A spatial model to jointly analyze self-reported survey data of COVID-19 symptoms and official COVID-19 incidence data.
Biometrical journal. Biometrische Zeitschrift(2023)
摘要
This work presents a joint spatial modeling framework to improve estimation of the spatial distribution of the latent COVID-19 incidence in Belgium, based on test-confirmed COVID-19 cases and crowd-sourced symptoms data as reported in a large-scale online survey. Correction is envisioned for stochastic dependence between the survey's response rate and spatial COVID-19 incidence, commonly known as preferential sampling, but not found significant. Results show that an online survey can provide valuable auxiliary data to optimize spatial COVID-19 incidence estimation based on confirmed cases in situations with limited testing capacity. Furthermore, it is shown that an online survey on COVID-19 symptoms with a sufficiently large sample size per spatial entity is capable of pinpointing the same locations that appear as test-confirmed clusters, approximately 1 week earlier. We conclude that a large-scale online study provides an inexpensive and flexible method to collect timely information of an epidemic during its early phase, which can be used by policy makers in an early phase of an epidemic and in conjunction with other monitoring systems.
更多查看译文
关键词
COVID-19,bivariate conditional autoregressive random effect,disease mapping,preferential sampling,survey data
AI 理解论文
溯源树
样例
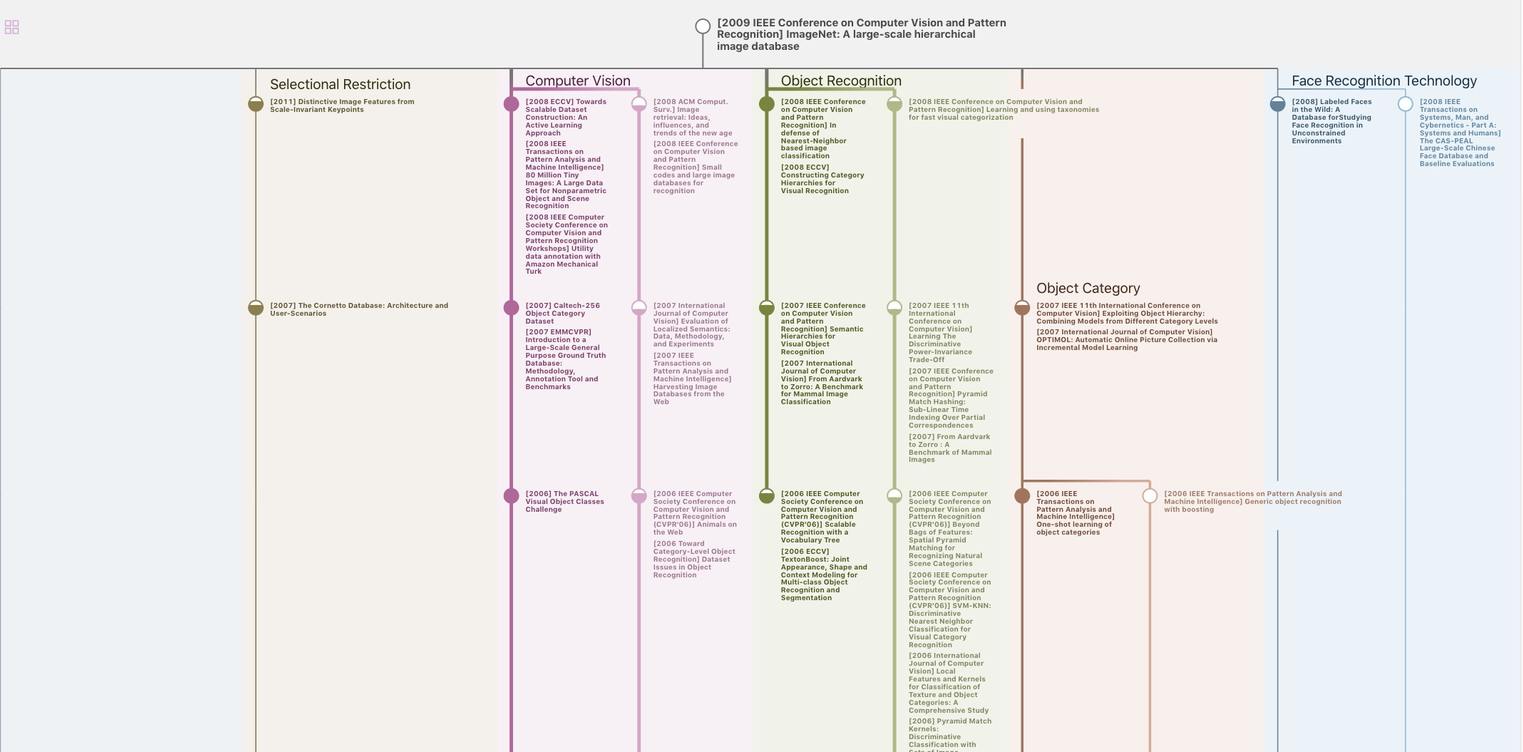
生成溯源树,研究论文发展脉络
Chat Paper
正在生成论文摘要