An explainable framework for load forecasting of a regional integrated energy system based on coupled features and multi-task learning
Protection and Control of Modern Power Systems(2022)
Abstract
To extract strong correlations between different energy loads and improve the interpretability and accuracy for load forecasting of a regional integrated energy system (RIES), an explainable framework for load forecasting of an RIES is proposed. This includes the load forecasting model of RIES and its interpretation. A coupled feature extracting strategy is adopted to construct coupled features between loads as the input variables of the model. It is designed based on multi-task learning (MTL) with a long short-term memory (LSTM) model as the sharing layer. Based on SHapley Additive exPlanations (SHAP), this explainable framework combines global and local interpretations to improve the interpretability of load forecasting of the RIES. In addition, an input variable selection strategy based on the global SHAP value is proposed to select input feature variables of the model. A case study is given to verify the effectiveness of the proposed model, constructed coupled features, and input variable selection strategy. The results show that the explainable framework intuitively improves the interpretability of the prediction model.
MoreTranslated text
Key words
Load forecasting, Regional integrated energy system, Coupled feature, SHapley additive exPlanations, Interpretability of deep learning
AI Read Science
Must-Reading Tree
Example
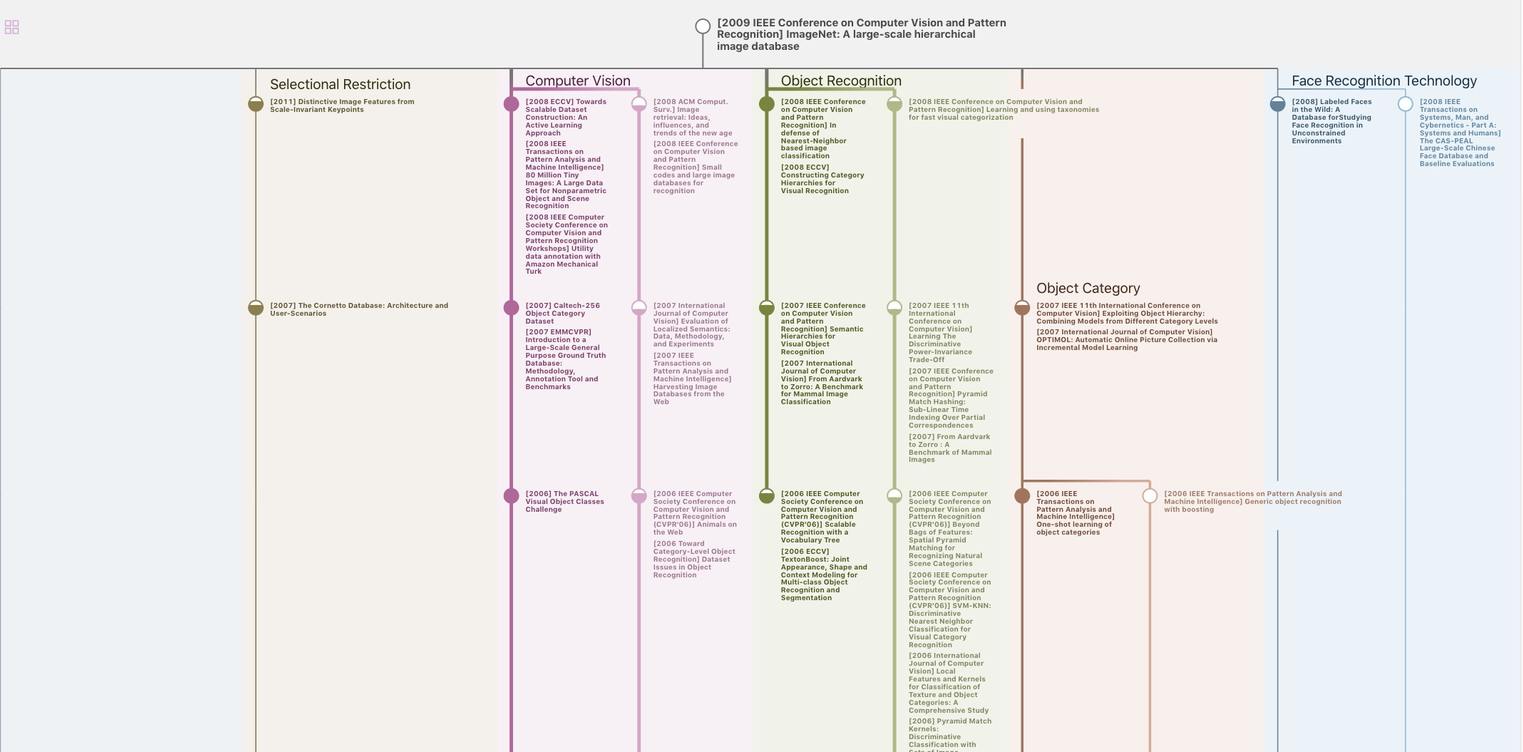
Generate MRT to find the research sequence of this paper
Chat Paper
Summary is being generated by the instructions you defined