Engineering Extracellular Electron Transfer in Escherichia coli for Microbial Electrochemical Devices
ECS Meeting Abstracts(2022)
摘要
Microbes hold great potential for green electrochemical synthesis as well as bio-electricity generation from organic waste. The key to the successful development of microbial electrochemical devices lies with the ability of the microbes to transport electrons across their outer membranes. These devices thus often rely on the use of microbes known as exoelectrogens, which include bacteria that have evolved metabolic pathways that enable extracellular electron transfer (EET) to solid substrates for cellular respiration. While exoelectrogens demonstrate facilitated electron transfer with the electrodes of these devices, natural exoelectrogens are often restricted to utilizing a narrow range of substrates that limits their versatility for processing different organic wastes and electrosynthesizing a rich diversity of high-value chemicals. By contrast, non-exoelectrogenic microbes such as Escherichia coli that lack efficient EET pathways are host to rich metabolic reaction networks that can be more readily tailored for various applications using synthetic biology. In this work, we biologically engineer E. coli for enhanced EET. To this end, we expressed different combinations of proteins from the natural exoelectrogen S. oneidensis MR-1, as well as E. coli native proteins to optimize EET of the bioengineered strain. The bioengineered strains show significant improvements in electron transfer rates, as confirmed through colorimetric reduction assays of soluble electron acceptors and electrochemical characterizations following electrode reduction. The improved EET demonstrated in this work paves the way for increasing the efficiency of existing E. c oli - based electrochemical devices while opening the doors to new applications that benefit from the broad chemical repertoire of these microbes.
更多查看译文
AI 理解论文
溯源树
样例
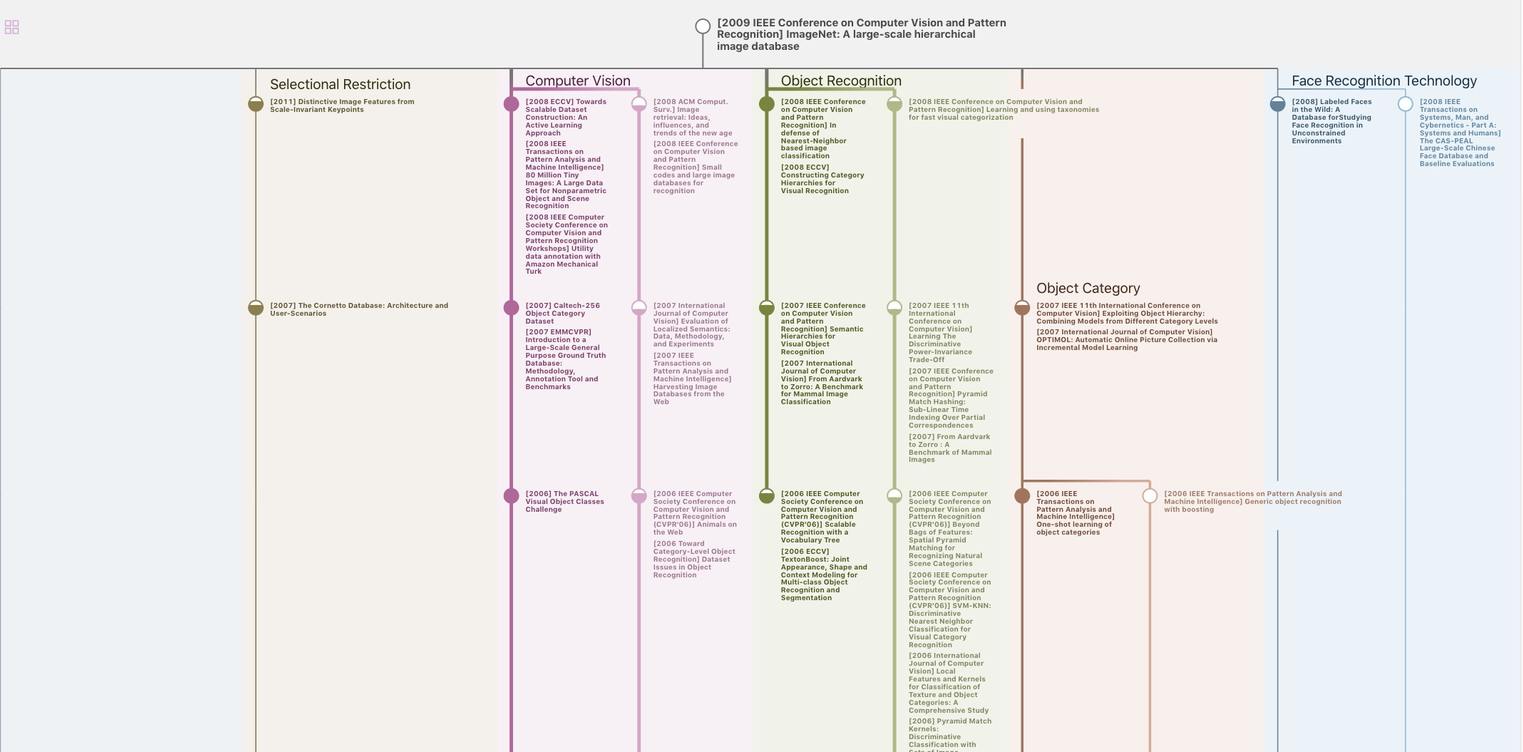
生成溯源树,研究论文发展脉络
Chat Paper
正在生成论文摘要