Stochastic Approximation with Decision-Dependent Distributions: Asymptotic Normality and Optimality
JOURNAL OF MACHINE LEARNING RESEARCH(2024)
摘要
We analyze a stochastic approximation algorithm for decision -dependent problems, wherein the data distribution used by the algorithm evolves along the iterate sequence. The primary examples of such problems appear in performative prediction and its multiplayer extensions. We show that under mild assumptions, the deviation between the average iterate of the algorithm and the solution is asymptotically normal, with a covariance that clearly decouples the effects of the gradient noise and the distributional shift. Moreover, building on the work of H ' ajek and Le Cam, we show that the asymptotic performance of the algorithm with averaging is locally minimax optimal.
更多查看译文
关键词
stochastic approximation,decision-dependent distributions,performative prediction,asymptotic normality,local asymptotic minimax optimality
AI 理解论文
溯源树
样例
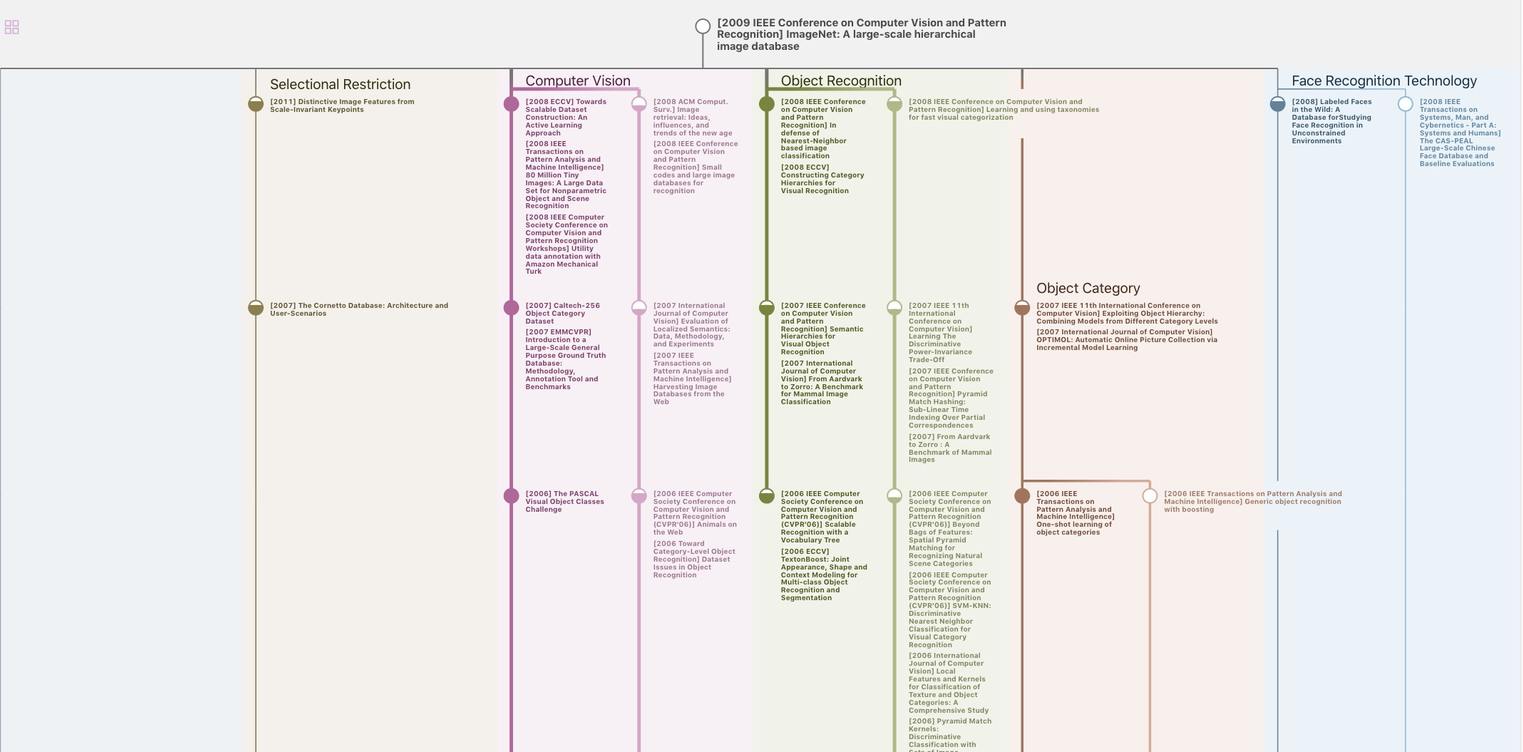
生成溯源树,研究论文发展脉络
Chat Paper
正在生成论文摘要