JAN: Joint Attention Networks for Automatic ICD Coding
IEEE Journal of Biomedical and Health Informatics(2022)
摘要
The International Classification of Diseases (ICD) code is a disease classification method formulated by the World Health Organization(WHO). ICD coding usually requires clinicians to manually allocate ICD codes to clinical documents, which is labor-intensive, expensive, and error-prone. Therefore, many methods have been introduced for automatic ICD coding. However, most of the methods have ignored or cannot combine two essential features well: long-tailed label distribution and label correlation. In this paper, we propose a novel end-to-end Joint Attention Network (JAN) to solve these two problems. JAN includes Document-based attention and Label-based attention to capture semantic information from clinical document text and label description, respectively, which helps solve the classification of dense and sparse data in long-tailed label distribution. Besides, an Adaptive fusion layer and CorNet block are presented to adaptively adjust the weight of these two attentions and exploit label co-occurrence relations, respectively. Experiments on the MIMIC-III and MIMIC-II datasets demonstrate that our proposed JAN outperformed previous state-of-art methods achieving Micro-F1 of 0.553, Micro-AUC of 0.989 and precision at top 8(P@8) of 0.735. Finally, we also provide attention and label correlation visualization to verify the effectiveness of our model and improve the interpretation of our deep learning-based method.
更多查看译文
关键词
Automatic ICD coding,attention network,long-tailed label distribution,label correlation
AI 理解论文
溯源树
样例
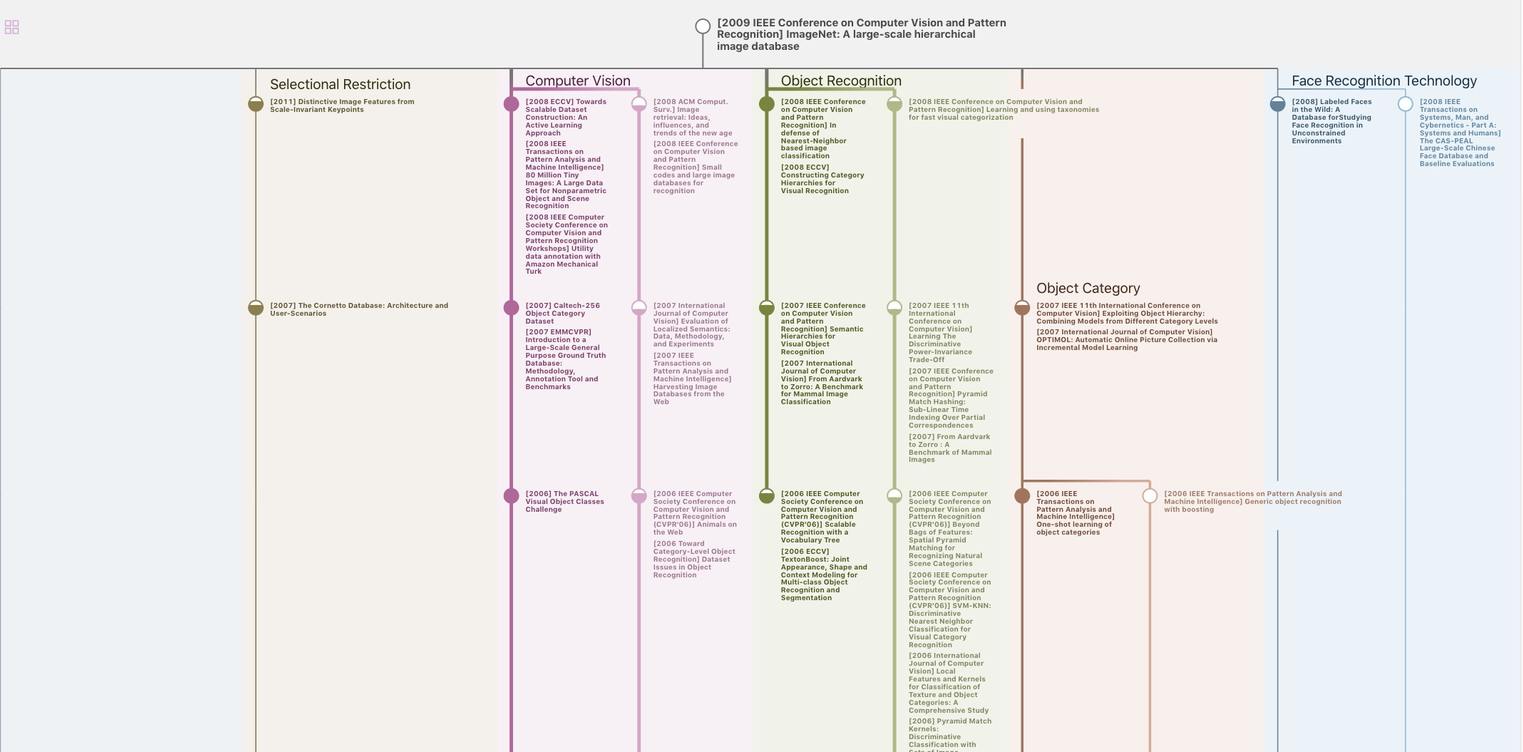
生成溯源树,研究论文发展脉络
Chat Paper
正在生成论文摘要