The estimation of hourly PM2.5 concentrations across China based on a Spatial and Temporal Weighted Continuous Deep Neural Network (STWC-DNN)
ISPRS Journal of Photogrammetry and Remote Sensing(2022)
摘要
The continuous distributions of PM2.5 concentrations and predictor variables in the surrounding regions influence the PM2.5 concentrations in the prediction positions notably, yet few machine learning models quantified the spatially continuous interactions between PM2.5 concentrations and predictor variations, which limits the prediction accuracy. To fill this gap, a Spatial and Temporal Weighted Continuous Deep Neural Network (STWC-DNN) was proposed. For STWC-DNN, three sub-networks, Single Pixel Network (SPN), Multiple Station Network (MSN), and Continuous Region Network (CRN) were designed to analyze the influence of predictor variables at the prediction position, the influence of PM2.5 concentrations from surrounding stations, and the influence of continuous raster predictor variables from surrounding pixels respectively. STWC-DNN was experimented using hourly Himawari AOD data and the outputs were compared with a series of advanced models. STWC-DNN achieved higher accuracy than existing models and the sample-based, time-based, and station-based 10-fold cross-validation (CV) R2 were 0.92, 0.90, and 0.79, respectively. The principle of establishing STWC-DNN sheds useful lights on the effective use of raster predictor variables and automatic spatiotemporal weight function to better estimate PM2.5 and other airborne pollutants based on multiple data sources. The codes of STWC-DNN are now available at https://github.com/wangzh2022/STWC-DNN.
更多查看译文
关键词
PM2.5 estimation,AOD,Himawari-8,Deep neural network,Automatic spatiotemporal weight function,Continuous spatial distribution
AI 理解论文
溯源树
样例
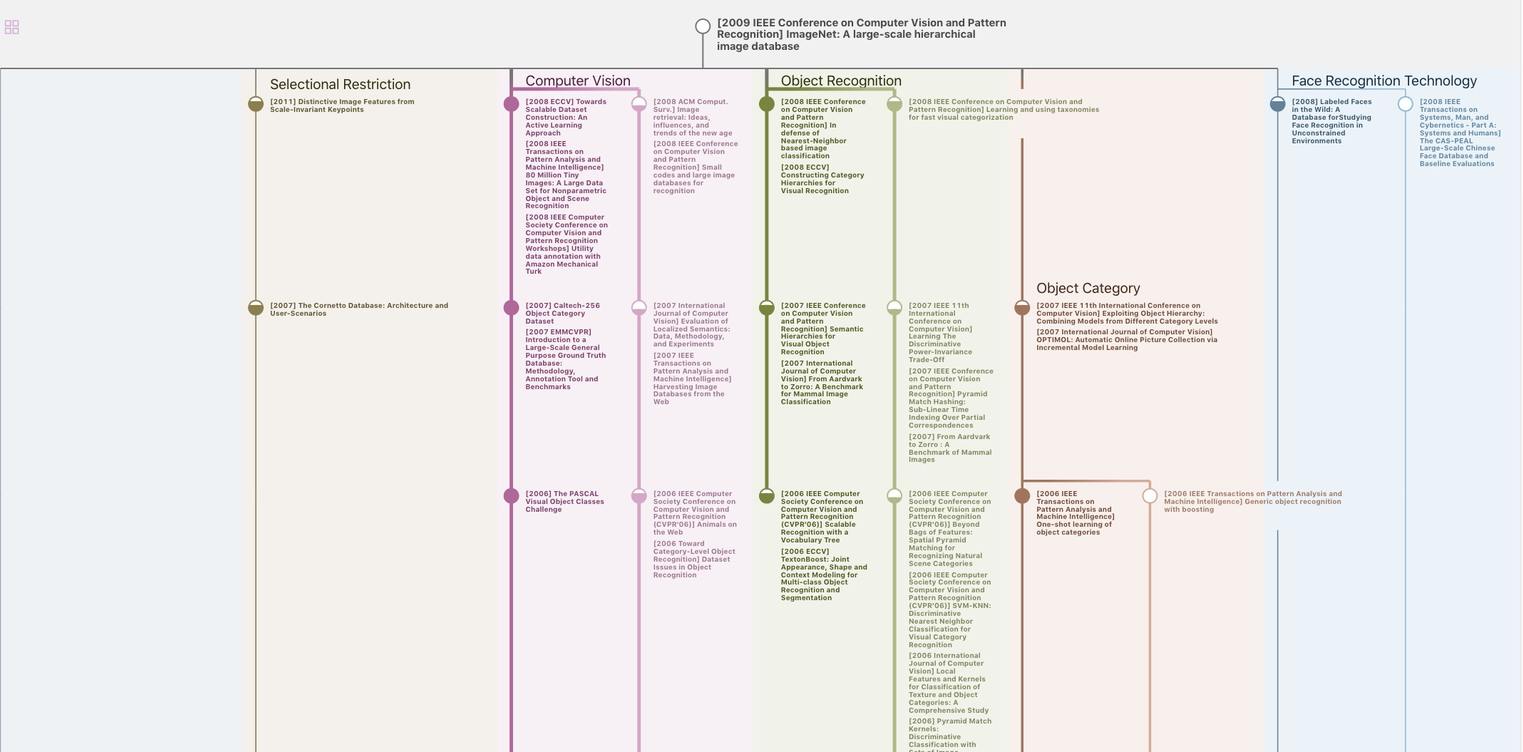
生成溯源树,研究论文发展脉络
Chat Paper
正在生成论文摘要