The recognition of 3-phase power quality events using optimal feature selection and random forest classifier.
International Journal of Computational Vision and Robotics(2023)
摘要
This article proposes a novel feature vector by combining the K-means apriori feature selection (KAFS) algorithm and statistical technique to classify 3-phase power quality disturbance (PQD) events. While the K-means algorithm has clustered the raw signals, the apriori algorithm is capable of fetching the desired discriminative features of the chosen PQD events. Further, these discriminative features extracted have been utilised to compute nine statistical parameters. The reliability of the novel feature vector has been measured in classifying the 3-phase PQD events with similar statistical parameters obtained from the raw PQD samples. Finally, the ability of the short-time Fourier transform (STFT) as a time-frequency tool has been evaluated using the KAFS algorithms for the said task. The random forest (RF) classifier is chosen to validate the efficacy of the proposed feature vectors. The novel optimised feature vectors using the KAFS have indeed enhanced the recognition accuracy as revealed from our results.
更多查看译文
关键词
power quality,feature selection,classification,recognition accuracy,random forest algorithm
AI 理解论文
溯源树
样例
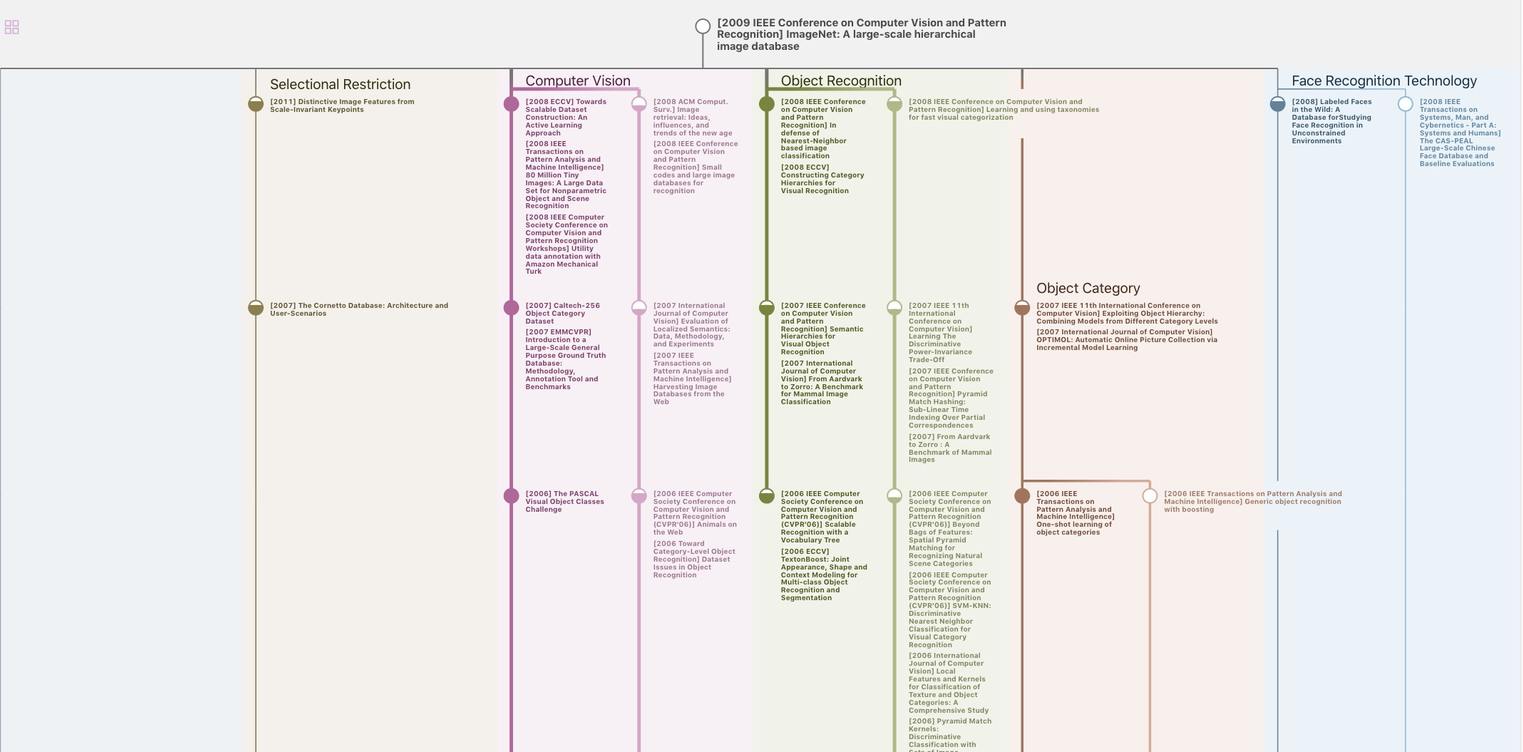
生成溯源树,研究论文发展脉络
Chat Paper
正在生成论文摘要