Brick Tic-Tac-Toe: Exploring the Generalizability of AlphaZero to Novel Test Environments
arxiv(2022)
摘要
Traditional reinforcement learning (RL) environments typically are the same for both the training and testing phases. Hence, current RL methods are largely not generalizable to a test environment which is conceptually similar but different from what the method has been trained on, which we term the novel test environment. As an effort to push RL research towards algorithms which can generalize to novel test environments, we introduce the Brick Tic-Tac-Toe (BTTT) test bed, where the brick position in the test environment is different from that in the training environment. Using a round-robin tournament on the BTTT environment, we show that traditional RL state-search approaches such as Monte Carlo Tree Search (MCTS) and Minimax are more generalizable to novel test environments than AlphaZero is. This is surprising because AlphaZero has been shown to achieve superhuman performance in environments such as Go, Chess and Shogi, which may lead one to think that it performs well in novel test environments. Our results show that BTTT, though simple, is rich enough to explore the generalizability of AlphaZero. We find that merely increasing MCTS lookahead iterations was insufficient for AlphaZero to generalize to some novel test environments. Rather, increasing the variety of training environments helps to progressively improve generalizability across all possible starting brick configurations.
更多查看译文
关键词
alphazero,test,tic-tac-toe
AI 理解论文
溯源树
样例
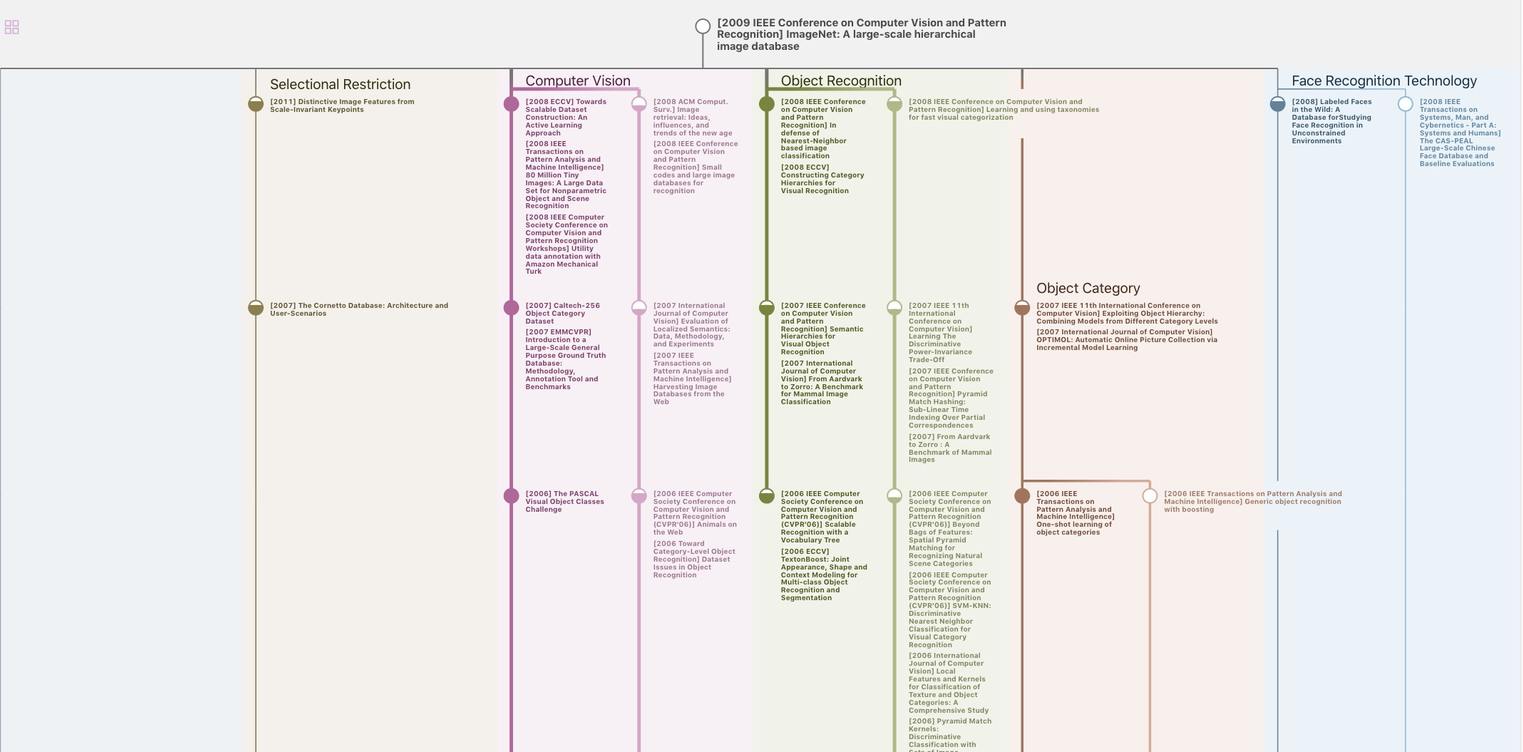
生成溯源树,研究论文发展脉络
Chat Paper
正在生成论文摘要