Using wavelets to capture deviations from smoothness in galaxy-scale strong lenses
arxiv(2022)
摘要
Modeling the mass distribution of galaxy-scale strong gravitational lenses is a task of increasing difficulty. The high-resolution and depth of imaging data now available render simple analytical forms ineffective at capturing lens structures spanning a large range in spatial scale, mass scale, and morphology. In this work, we address the problem with a novel multiscale method based on wavelets. We tested our method on simulated Hubble Space Telescope (HST) imaging data of strong lenses containing the following different types of mass substructures making them deviate from smooth models: (1) a localized small dark matter subhalo, (2) a Gaussian random field (GRF) that mimics a nonlocalized population of subhalos along the line of sight, and (3) galaxy-scale multipoles that break elliptical symmetry. We show that wavelets are able to recover all of these structures accurately. This is made technically possible by using gradient-informed optimization based on automatic differentiation over thousands of parameters, which also allow us to sample the posterior distributions of all model parameters simultaneously. By construction, our method merges the two main modeling paradigms - analytical and pixelated - with machine-learning optimization techniques into a single modular framework. It is also well-suited for the fast modeling of large samples of lenses. All methods presented here are publicly available in our new Herculens package.
更多查看译文
关键词
galaxies,structure,dark matter,methods,data analysis,gravitation,gravitational lensing,strong
AI 理解论文
溯源树
样例
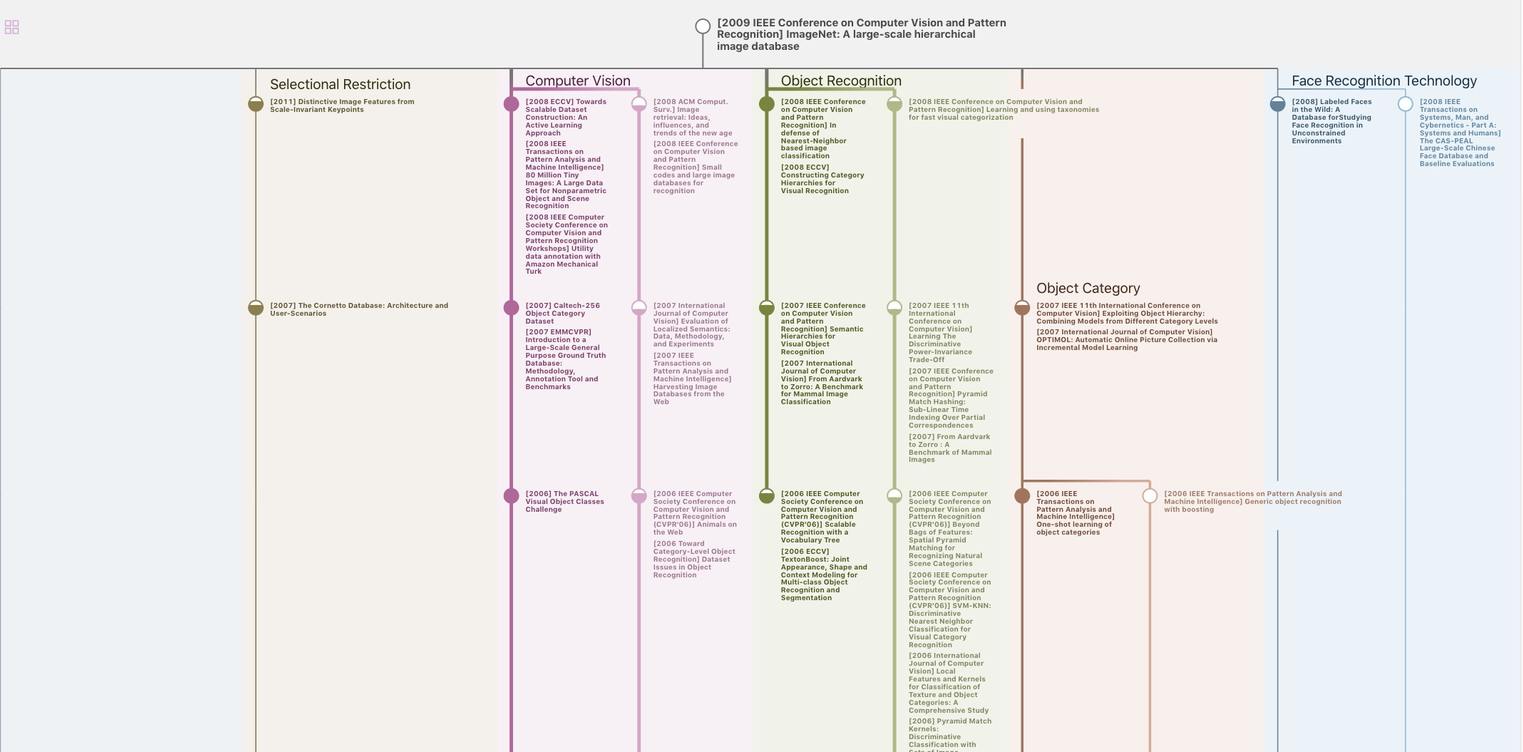
生成溯源树,研究论文发展脉络
Chat Paper
正在生成论文摘要