Kronecker product approximation of operators in spectral norm via alternating sdp
SIAM JOURNAL ON MATRIX ANALYSIS AND APPLICATIONS(2023)
摘要
The decomposition or approximation of a linear operator on a matrix space as a sum of Kronecker products plays an important role in matrix equations and low-rank modeling. The approximation problem in Frobenius norm admits a well-known solution via the singular value decomposition. However, the approximation problem in spectral norm, which is more natural for linear operators, is much more challenging. In particular, the Frobenius norm solution can be far from optimal in spectral norm. We describe an alternating optimization method based on semidefinite programming to obtain high-quality approximations in spectral norm, and we present computational experiments to illustrate the advantages of our approach.
更多查看译文
关键词
operator approximation,Kronecker product,semidefinite programming,alternating optimization,matrix equations
AI 理解论文
溯源树
样例
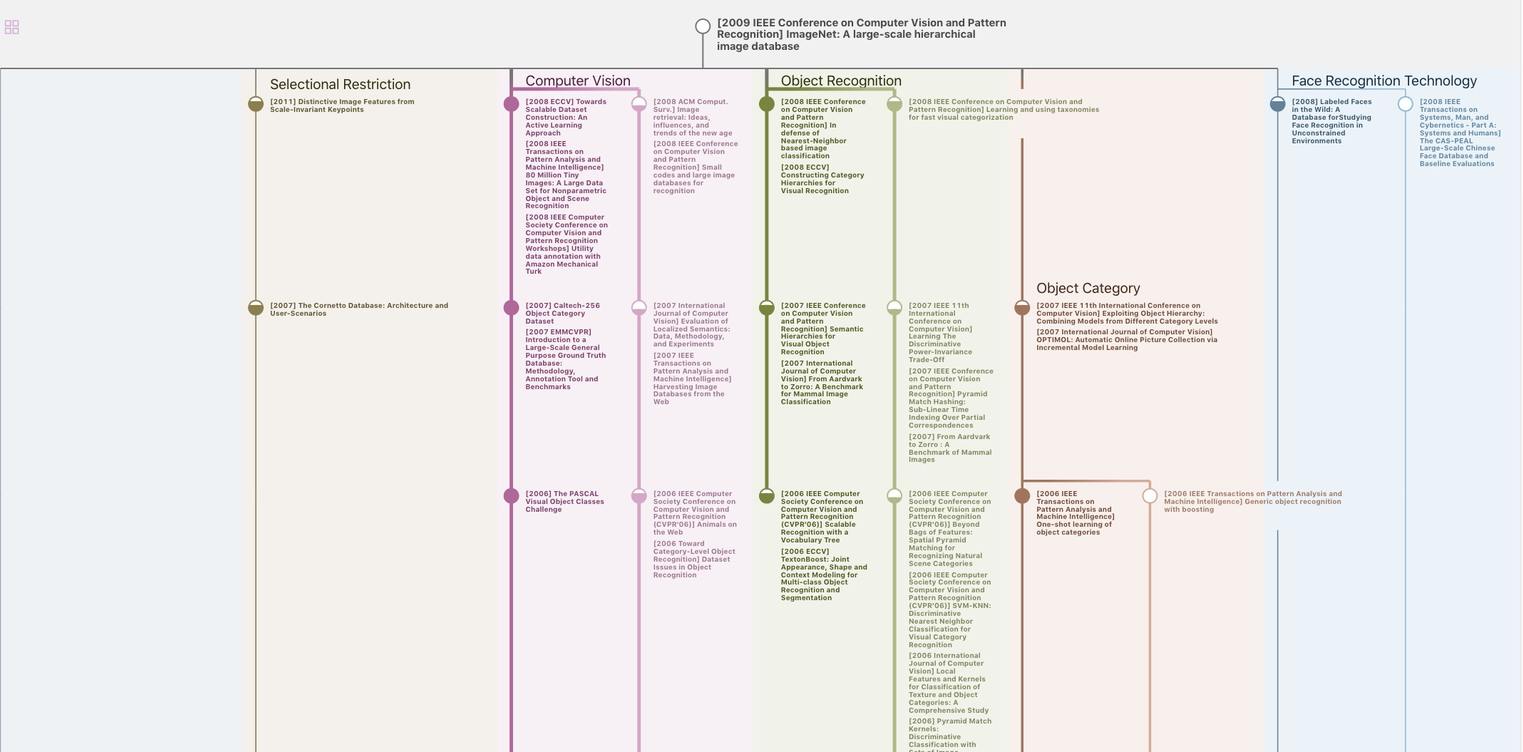
生成溯源树,研究论文发展脉络
Chat Paper
正在生成论文摘要