An Explainable AI Approach for the Rapid Diagnosis of COVID-19 Using Ensemble Learning Algorithms
FRONTIERS IN PUBLIC HEALTH(2022)
摘要
Background: Artificial intelligence-based disease prediction models have a greater potential to screen COVID-19 patients than conventional methods. However, their application has been restricted because of their underlying black-box nature. Objective: To addressed this issue, an explainable artificial intelligence (XAI) approach was developed to screen patients for COVID-19. Methods: A retrospective study consisting of 1,737 participants (759 COVID-19 patients and 978 controls) admitted to San Raphael Hospital (OSR) from February to May 2020 was used to construct a diagnosis model. Finally, 32 key blood test indices from 1,374 participants were used for screening patients for COVID-19. Four ensemble learning algorithms were used: random forest (RF), adaptive boosting (AdaBoost), gradient boosting decision tree (GBDT), and extreme gradient boosting (XGBoost). Feature importance fromthe perspective of the clinical domain and visualized interpretations were illustrated by using local interpretable model-agnostic explanations (LIME) plots. Results: The GBDT model [area under the curve (AUC): 86.4%; 95% confidence interval (CI) 0.821-0.907] outperformed the RF model (AUC: 85.7%; 95% CI 0.813-0.902), AdaBoost model (AUC: 85.4%; 95% CI 0.810-0.899), and XGBoost model (AUC: 84.9%; 95% CI 0.803-0.894) in distinguishing patients with COVID-19 from those without. The cumulative feature importance of lactate dehydrogenase, white blood cells, and eosinophil counts was 0.145, 0.130, and 0.128, respectively. Conclusions: Ensemble machining learning (ML) approaches, mainly GBDT and LIME plots, are efficient for screening patients with COVID-19 and might serve as a potential tool in the auxiliary diagnosis of COVID-19. Patients with higher WBC count, higher LDH level, or higher EOT count, were more likely to have COVID-19.
更多查看译文
关键词
artificial intelligence, ensemble learning, explainable, disease prediction, COVID-19
AI 理解论文
溯源树
样例
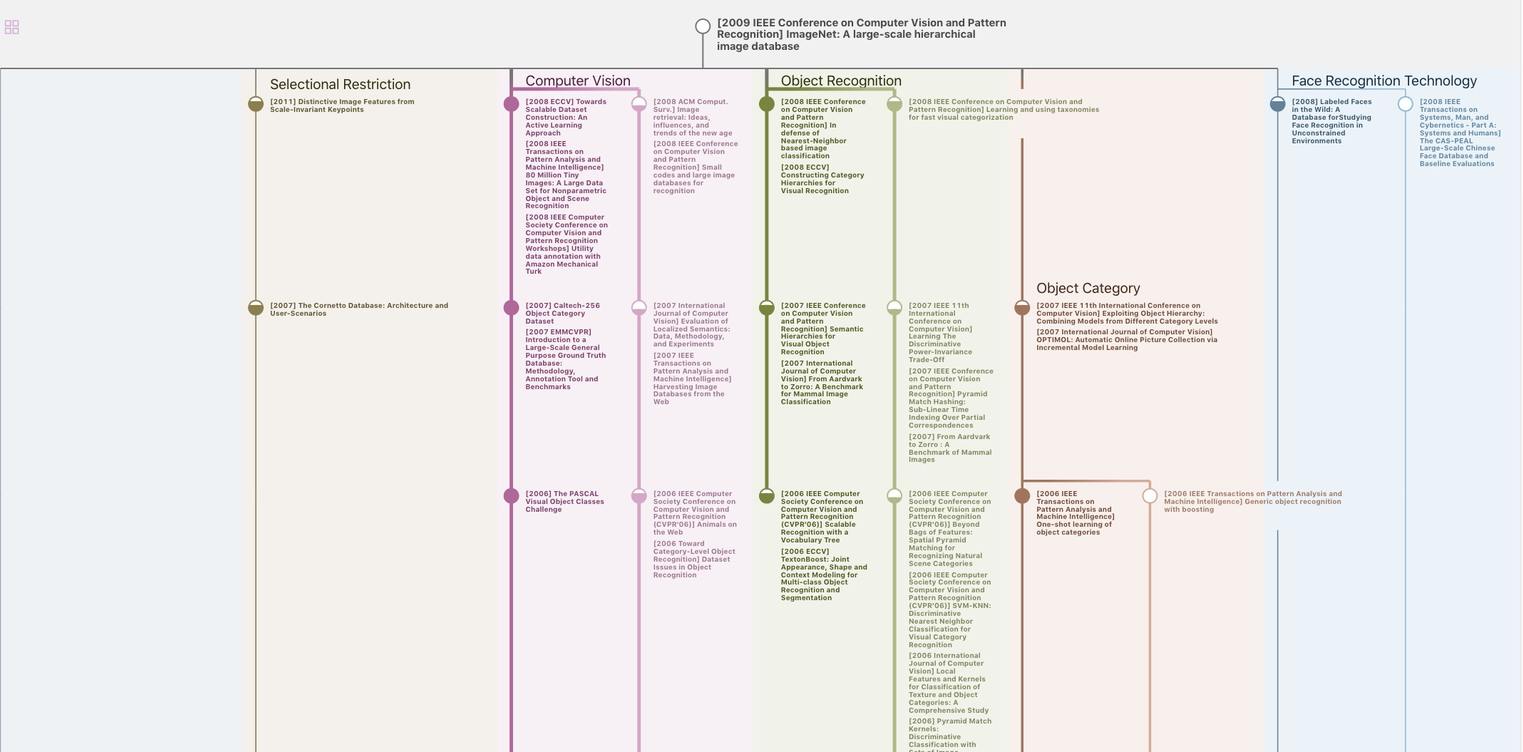
生成溯源树,研究论文发展脉络
Chat Paper
正在生成论文摘要