Policy Optimization for Markovian Jump Linear Quadratic Control: Gradient Method and Global Convergence
IEEE Transactions on Automatic Control(2023)
摘要
Recently, policy optimization has received renewed attention from the control community due to various applications in reinforcement learning tasks. In this article, we investigate the global convergence of the gradient method for quadratic optimal control of discrete-time Markovian jump linear systems (MJLS). First, we study the optimization landscape of direct policy optimization for MJLS, with static-state feedback controllers and quadratic performance costs. Despite the nonconvexity of the resultant problem, we are still able to identify several useful properties such as coercivity, gradient dominance, and smoothness. Based on these properties, we prove that the gradient method converges to the optimal-state feedback controller for MJLS at a linear rate if initialized at a controller, which is mean-square stabilizing. This article brings new insights for understanding the performance of the policy gradient method on the Markovian jump linear quadratic control problem.
更多查看译文
关键词
Markovian jump linear systems (MJLS),optimal control,policy gradient methods,reinforcement learning (RL)
AI 理解论文
溯源树
样例
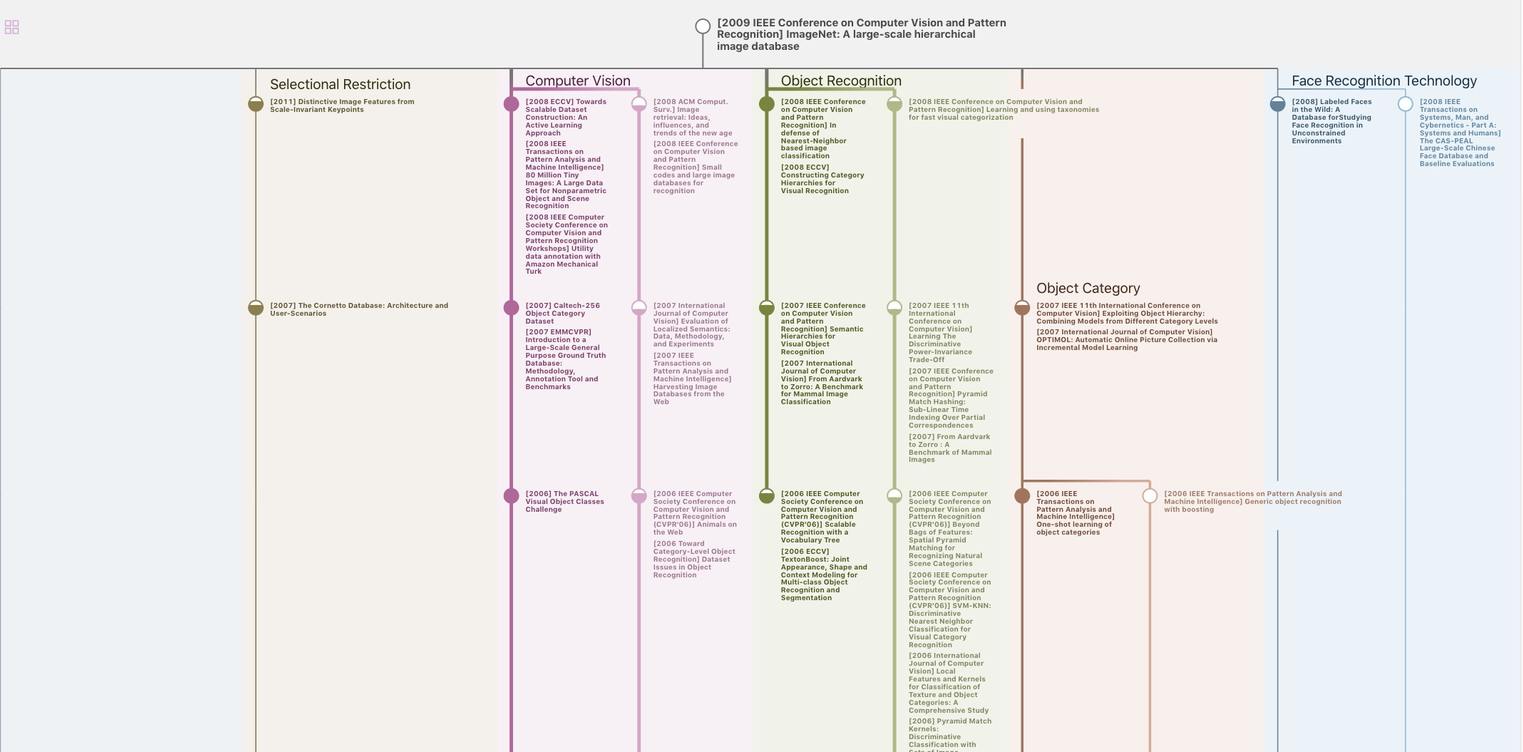
生成溯源树,研究论文发展脉络
Chat Paper
正在生成论文摘要