Deep Metric Learning on the SPD Manifold for Image Set Classification
IEEE TRANSACTIONS ON CIRCUITS AND SYSTEMS FOR VIDEO TECHNOLOGY(2024)
摘要
Thanks to the efficacy of Symmetric Positive Definite (SPD) manifold in characterizing video sequences (image sets), image set-based visual classification has made remarkable progress. However, the issue of large intra-class diversity and inter-class similarity is still an open challenge for the research community. Although several recent studies have alleviated the above issue by constructing Riemannian neural networks for SPD matrix nonlinear processing, the degradation of structural information during multi-stage feature transformation impedes them from going deeper. Besides, a single cross-entropy loss is insufficient for discriminative learning as it neglects the peculiarities of data distribution. To this end, this paper develops a novel framework for image set classification. Specifically, we first choose a mainstream neural network built on the SPD manifold (SPDNet) [25] as the backbone with a stacked SPD manifold autoencoder (SSMAE) built on the tail to enrich the structured representations. Due to the associated reconstruction error terms, the embedding mechanism of both SSMAE and each SPD manifold autoencoder (SMAE) forms an approximate identity mapping, simplifying the training of the suggested deeper network. Then, the ReCov layer is introduced with a nonlinear function for the constructed architecture to narrow the discrepancy of the intra-class distributions from the perspective of regularizing the local statistical information of the SPD data. Afterward, two progressive metric learning stages are coupled with the proposed SSMAE to explicitly capture, encode, and analyze the geometric distributions of the generated deep representations during training. In consequence, not only a more powerful Riemannian network embedding but also effective classifiers can be obtained. Finally, a simple maximum voting strategy is applied to the outputs of the learned multiple classifiers for classification. The proposed model is evaluated on three typical visual classification tasks using widely adopted benchmarking datasets. Extensive experiments show its superiority over the state of the arts.
更多查看译文
关键词
SPD manifold,image set classification,Riemannian neural network,stacked SPD manifold autoencoder (SSMAE),metric learning
AI 理解论文
溯源树
样例
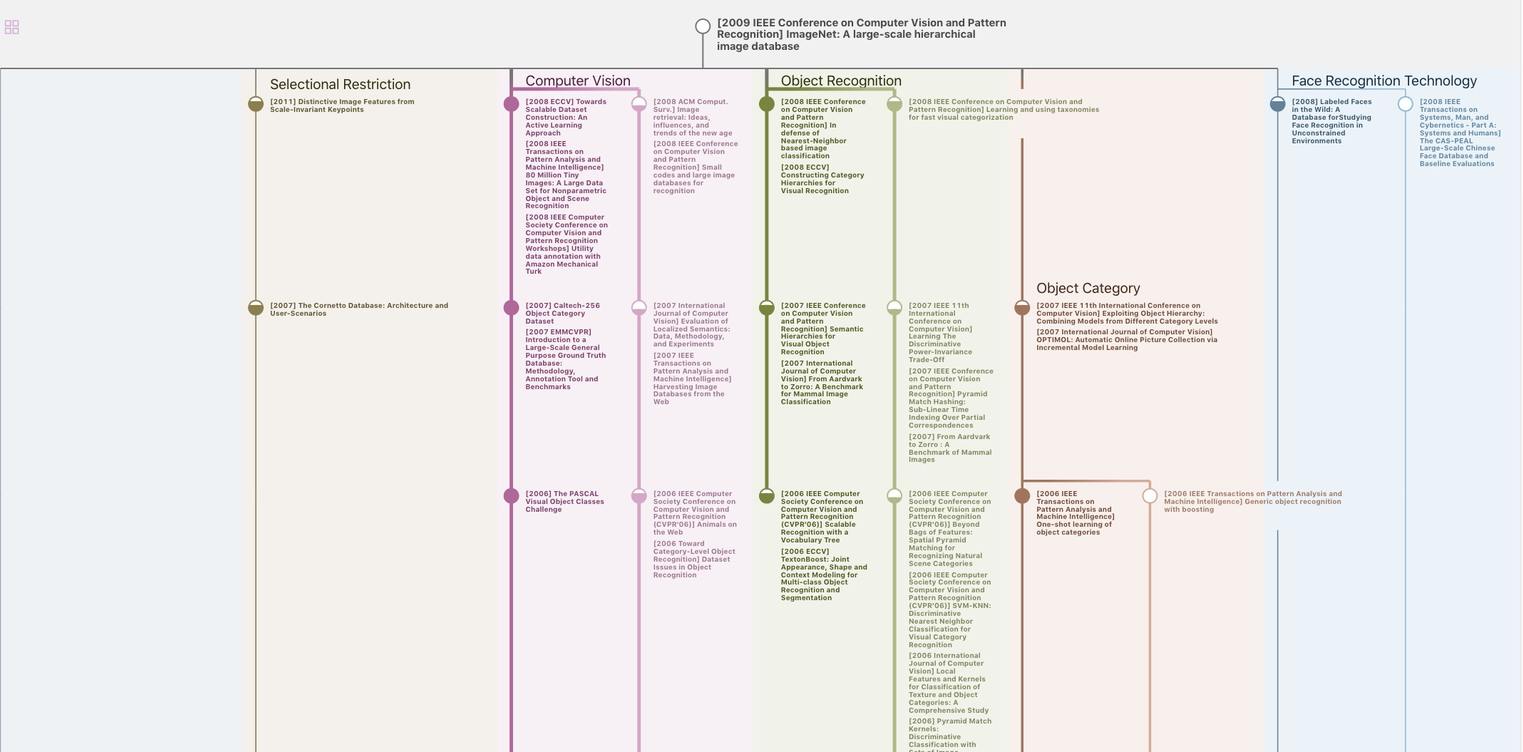
生成溯源树,研究论文发展脉络
Chat Paper
正在生成论文摘要