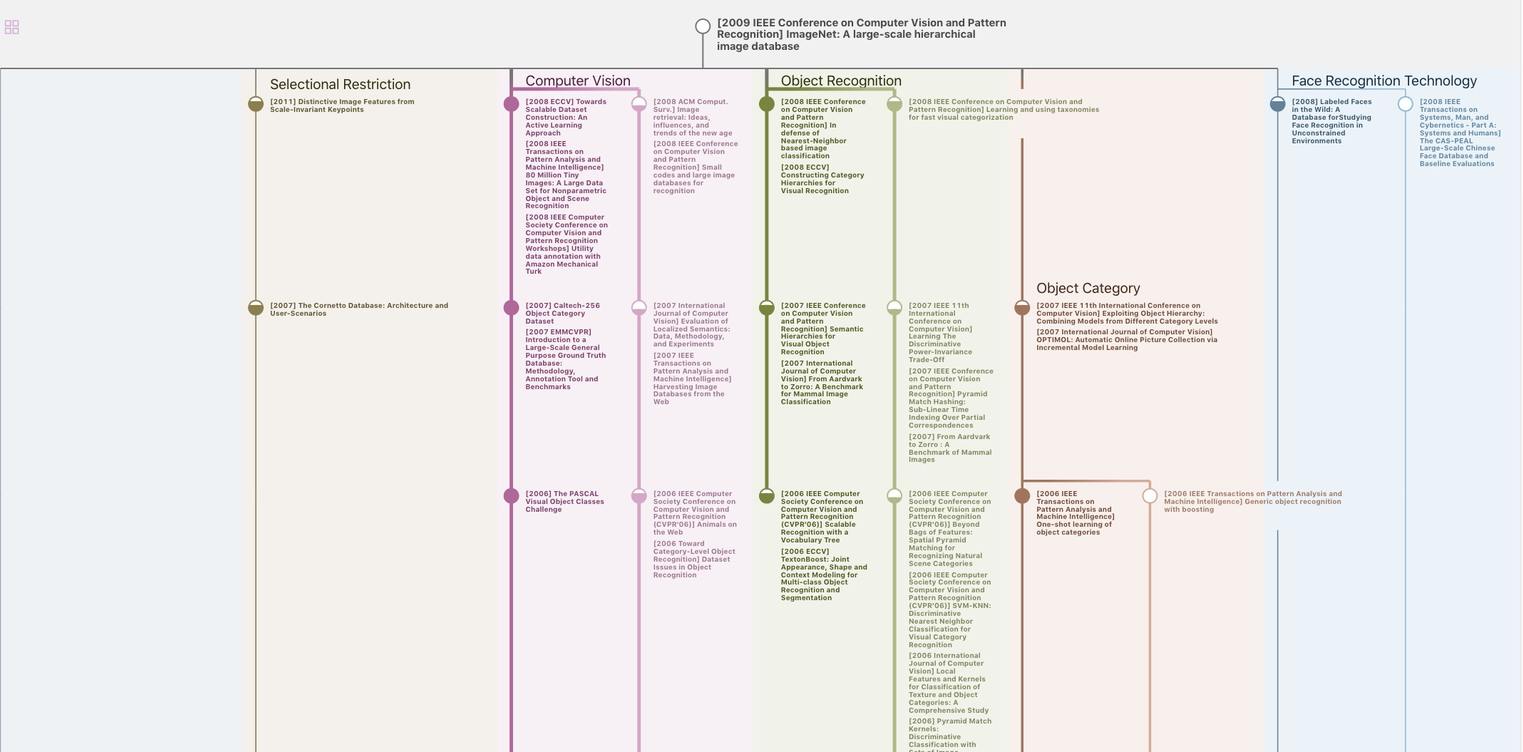
生成溯源树,研究论文发展脉络
Chat Paper
正在生成论文摘要