Model Reference Control by Recurrent Neural Network Built with Paraconsistent Neurons for Trajectory Tracking of a Rotary Inverted Pendulum
SSRN Electronic Journal(2022)
摘要
This investigation presents a recurrent paraconsistent neural network (RPNN), as the main element of the model reference control (MRC) strategy for the rotary inverted pendulum (RIP). The RIP character-istics, such as nonlinearity, two-degree-of-freedom (2DoF) motion, and under-actuated system, make it an ideal device to apply and test the RPNN. The designed paraconsistent neural model reference controller (PNMRC) uses three RPNNs: two of them to model the arm and pendulum angles and the third one to control the system while tracking a reference trajectory. The hidden neurons of the RPNN use the paraconsistent annotated logic by 2-value annotations (PAL2v) rules as an activation function. PAL2v, as a member of the paraconsistent logics family, deals with uncertain and contradictory data, representing a potentially robust alternative to applications of artificial neural networks in control. The PAL2v neuron is detailed and compared with other activation functions in recurrent neural networks (RNN). With real-time experiments, the PNMRC strategy is compared with classical control methodology, presenting excellent performance. ?? 2022 Elsevier B.V. All rights reserved.
更多查看译文
关键词
Paraconsistent logic,Neural models,Recurrent networks,Paraconsistent neural networks,Nonlinear control systems
AI 理解论文
溯源树
样例
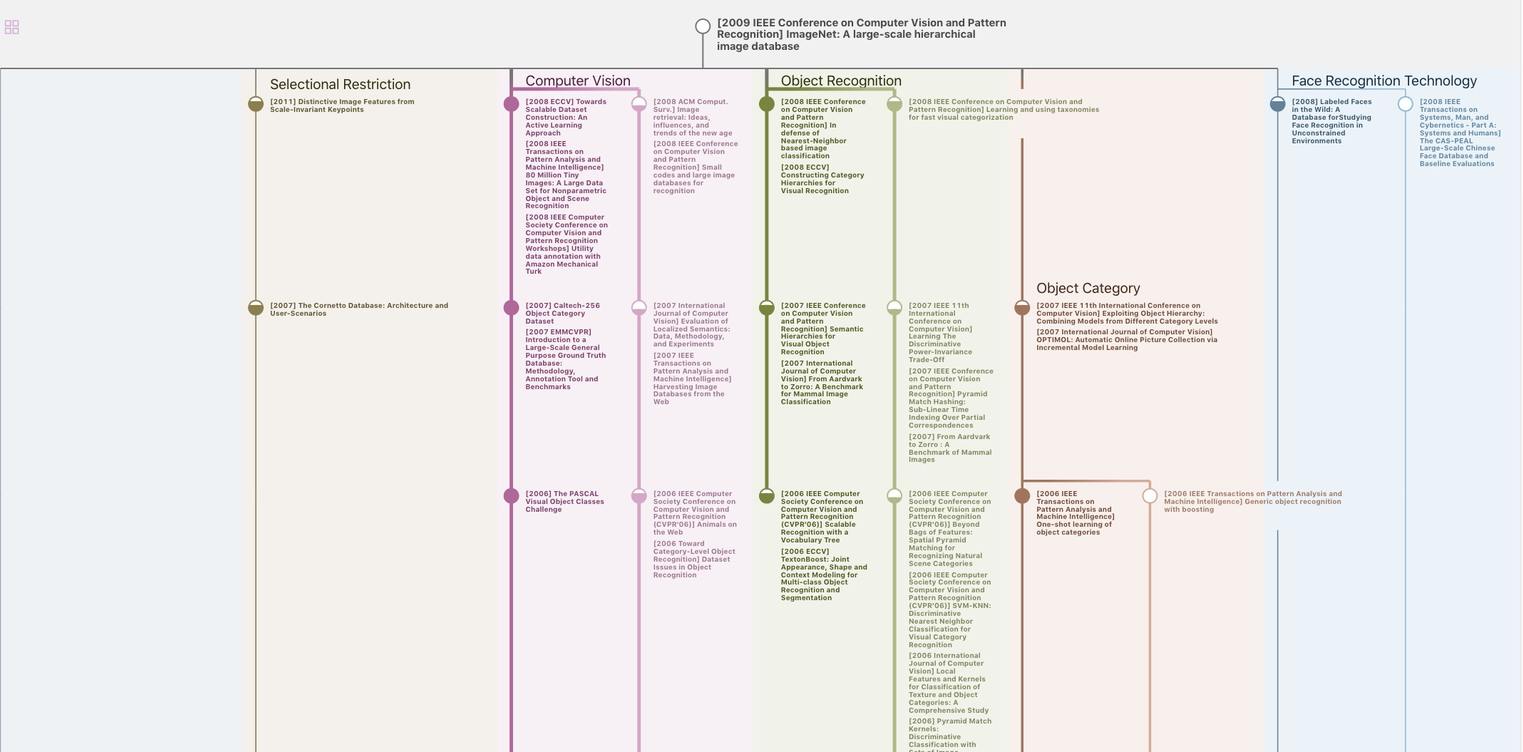
生成溯源树,研究论文发展脉络
Chat Paper
正在生成论文摘要