Interpretability by design using computer vision for behavioral sensing in child and adolescent psychiatry
arxiv(2022)
摘要
Observation is an essential tool for understanding and studying human behavior and mental states. However, coding human behavior is a time-consuming, expensive task, in which reliability can be difficult to achieve and bias is a risk. Machine learning (ML) methods offer ways to improve reliability, decrease cost, and scale up behavioral coding for application in clinical and research settings. Here, we use computer vision to derive behavioral codes or concepts of a gold standard behavioral rating system, offering familiar interpretation for mental health professionals. Features were extracted from videos of clinical diagnostic interviews of children and adolescents with and without obsessive-compulsive disorder. Our computationally-derived ratings were comparable to human expert ratings for negative emotions, activity-level/arousal and anxiety. For the attention and positive affect concepts, our ML ratings performed reasonably. However, results for gaze and vocalization indicate a need for improved data quality or additional data modalities.
更多查看译文
关键词
adolescent psychiatry,computer vision,behavioral sensing
AI 理解论文
溯源树
样例
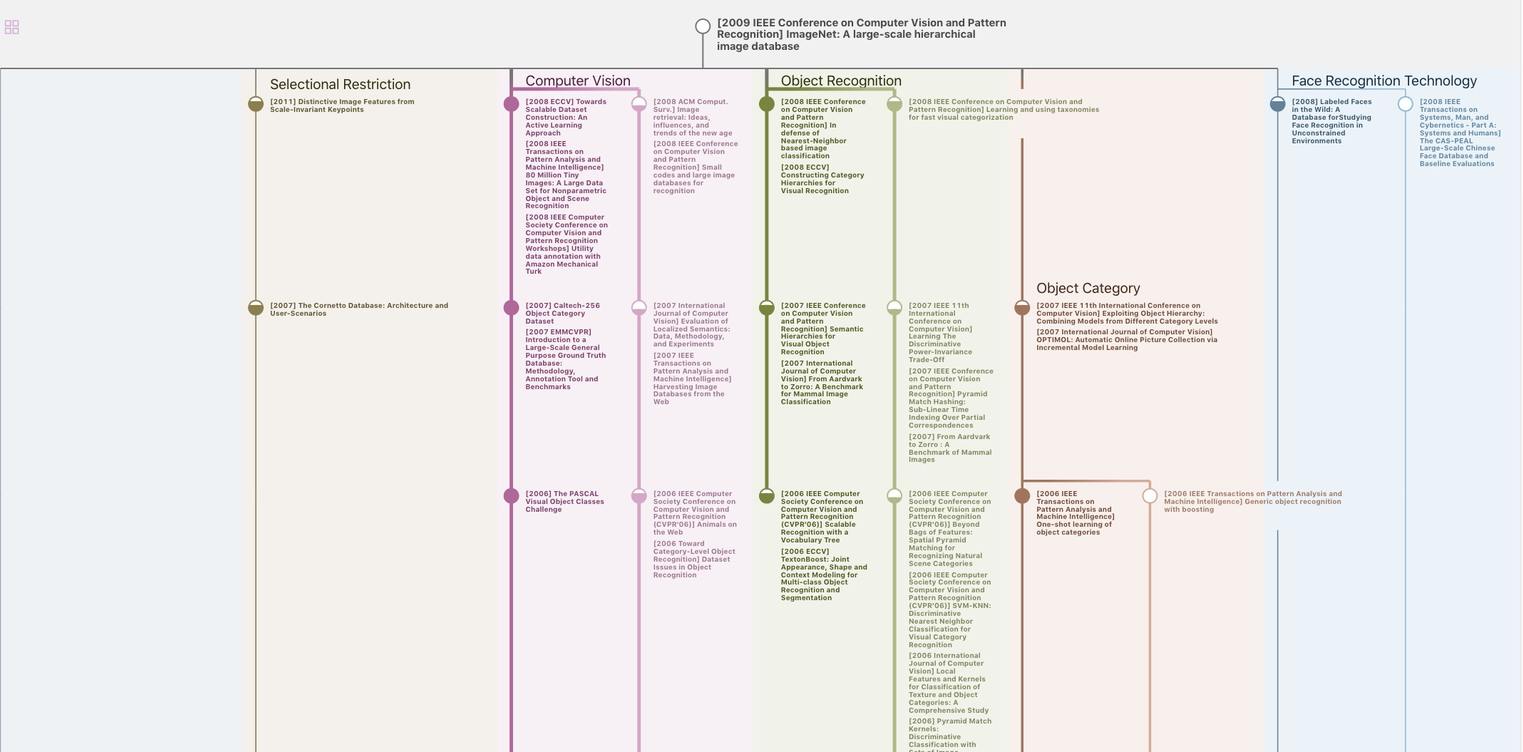
生成溯源树,研究论文发展脉络
Chat Paper
正在生成论文摘要