Noisy Heuristics NAS: A Network Morphism based Neural Architecture Search using Heuristics
CoRR(2022)
摘要
Network Morphism based Neural Architecture Search (NAS) is one of the most efficient methods, however, knowing where and when to add new neurons or remove dis-functional ones is generally left to black-box Reinforcement Learning models. In this paper, we present a new Network Morphism based NAS called Noisy Heuristics NAS which uses heuristics learned from manually developing neural network models and inspired by biological neuronal dynamics. Firstly, we add new neurons randomly and prune away some to select only the best fitting neurons. Secondly, we control the number of layers in the network using the relationship of hidden units to the number of input-output connections. Our method can increase or decrease the capacity or non-linearity of models online which is specified with a few meta-parameters by the user. Our method generalizes both on toy datasets and on real-world data sets such as MNIST, CIFAR-10, and CIFAR-100. The performance is comparable to the hand-engineered architecture ResNet-18 with the similar parameters.
更多查看译文
关键词
neural architecture
AI 理解论文
溯源树
样例
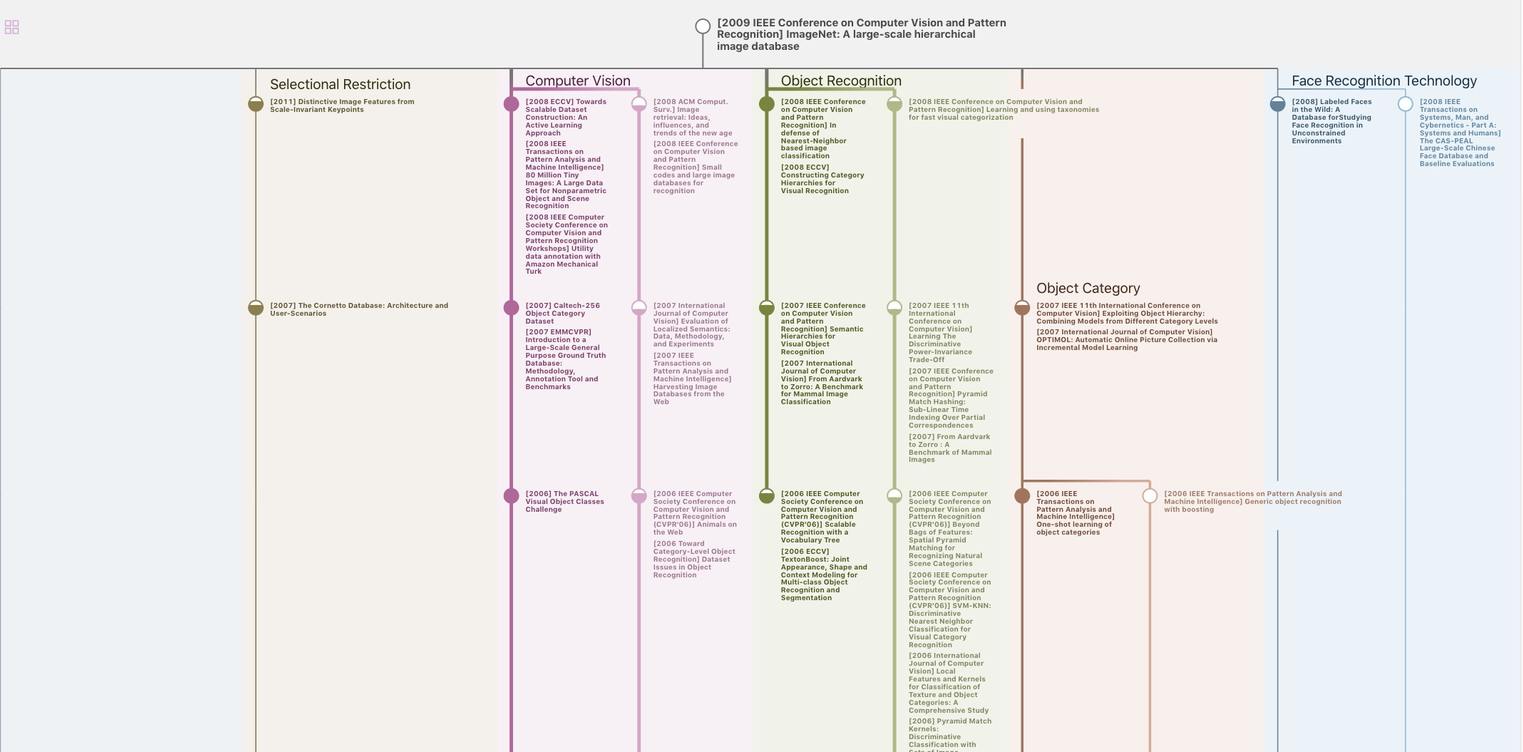
生成溯源树,研究论文发展脉络
Chat Paper
正在生成论文摘要