Label-Efficient Self-Supervised Speaker Verification With Information Maximization and Contrastive Learning
Conference of the International Speech Communication Association (INTERSPEECH)(2022)
摘要
State-of-the-art speaker verification systems are inherently dependent on some kind of human supervision as they are trained on massive amounts of labeled data. However, manually annotating utterances is slow, expensive and not scalable to the amount of data available today. In this study, we explore self-supervised learning for speaker verification by learning representations directly from raw audio. The objective is to produce robust speaker embeddings that have small intra-speaker and large inter-speaker variance. Our approach is based on recent information maximization learning frameworks and an intensive data augmentation pre-processing step. We evaluate the ability of these methods to work without contrastive samples before showing that they achieve better performance when combined with a contrastive loss. Furthermore, we conduct experiments to show that our method reaches competitive results compared to existing techniques and can get better performances compared to a supervised baseline when fine-tuned with a small portion of labeled data.
更多查看译文
关键词
Speaker Recognition, Self-Supervised Learning, Speaker Embeddings, Label-efficient
AI 理解论文
溯源树
样例
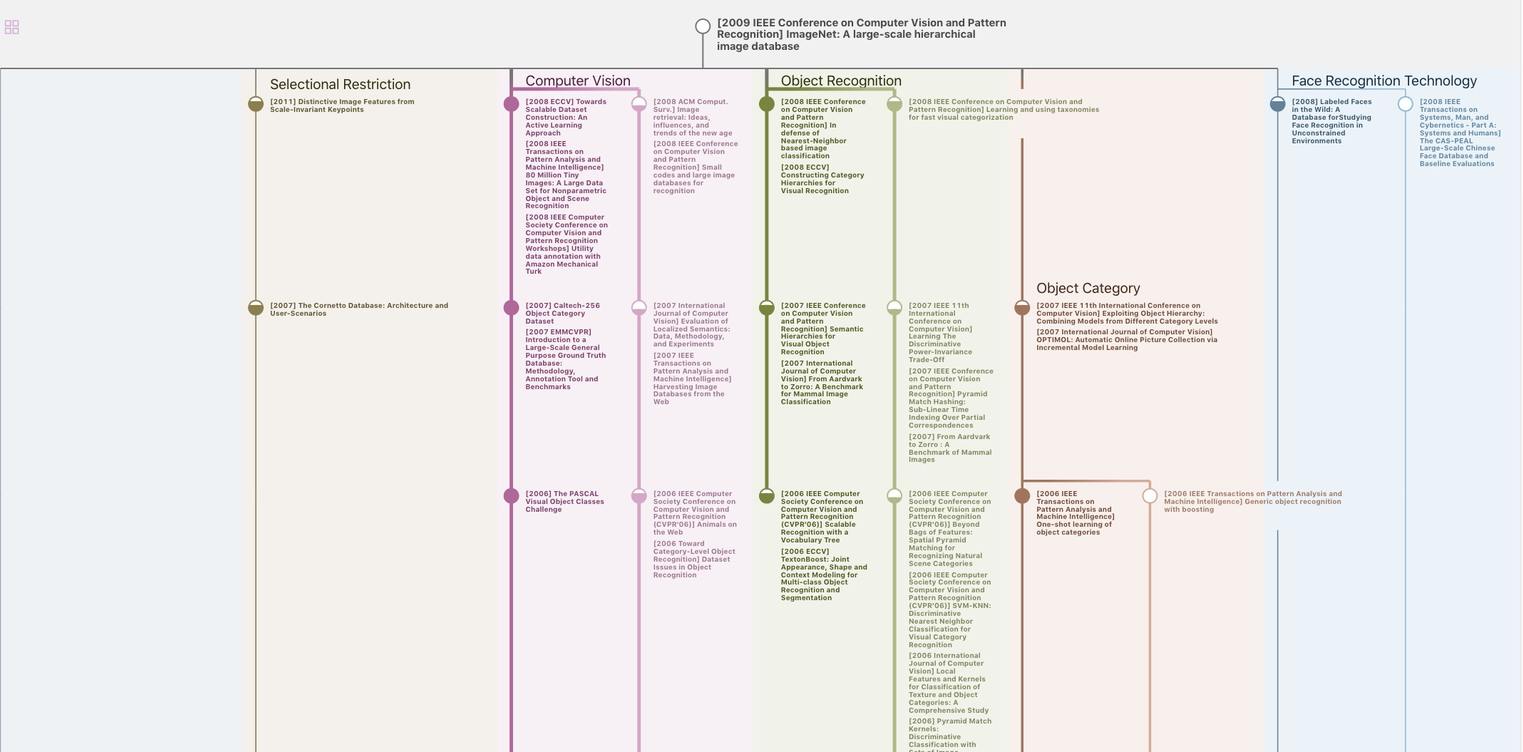
生成溯源树,研究论文发展脉络
Chat Paper
正在生成论文摘要