Barrier Lyapunov Function-Based Safe Reinforcement Learning for Autonomous Vehicles With Optimized Backstepping
IEEE TRANSACTIONS ON NEURAL NETWORKS AND LEARNING SYSTEMS(2024)
摘要
Guaranteed safety and performance under various circumstances remain technically critical and practically challenging for the wide deployment of autonomous vehicles. Safety-critical systems in general, require safe performance even during the reinforcement learning (RL) period. To address this issue, a Barrier Lyapunov Function-based safe RL (BLF-SRL) algorithm is proposed here for the formulated nonlinear system in strict-feedback form. This approach appropriately arranges and incorporates the BLF items into the optimized backstepping control method to constrain the state-variables in the designed safety region during learning. Wherein, thus, the optimal virtual/actual control in every backstepping subsystem is decomposed with BLF items and also with an adaptive uncertain item to be learned, which achieves safe exploration during the learning process. Then, the principle of Bellman optimality of continuous-time Hamilton-Jacobi-Bellman equation in every backstepping subsystem is satisfied with independently approximated actor and critic under the framework of actor-critic through the designed iterative updating. Eventually, the overall system control is optimized with the proposed BLF-SRL method. It is furthermore noteworthy that the variance of the attained control performance under uncertainty is also reduced with the proposed method. The effectiveness of the proposed method is verified with two motion control problems for autonomous vehicles through appropriate comparison simulations.
更多查看译文
关键词
Safety,Backstepping,Uncertainty,Nonlinear systems,Autonomous vehicles,Lyapunov methods,Control systems,Adaptive dynamic programming (ADP),autonomous vehicles,Barrier Lyapunov Function (BLF),safe reinforcement learning (SRL)
AI 理解论文
溯源树
样例
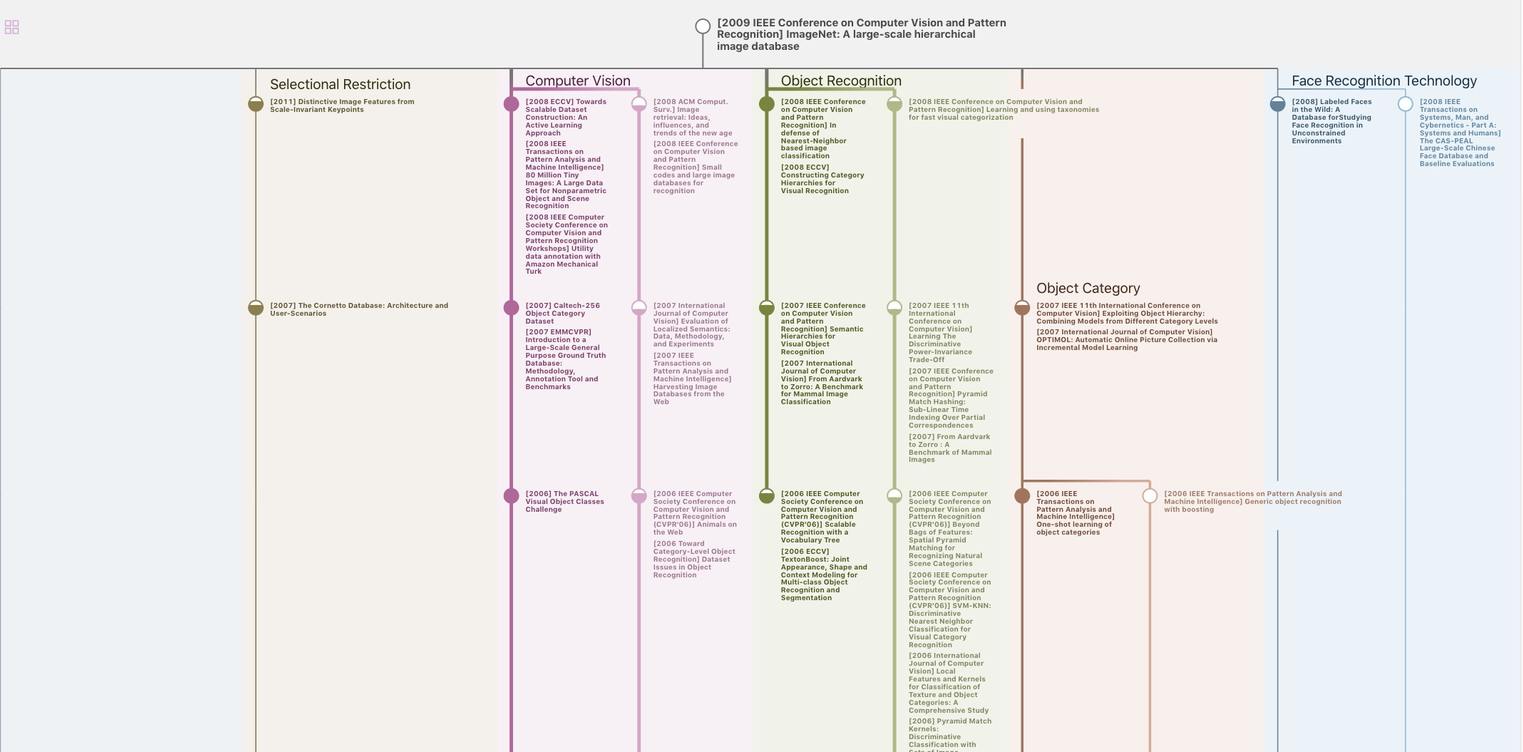
生成溯源树,研究论文发展脉络
Chat Paper
正在生成论文摘要