Boosting the interpretability of clinical risk scores with intervention predictions
arxiv(2022)
摘要
Machine learning systems show significant promise for forecasting patient adverse events via risk scores. However, these risk scores implicitly encode assumptions about future interventions that the patient is likely to receive, based on the intervention policy present in the training data. Without this important context, predictions from such systems are less interpretable for clinicians. We propose a joint model of intervention policy and adverse event risk as a means to explicitly communicate the model's assumptions about future interventions. We develop such an intervention policy model on MIMIC-III, a real world de-identified ICU dataset, and discuss some use cases that highlight the utility of this approach. We show how combining typical risk scores, such as the likelihood of mortality, with future intervention probability scores leads to more interpretable clinical predictions.
更多查看译文
关键词
clinical risk scores,interpretability,predictions
AI 理解论文
溯源树
样例
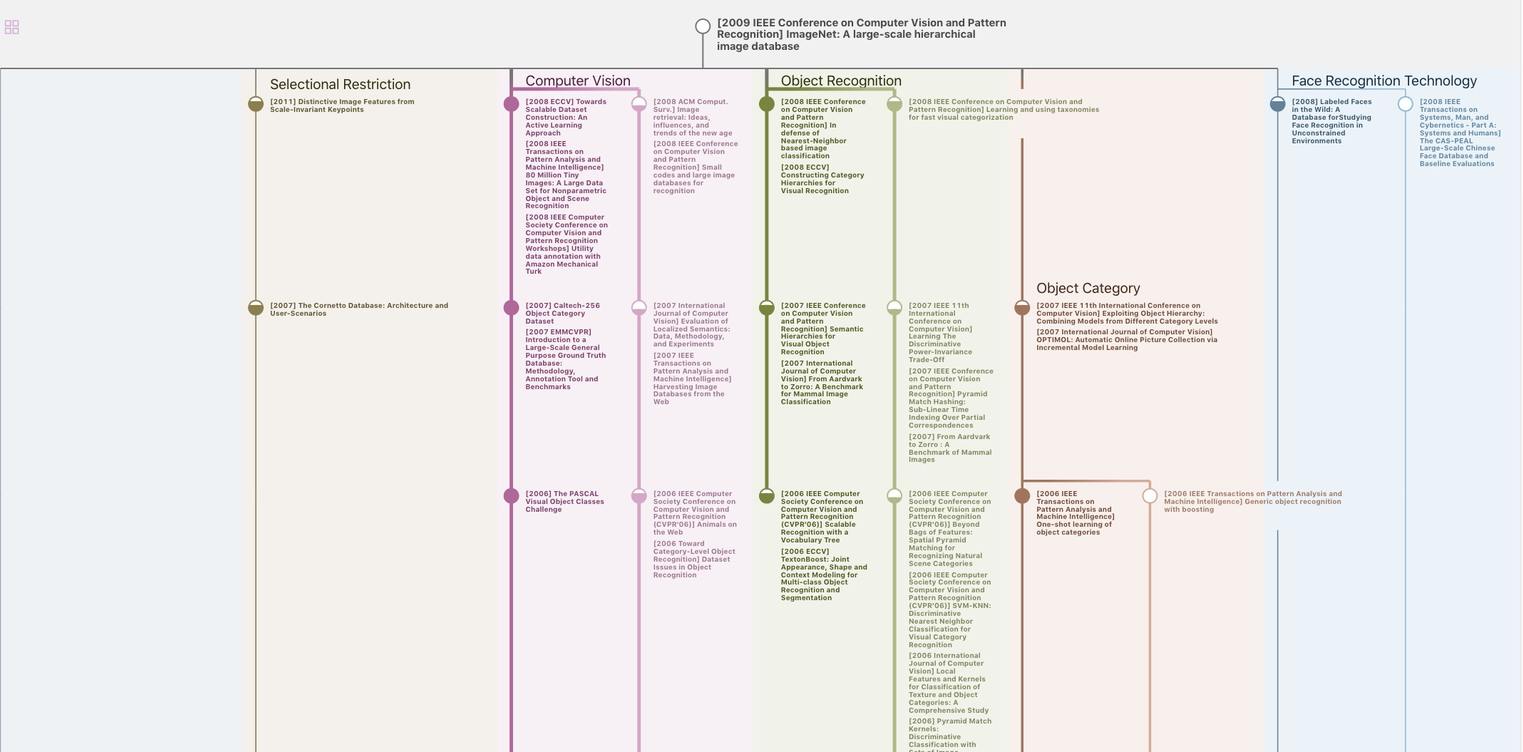
生成溯源树,研究论文发展脉络
Chat Paper
正在生成论文摘要