PRNU-based Image Forgery Localization with Deep Multi-scale Fusion
ACM Transactions on Multimedia Computing, Communications, and Applications(2023)
摘要
Photo-response non-uniformity (PRNU), as a class of device fingerprint, plays a key role in the forgery detection/localization for visual media. The state-of-the-art PRNU-based forensics methods generally rely on the multi-scale trace analysis and result fusion, with Markov random field model. However, such handcrafted strategies are difficult to provide satisfactory multi-scale decision, exhibiting a high false-positive rate. Motivated by this, we propose an end-to-end multi-scale decision fusion strategy, where a mapping from multi-scale forgery probabilities to binary decision is achieved by a supervised deep fully connected neural network. As the first time, the deep learning technology is employed in PRNU-based forensics for more flexible and reliable integration of multi-scale information. The benchmark experiments exhibit the state-ofthe-art accuracy performance of our method in both pixel-level and image-level, especially for false positives. Additional robustness experiments also demonstrate the benefits of the proposed method in resisting noise and compression attacks.
更多查看译文
关键词
Image forgery localization,multi-scale analysis,photo-response non-uniformity,deep learning
AI 理解论文
溯源树
样例
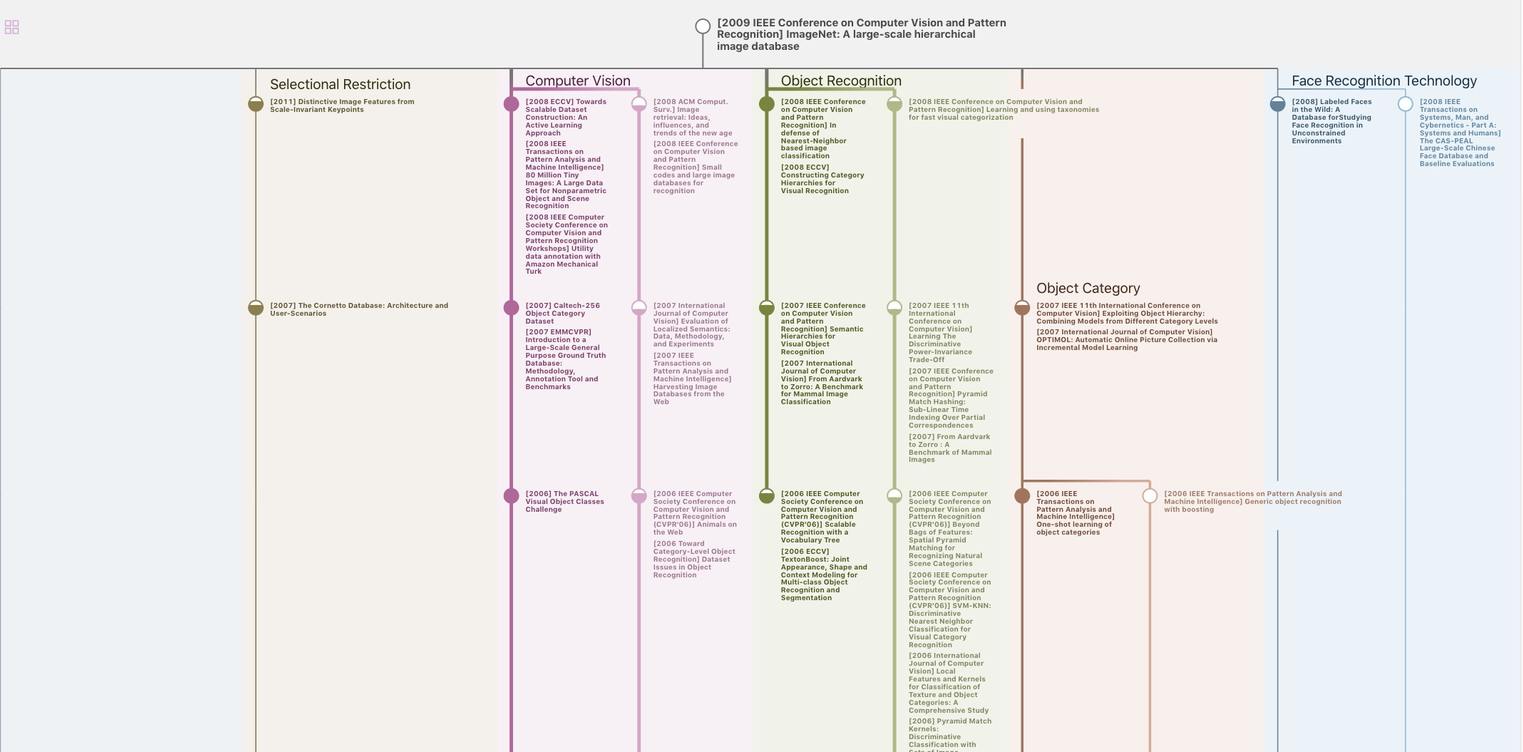
生成溯源树,研究论文发展脉络
Chat Paper
正在生成论文摘要