A novel information processing method based on an ensemble of Auto-Encoders for unsupervised fault detection
COMPUTERS IN INDUSTRY(2022)
摘要
The current research paper proposes a novel information processing Deep Learning framework for un-supervised fault detection applications, where during the training process only samples from the normal class are available. Recently, unsupervised fault detection methods based on the Auto-Encoders have been successful and are used extensively. They are supported by the assumption that abnormal unknown sam-ples that don't belong to the learned manifold of the training dataset of normal points produce higher reconstruction cost than the normal samples. The presented scheme is based on an ensemble of different types of Auto-Encoders; each of them is trained independently for the one-class fault detection task. The final agreement of the normality of the testing sample is made from a soft voting process, where the confidence of each Auto-Encoder about its decision is considered. The significance of each individual Auto -Encoder in the final decision is extracted from a statistical analysis of the independent training process of each one. Simulation results with three widely used fault detection datasets show the effectiveness of the proposed model.(c) 2022 Elsevier B.V. All rights reserved.
更多查看译文
关键词
Anomaly detection,Auto -Encoders,Deep learning,Ensemble learning,Fault detection,Unsupervised learning
AI 理解论文
溯源树
样例
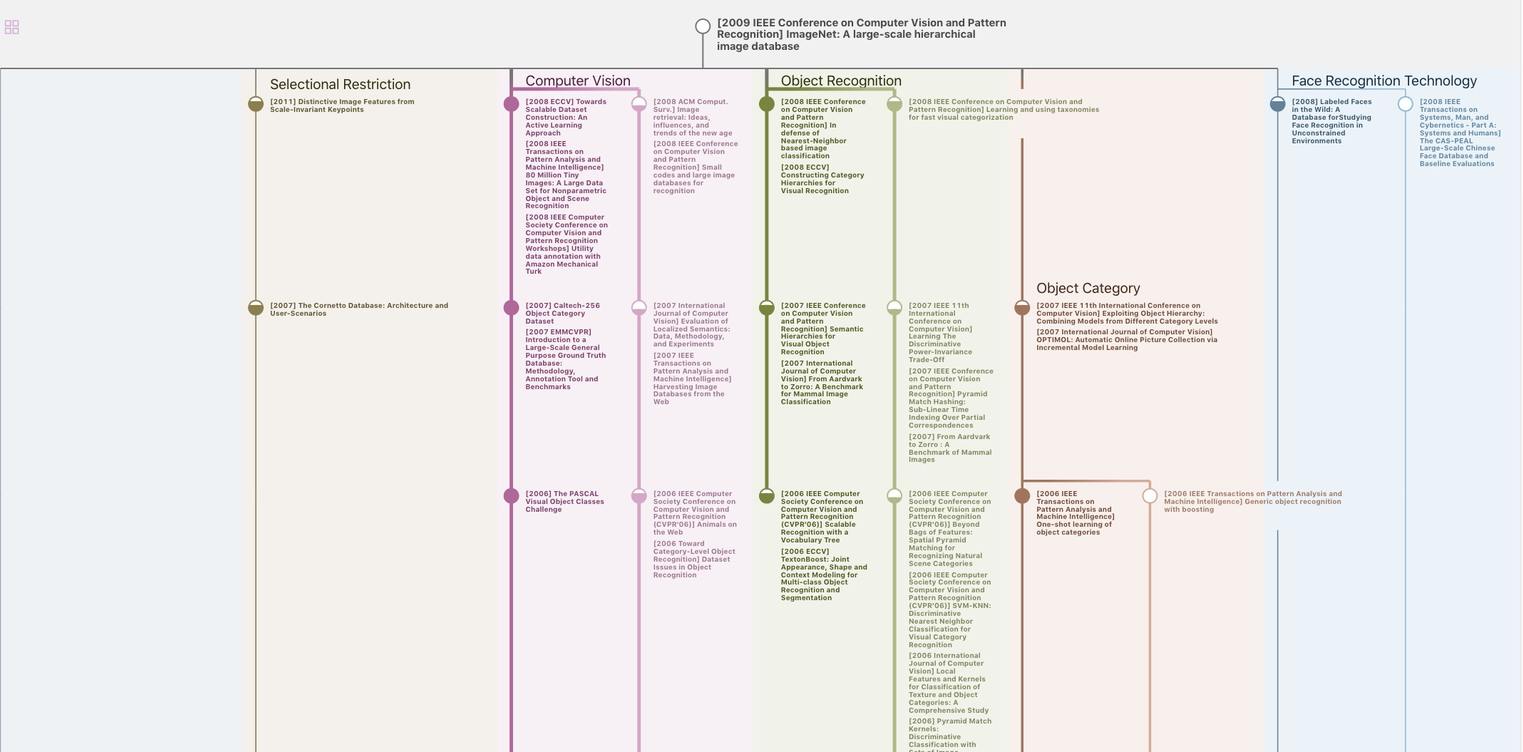
生成溯源树,研究论文发展脉络
Chat Paper
正在生成论文摘要