Weakly supervised surface defect classification network based on Gaussian model
2022 7th International Conference on Intelligent Computing and Signal Processing (ICSP)(2022)
摘要
Due to the complex morphological features of surface defects, algorithms using the traditional hand-crafted features cannot achieve high detection accuracy. Deep learning-based methods have achieved higher accuracy than traditional methods, so deep learning algorithm has been greatly developed in the field of surface defect detection. However, these algorithms need to label a large amount of fine sample information, which requires a lot of human labor, and the labeling results directly affect the accuracy of final prediction, thus limiting the development of deep learning algorithms in this field. To address the above problems, we propose a weakly supervised surface defect classification neural network, which uses resnet50 as the backbone feature extraction network. We design a feature cascade aggregation module and a 2D Gaussian module to set different weights for different regions. These modules allow the neural network to notice defect locations more quickly. Thus, the number of finely labeled samples is reduced, and high classification accuracy is obtained. Experimental results show that the proposed algorithm achieves excellent performance on the public dataset and the homemade dataset. Compared to other methods, our proposed algorithm achieves better or similar accuracy, but is quite faster.
更多查看译文
关键词
Weakly supervised,surface defect classification,deep learning
AI 理解论文
溯源树
样例
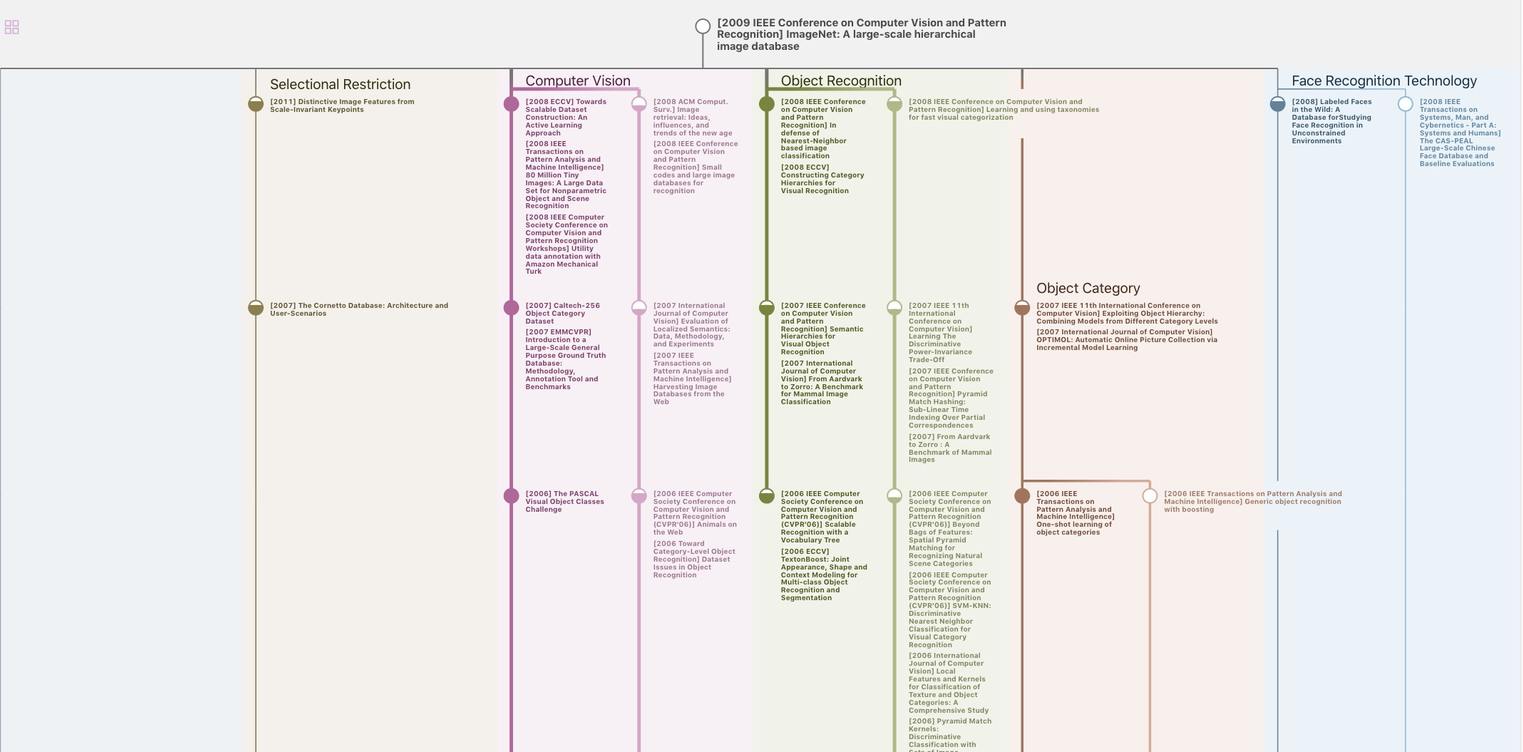
生成溯源树,研究论文发展脉络
Chat Paper
正在生成论文摘要