Reachability Analysis of a General Class of Neural Ordinary Differential Equations.
International Conference on Formal Modeling and Analysis of Timed Systems (FORMATS)(2022)
摘要
Continuous deep learning models, referred to as Neural Ordinary Differential Equations (Neural ODEs), have received considerable attention over the last several years. Despite their burgeoning impact, there is a lack of formal analysis techniques for these systems. In this paper, we consider a general class of neural ODEs with varying architectures and layers, and introduce a novel reachability framework that allows for the formal analysis of their behavior. The methods developed for the reachability analysis of neural ODEs are implemented in a new tool called NNVODE. Specifically, our work extends an existing neural network verification tool to support neural ODEs. We demonstrate the capabilities and efficacy of our methods through the analysis of a set of benchmarks that include neural ODEs used for classification, and in control and dynamical systems, including an evaluation of the efficacy and capabilities of our approach with respect to existing software tools within the continuous-time systems reachability literature, when it is possible to do so.
更多查看译文
关键词
neural ordinary differential
AI 理解论文
溯源树
样例
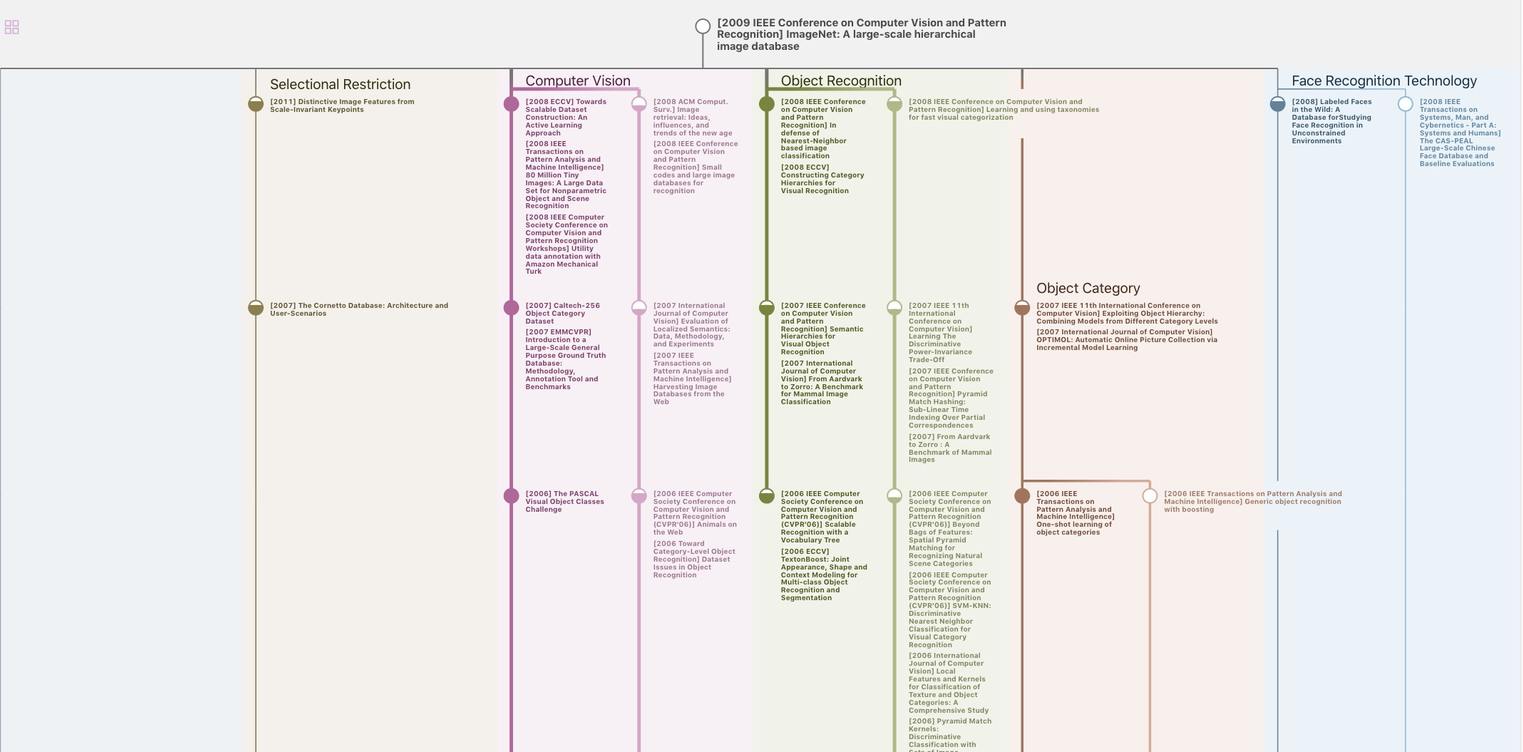
生成溯源树,研究论文发展脉络
Chat Paper
正在生成论文摘要