Dual-Level Knowledge Distillation via Knowledge Alignment and Correlation
IEEE TRANSACTIONS ON NEURAL NETWORKS AND LEARNING SYSTEMS(2024)
摘要
Knowledge distillation (KD) has become a widely used technique for model compression and knowledge transfer. We find that the standard KD method performs the knowledge alignment on an individual sample indirectly via class prototypes and neglects the structural knowledge between different samples, namely, knowledge correlation. Although recent contrastive learning-based distillation methods can be decomposed into knowledge alignment and correlation, their correlation objectives undesirably push apart representations of samples from the same class, leading to inferior distillation results. To improve the distillation performance, in this work, we propose a novel knowledge correlation objective and introduce the dual-level knowledge distillation (DLKD), which explicitly combines knowledge alignment and correlation together instead of using one single contrastive objective. We show that both knowledge alignment and correlation are necessary to improve the distillation performance. In particular, knowledge correlation can serve as an effective regularization to learn generalized representations. The proposed DLKD is task-agnostic and model-agnostic, and enables effective knowledge transfer from supervised or self-supervised pretrained teachers to students. Experiments show that DLKD outperforms other state-of-the-art methods on a large number of experimental settings including: 1) pretraining strategies; 2) network architectures; 3) datasets; and 4) tasks.
更多查看译文
关键词
Correlation,Knowledge engineering,Task analysis,Standards,Network architecture,Prototypes,Training,Convolutional neural networks,dual-level knowledge,knowledge distillation (KD),representation learning,teacher-student model
AI 理解论文
溯源树
样例
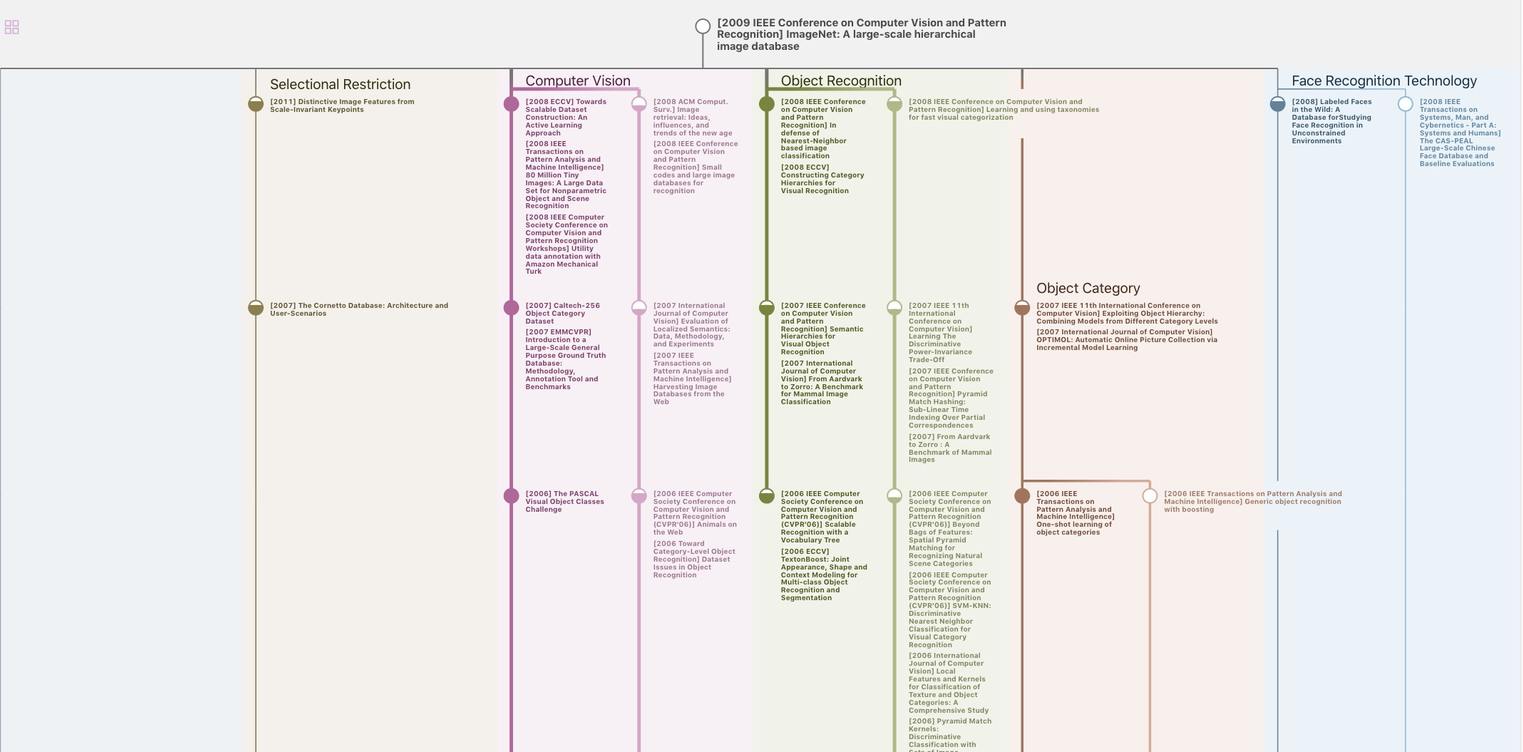
生成溯源树,研究论文发展脉络
Chat Paper
正在生成论文摘要