Real-Time And Robust 3D Object Detection with Roadside LiDARs
arxiv(2022)
摘要
This work aims to address the challenges in autonomous driving by focusing on the 3D perception of the environment using roadside LiDARs. We design a 3D object detection model that can detect traffic participants in roadside LiDARs in real-time. Our model uses an existing 3D detector as a baseline and improves its accuracy. To prove the effectiveness of our proposed modules, we train and evaluate the model on three different vehicle and infrastructure datasets. To show the domain adaptation ability of our detector, we train it on an infrastructure dataset from China and perform transfer learning on a different dataset recorded in Germany. We do several sets of experiments and ablation studies for each module in the detector that show that our model outperforms the baseline by a significant margin, while the inference speed is at 45 Hz (22 ms). We make a significant contribution with our LiDAR-based 3D detector that can be used for smart city applications to provide connected and automated vehicles with a far-reaching view. Vehicles that are connected to the roadside sensors can get information about other vehicles around the corner to improve their path and maneuver planning and to increase road traffic safety.
更多查看译文
关键词
Autonomous and connected vehicles, Traffic perception, 3D object detection, Roadside LiDAR, Point clouds, Deep learning, Transfer learning, Infrastructure, Testfield A9
AI 理解论文
溯源树
样例
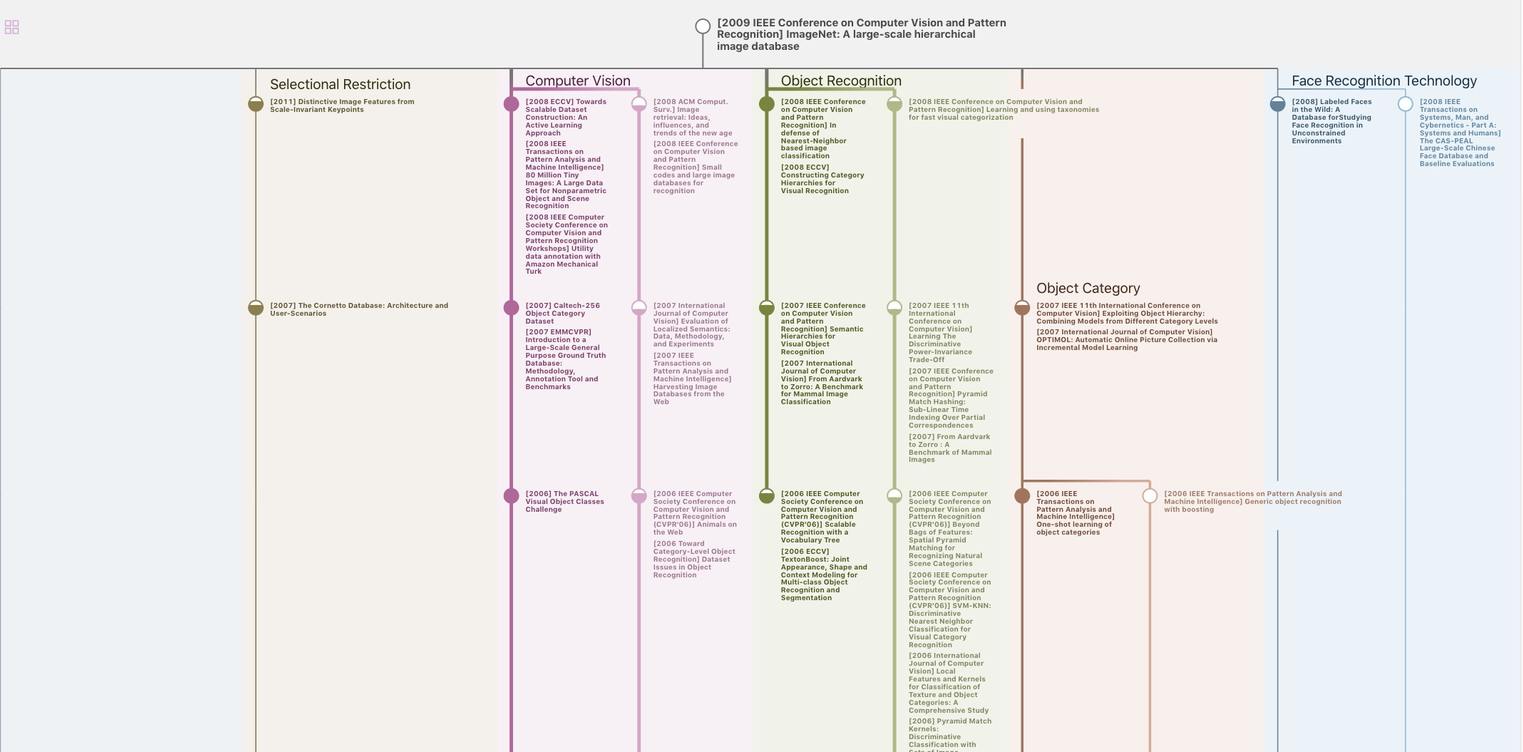
生成溯源树,研究论文发展脉络
Chat Paper
正在生成论文摘要