EfficientLEAF: A Faster LEarnable Audio Frontend of Questionable Use
2022 30th European Signal Processing Conference (EUSIPCO)(2022)
摘要
In audio classification, differentiable auditory filter-banks with few parameters cover the middle ground between hard-coded spectrograms and raw audio. LEAF [1], a Gabor-based filterbank combined with Per-Channel Energy Normalization (PCEN), has shown promising results, but is computationally expensive. With inhomogeneous convolution kernel sizes and strides, and by replacing PCEN with better parallelizable operations, we can reach similar results more efficiently. In experiments on six audio classification tasks, our frontend matches the accuracy of LEAF at 3% of the cost, but both fail to consistently outperform a fixed mel filterbank. The quest for learnable audio frontends is not solved.
更多查看译文
关键词
audio classification,CNNs,time-frequency rep-resentation,adaptive filterbanks
AI 理解论文
溯源树
样例
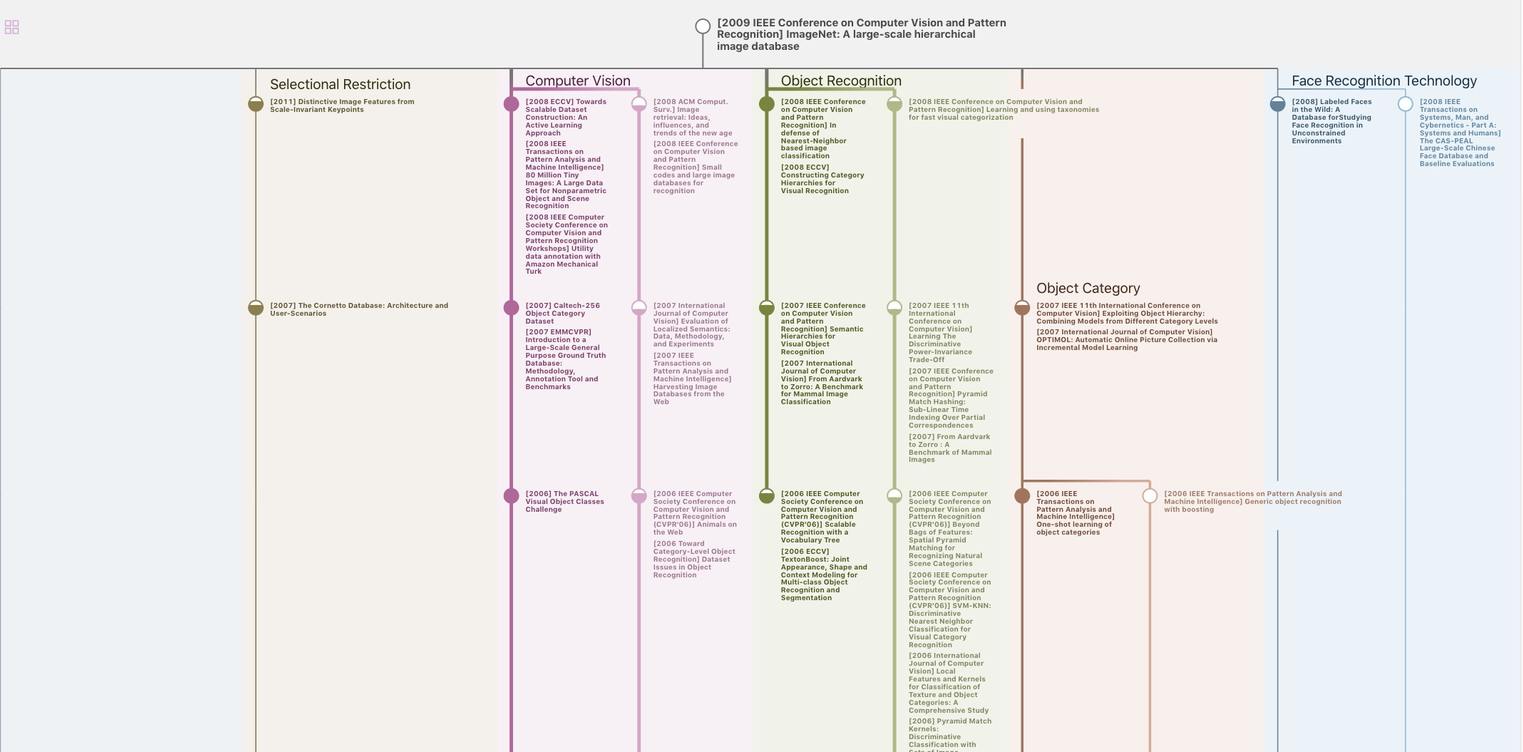
生成溯源树,研究论文发展脉络
Chat Paper
正在生成论文摘要