Semi-Supervised Leukocyte Segmentation Based on Adversarial Learning With Reconstruction Enhancement
IEEE TRANSACTIONS ON INSTRUMENTATION AND MEASUREMENT(2022)
摘要
The number, relative ratio, and appearance of peripheral blood leukocytes can assist doctors to diagnose diseases such as lymphoma and leukemia. Therefore, segmentation of peripheral blood leukocytes in blood smear images plays a crucial role in the diagnosis of certain diseases. We propose a semisupervised leukocyte segmentation method under the framework of adversarial learning, in which a discriminator is trained to differentiate the segmentation maps coming either from the ground truth or from the segmentation network. Specifically, we first construct a lightweight leukocyte segmentation network by modifying DeepLabV3+ with simplified MobileNetV2. In addition, a reconstruction decoder is developed as a constraint on feature extraction to recover the original leukocyte image from its segmentation result. Then, we design the discriminator in a fully convolutional manner, which as well enables semi-supervised learning by inferring reliable supervisory information for unlabeled images. Quantitative and qualitative experimental results on four image datasets demonstrate the superiority of the proposed method over several state-of-the-art methods, achieving the highest average F1 score of 0.9717 and mIoU of 0.9493 with only 20% labeled training samples.
更多查看译文
关键词
Generative adversarial network (GAN),image segmentation,leukocyte,reconstruction enhancement,semi-supervised learning
AI 理解论文
溯源树
样例
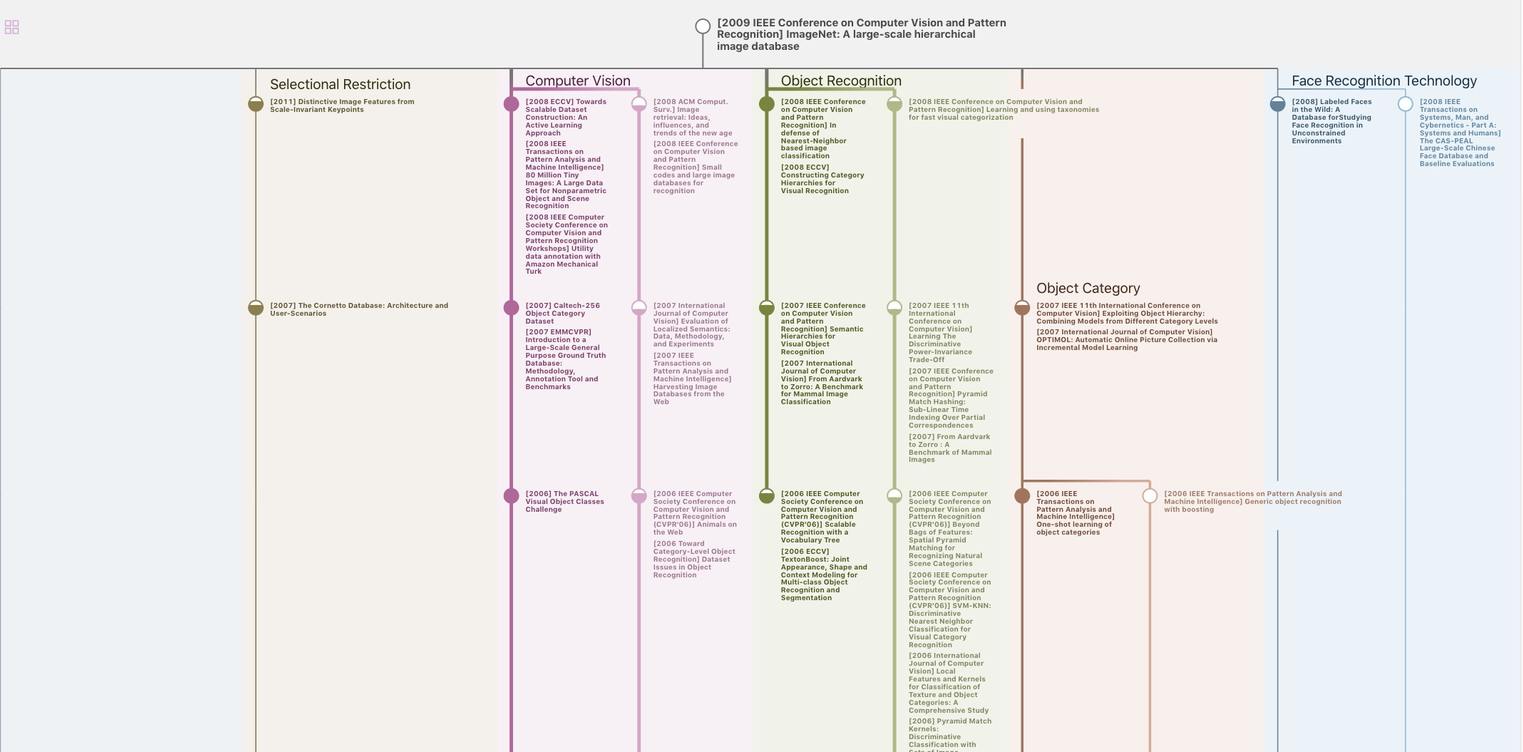
生成溯源树,研究论文发展脉络
Chat Paper
正在生成论文摘要