Comparison of MLP and RBF Neural Networks for Bearing Remaining Useful Life Prediction Based on Acoustic Emission
PROCEEDINGS OF THE INSTITUTION OF MECHANICAL ENGINEERS PART J-JOURNAL OF ENGINEERING TRIBOLOGY(2023)
关键词
MLP neural networks,RBF neural networks,remaining useful life,angular contact bearing,acoustic emission
AI 理解论文
溯源树
样例
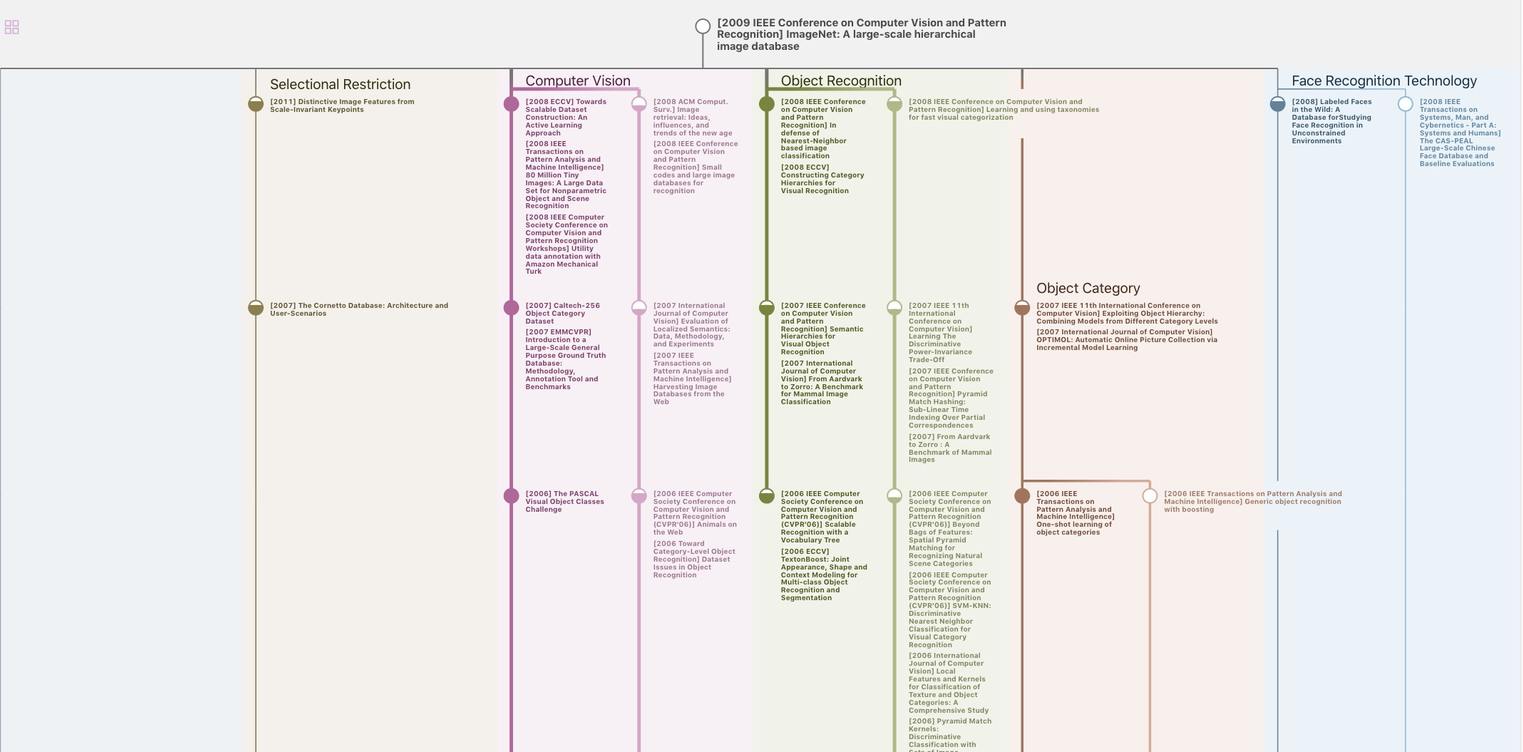
生成溯源树,研究论文发展脉络
Chat Paper
正在生成论文摘要